NECO: NEural Collapse Based Out-of-distribution detection
ICLR 2024(2023)
摘要
Detecting out-of-distribution (OOD) data is a critical challenge in machine learning due to model overconfidence, often without awareness of their epistemological limits. We hypothesize that ``neural collapse'', a phenomenon affecting in-distribution data for models trained beyond loss convergence, also influences OOD data. To benefit from this interplay, we introduce NECO, a novel post-hoc method for OOD detection, which leverages the geometric properties of ``neural collapse'' and of principal component spaces to identify OOD data. Our extensive experiments demonstrate that NECO achieves state-of-the-art results on both small and large-scale OOD detection tasks while exhibiting strong generalization capabilities across different network architectures. Furthermore, we provide a theoretical explanation for the effectiveness of our method in OOD detection. We plan to release the code after the anonymity period.
更多查看译文
关键词
anomaly detection,OOD
AI 理解论文
溯源树
样例
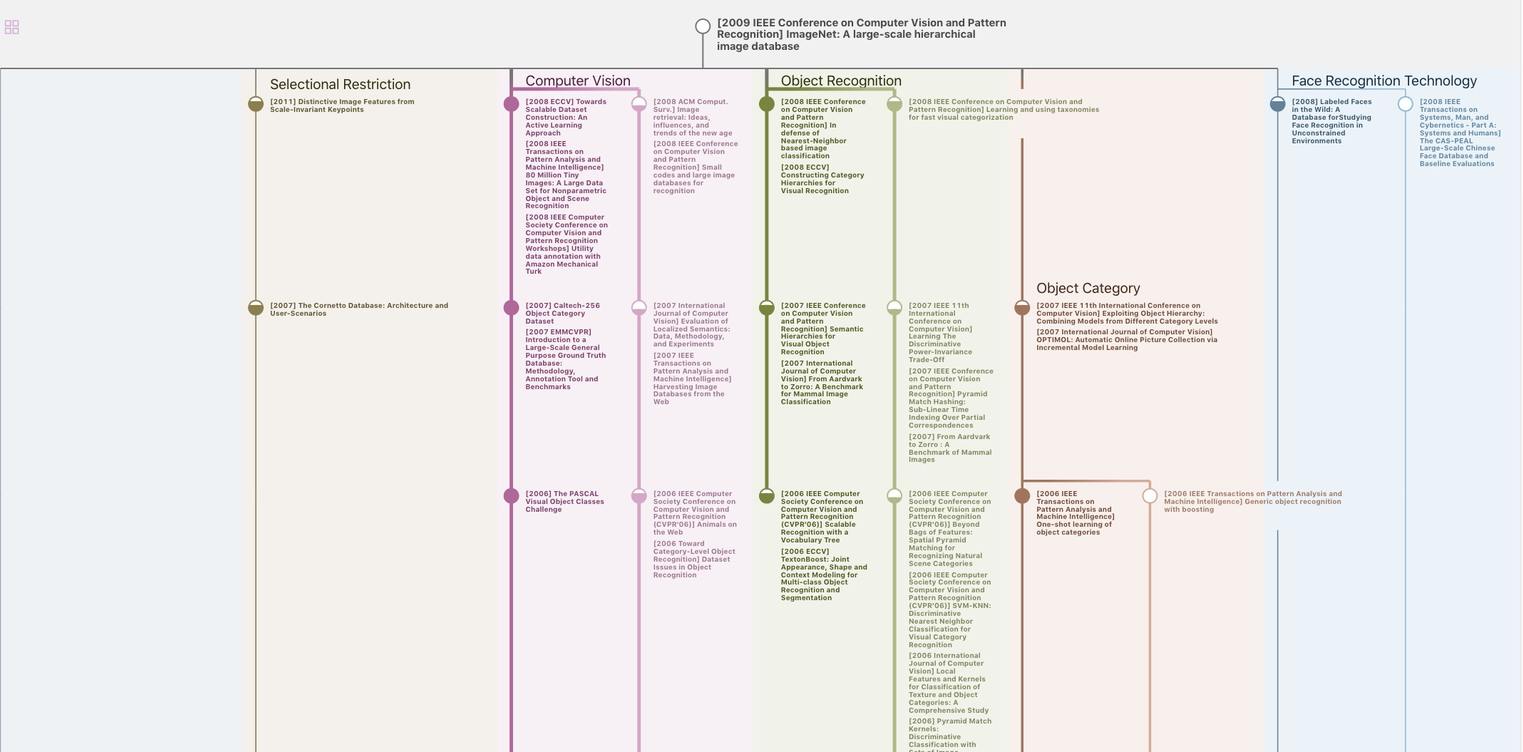
生成溯源树,研究论文发展脉络
Chat Paper
正在生成论文摘要