Collaborative Worker Safety Prediction Mechanism Using Federated Learning Assisted Edge Intelligence in Outdoor Construction Environment
IEEE Access(2023)
摘要
Monitoring construction site safety through physical observations is inherently flawed due to the complex and dynamic nature of construction sites. To overcome these challenges and enhance worker safety management, decentralized model training-assisted edge intelligence emerges as a promising solution. However, despite the potential benefits, our investigation reveals that no research for worker safety prediction has been grounded in the Federated Learning (FL) approach. In this context, we present a novel approach to worker safety prediction, leveraging FL in outdoor construction environments while preserving the privacy and security of sensitive data. Our methodology involves deploying sensor-based IoT devices at construction sites to collect highly granular spatial and temporal weather, building, and worker data. This data is then collaboratively utilized for training Deep Neural Network (DNN) models on the edge nodes in a cross-silos manner. To implement our approach, we establish a test-bed utilizing the EdgeX framework and constrained devices such as Raspberry Pi 4Bs, acting as edge nodes. Following the collaborative training, the resultant global model is deployed on participating nodes for edge inference, ensuring optimal network resource utilization and data privacy. The experimental results demonstrate the efficacy of the proposed approach in improving the utilization of construction safety management systems and reducing the risk of accidents and fatalities in the future. The outcome is a system that exhibits enhanced speed and responsiveness, a crucial aspect for time-sensitive applications such as the prediction of worker safety.
更多查看译文
关键词
Worker safety,outdoor construction site,federated learning,edge computing,EdgeX,Internet of Things
AI 理解论文
溯源树
样例
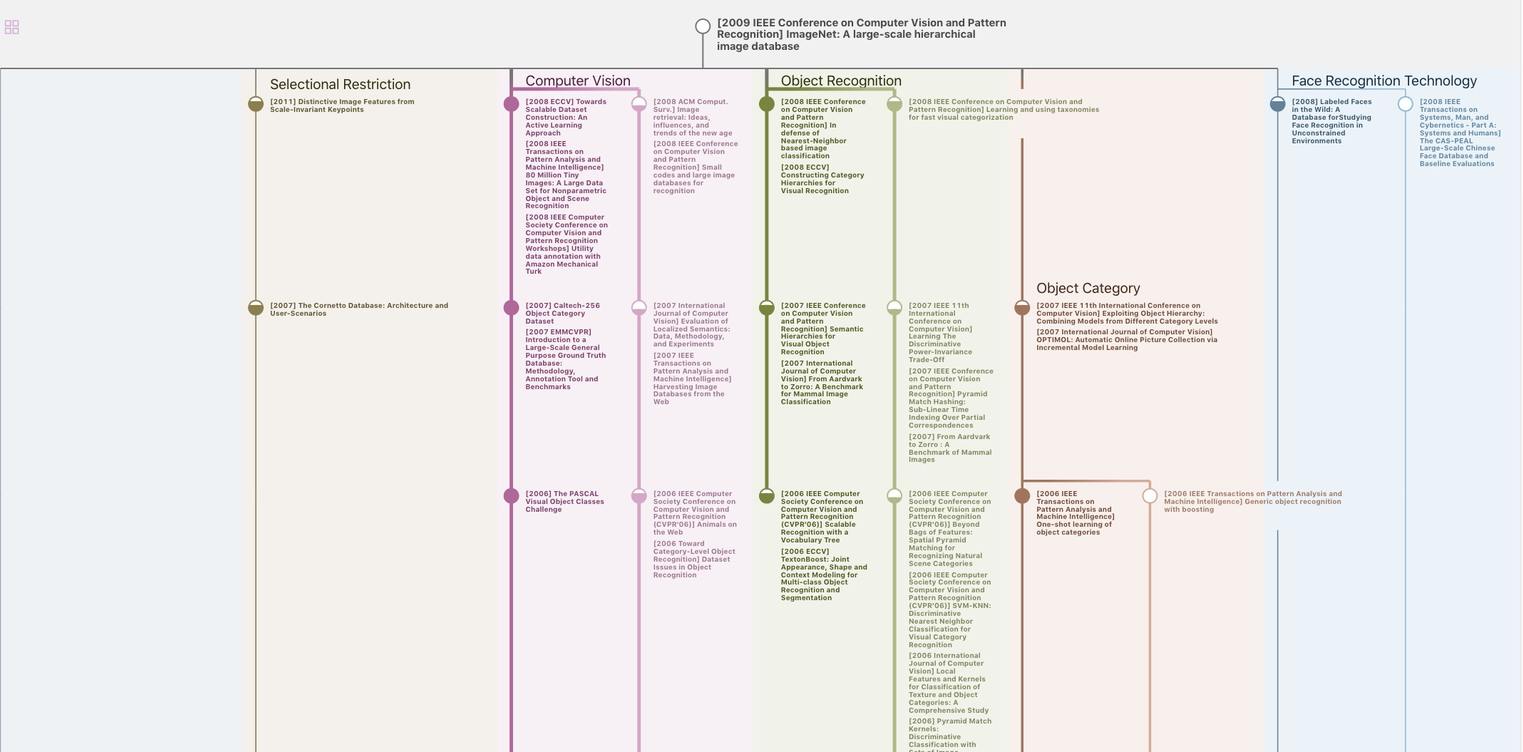
生成溯源树,研究论文发展脉络
Chat Paper
正在生成论文摘要