Genetic Algorithm-Based Dynamic Backdoor Attack on Federated Learning-Based Network Traffic Classification
2023 EIGHTH INTERNATIONAL CONFERENCE ON FOG AND MOBILE EDGE COMPUTING, FMEC(2023)
摘要
Federated learning enables multiple clients to collaboratively contribute to the learning of a global model orchestrated by a central server. This learning scheme promotes clients' data privacy and requires reduced communication overheads. In an application like network traffic classification, this helps hide the network vulnerabilities and weakness points. However, federated learning is susceptible to backdoor attacks, in which adversaries inject manipulated model updates into the global model. These updates inject a salient functionality in the global model that can be launched with specific input patterns. Nonetheless, the vulnerability of network traffic classification models based on federated learning to these attacks remains unexplored. In this paper, we propose GABAttack, a novel genetic algorithm-based backdoor attack against federated learning for network traffic classification. GABAttack utilizes a genetic algorithm to optimize the values and locations of backdoor trigger patterns, ensuring a better fit with the input and the model. This input-tailored dynamic attack is promising for improved attack evasiveness while being effective. Extensive experiments conducted over real-world network datasets validate the success of the proposed GABAttack in various situations while maintaining almost invisible activity. This research serves as an alarming call for network security experts and practitioners to develop robust defense measures against such attacks.
更多查看译文
关键词
Backdoor attack,trigger design,federated learning,network traffic classification,genetic algorithm
AI 理解论文
溯源树
样例
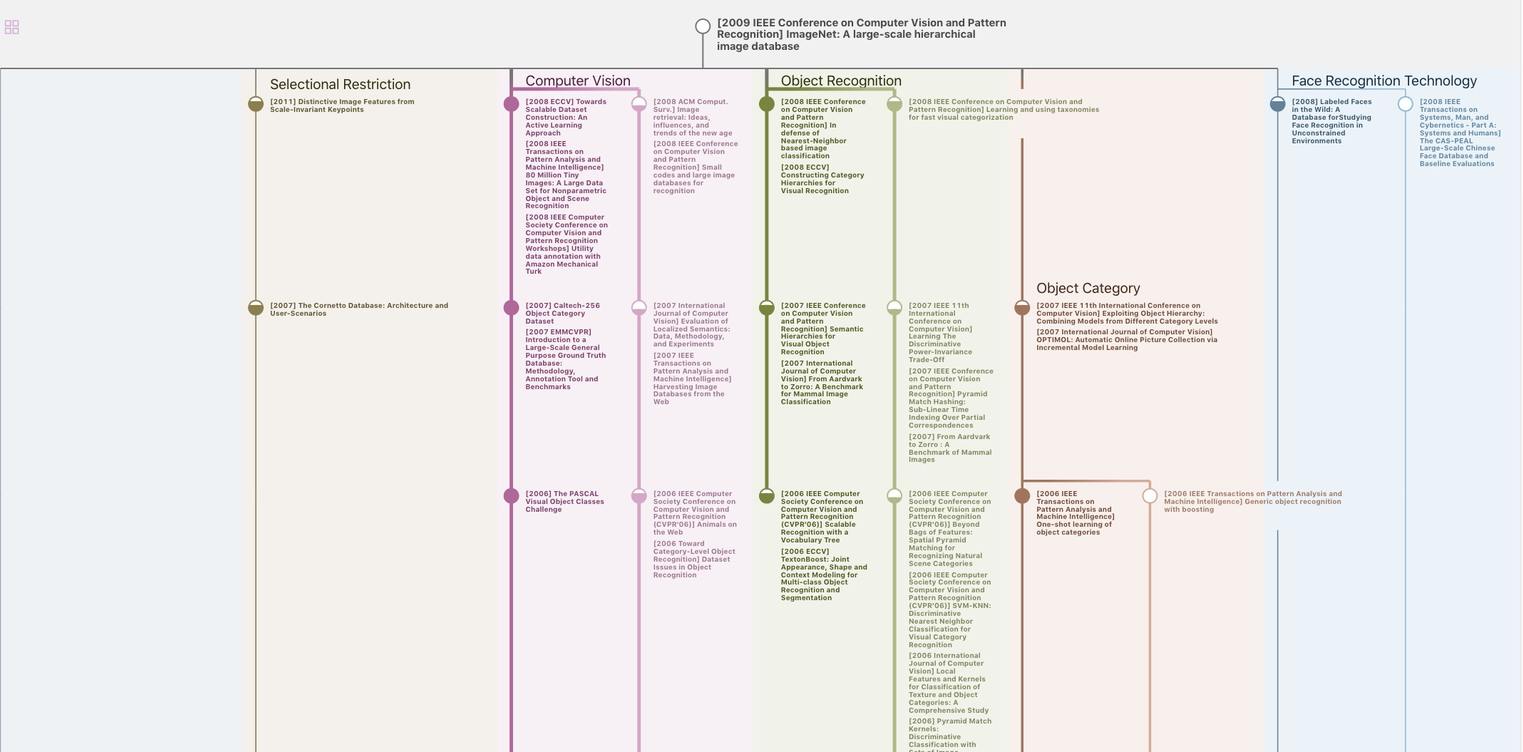
生成溯源树,研究论文发展脉络
Chat Paper
正在生成论文摘要