Few-Shot Specific Emitter Identification Using Asymmetric Masked Auto-Encoder
IEEE COMMUNICATIONS LETTERS(2023)
摘要
Specific emitter identification (SEI) based on radio frequency fingerprint (RFF) characteristics can be used to identify different transmitters, and the deep learning (DL)-based SEI methods have achieved good performance with sufficient samples. However, these methods are difficult to identify emitters when the labeled training samples are limited. Hence, we propose a few-shot SEI (FS-SEI) using asymmetric masked auto-encoder (AMAE) to solve the few-shot problem. Specifically, we use the sufficiently unlabeled training samples which refer as the source domain to drive the training process of AMAE to obtain an RFF extractor with good feature extraction performance on the source domain, and then the pre-trained RFF extractor together with a classifier is fine-tuned using limited labeled training samples which refer as the target domain. Simulation results show that the proposed AMAE-based FS-SEI method achieves state-of-the-art identification performance compared to other supervised and unsupervised methods on the LoRa dataset with 30 categories and WiFi dataset with 16 categories. The codes can be downloaded from GitHub: https://github.com/YZS666/A-Method-for-Solving-the-FS-SEI-Problem .
更多查看译文
关键词
Feature extraction,Training,Gold,Decoding,Wireless communication,Convolution,Communication system security,Few-shot specific emitter identification (FS-SEI),asymmetric masked auto-encoder (AMAE),unsupervised learning,transfer learning
AI 理解论文
溯源树
样例
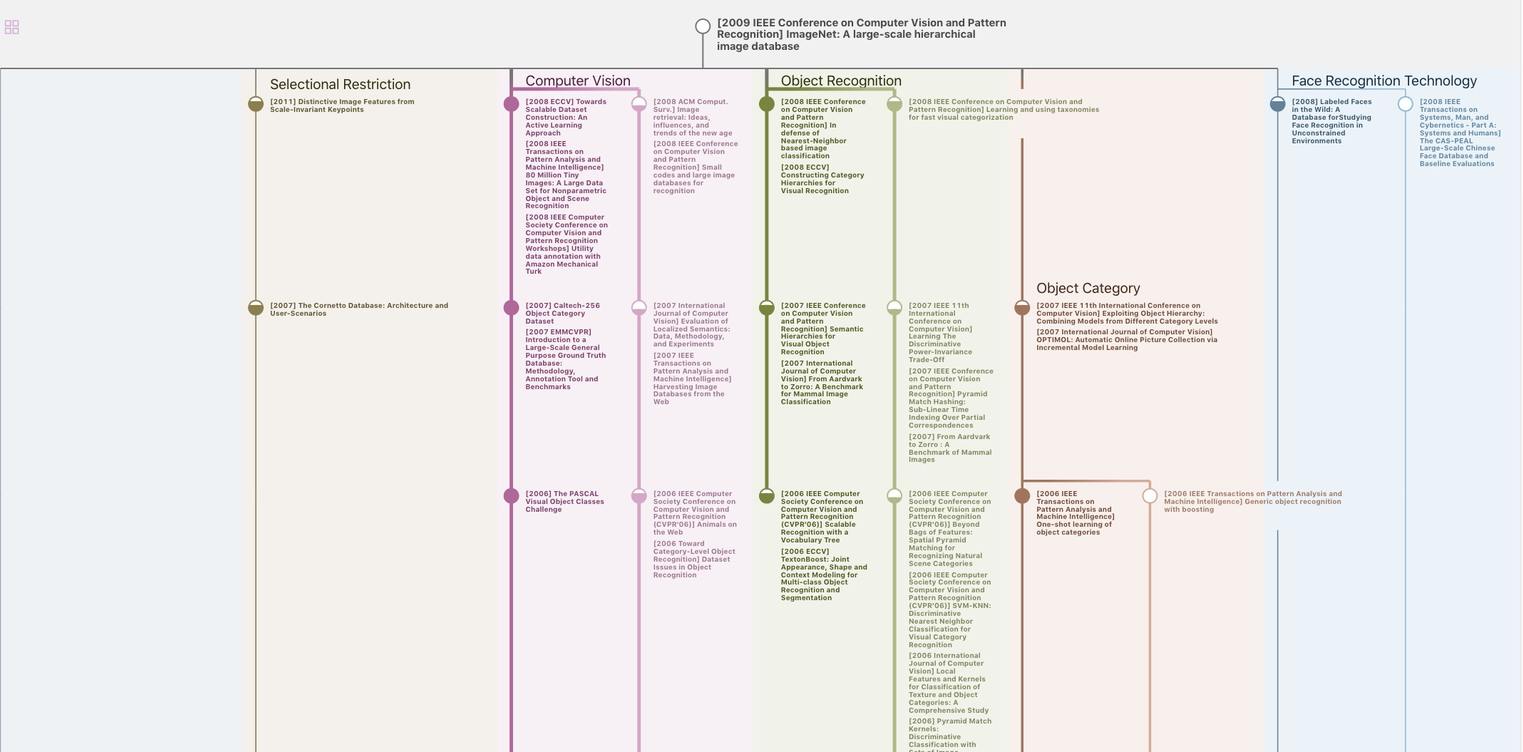
生成溯源树,研究论文发展脉络
Chat Paper
正在生成论文摘要