Sparse topic modeling via spectral decomposition and thresholding
arXiv (Cornell University)(2023)
摘要
The probabilistic Latent Semantic Indexing model assumes that the expectation of the corpus matrix is low-rank and can be written as the product of a topic-word matrix and a word-document matrix. In this paper, we study the estimation of the topic-word matrix under the additional assumption that the ordered entries of its columns rapidly decay to zero. This sparsity assumption is motivated by the empirical observation that the word frequencies in a text often adhere to Zipf's law. We introduce a new spectral procedure for estimating the topic-word matrix that thresholds words based on their corpus frequencies, and show that its $\ell_1$-error rate under our sparsity assumption depends on the vocabulary size $p$ only via a logarithmic term. Our error bound is valid for all parameter regimes and in particular for the setting where $p$ is extremely large; this high-dimensional setting is commonly encountered but has not been adequately addressed in prior literature. Furthermore, our procedure also accommodates datasets that violate the separability assumption, which is necessary for most prior approaches in topic modeling. Experiments with synthetic data confirm that our procedure is computationally fast and allows for consistent estimation of the topic-word matrix in a wide variety of parameter regimes. Our procedure also performs well relative to well-established methods when applied to a large corpus of research paper abstracts, as well as the analysis of single-cell and microbiome data where the same statistical model is relevant but the parameter regimes are vastly different.
更多查看译文
关键词
sparse topic modeling,spectral decomposition
AI 理解论文
溯源树
样例
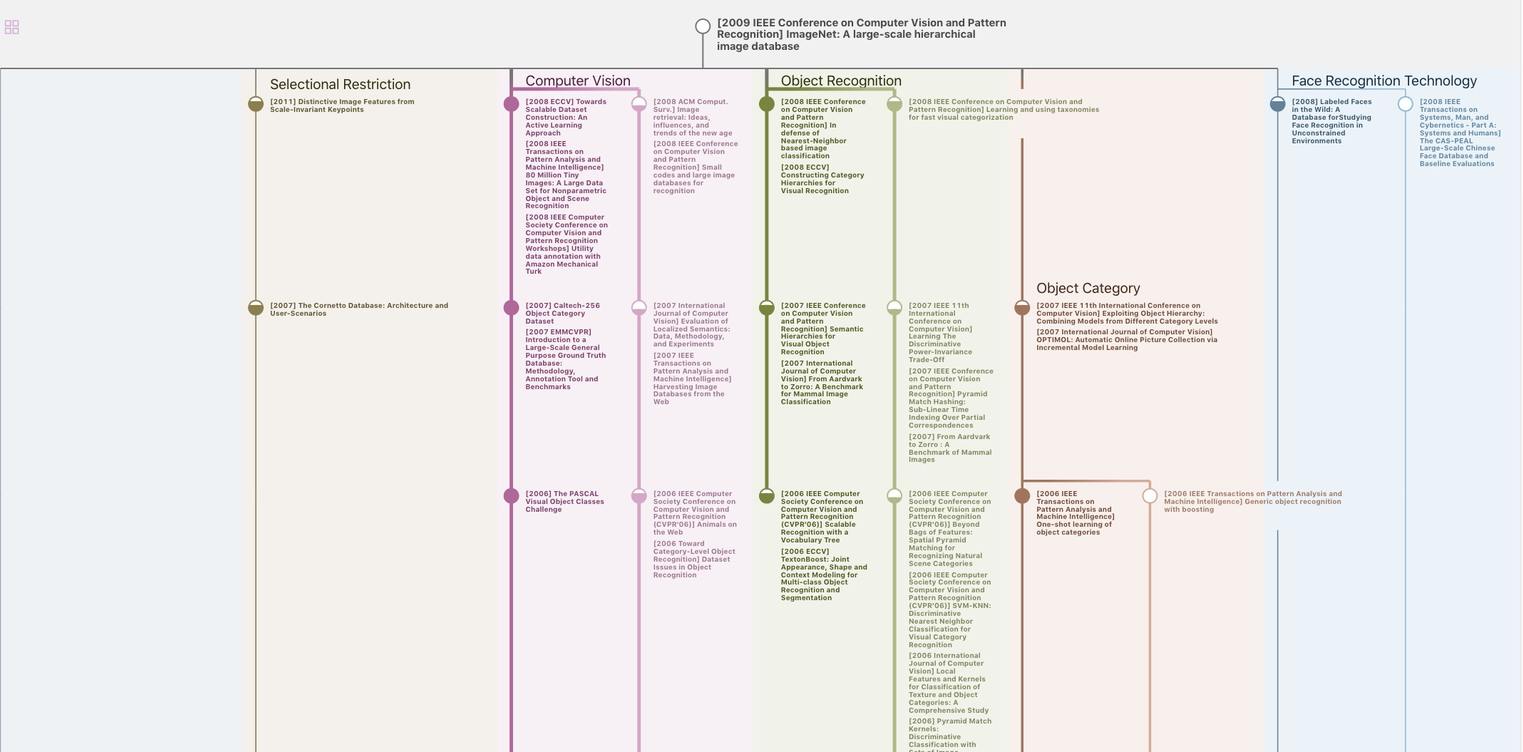
生成溯源树,研究论文发展脉络
Chat Paper
正在生成论文摘要