Pull-back Geometry of Persistent Homology Encodings
arXiv (Cornell University)(2023)
摘要
Persistent homology (PH) is a method that allows to create topology-inspired representations of data. In contrast to data-driven representation methods, it is an expert-based method with explicit computations designed by hand. Nonetheless, owing to the complexity of these computations, its properties and interpretation have remained elusive. We investigate the spectrum of the Jacobian of PH data encodings and the pull-back geometry that they induce on the data manifold. Then, by measuring different perturbations and features on the data manifold with respect to this geometry, we can identify which of them are recognized or ignored by the PH encodings. This also allows us to compare different encodings in terms of their induced geometry. Importantly, the approach does not require training and testing on a particular task and permits a direct exploration of PH. We experimentally demonstrate that the pull-back norm can be used as a predictor of performance on downstream tasks and to select suitable PH encodings accordingly.
更多查看译文
关键词
persistent homology encodings,pull-back
AI 理解论文
溯源树
样例
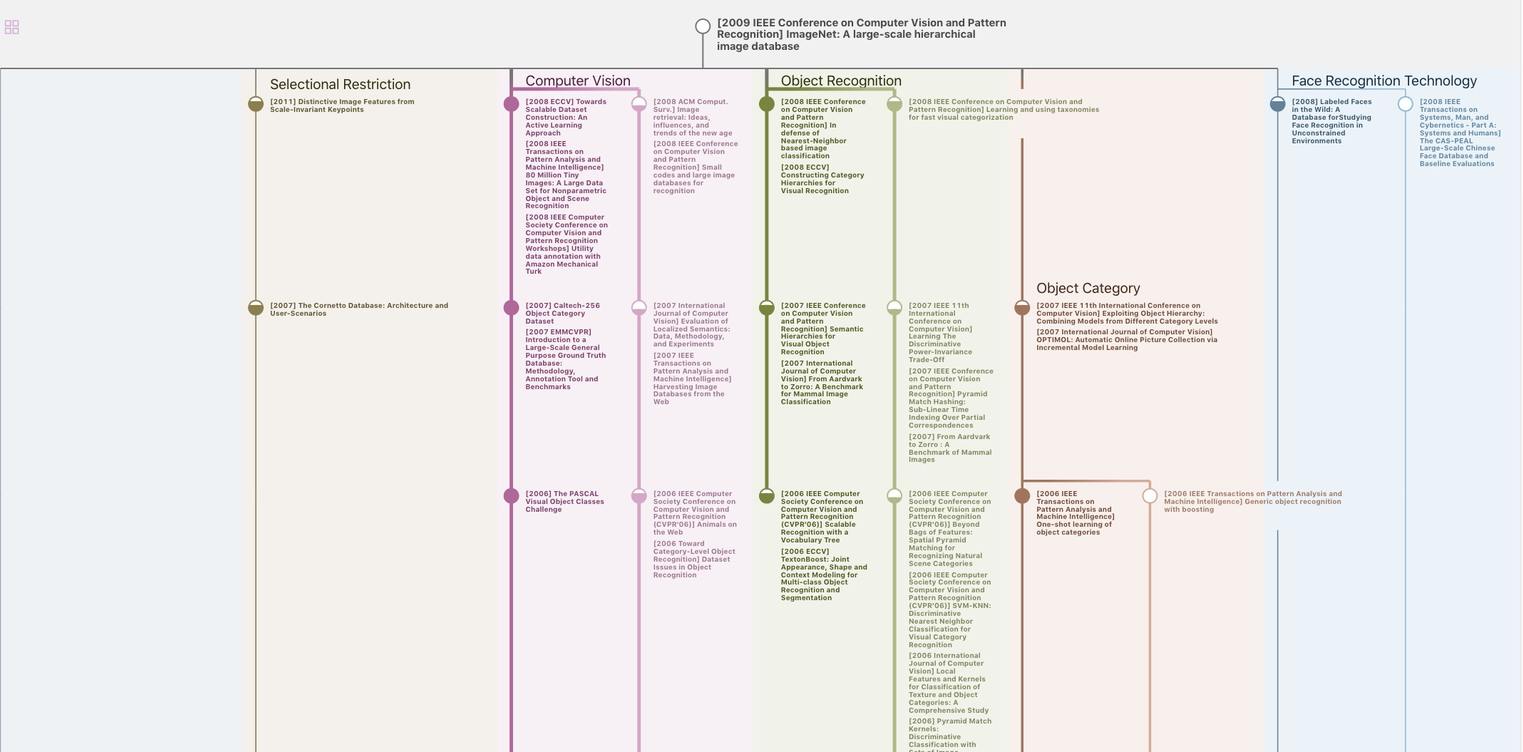
生成溯源树,研究论文发展脉络
Chat Paper
正在生成论文摘要