Robustness May be More Brittle than We Think under Different Degrees of Distribution Shifts
CoRR(2023)
摘要
Out-of-distribution (OOD) generalization is a complicated problem due to the idiosyncrasies of possible distribution shifts between training and test domains. Most benchmarks employ diverse datasets to address this issue; however, the degree of the distribution shift between the training domains and the test domains of each dataset remains largely fixed. This may lead to biased conclusions that either underestimate or overestimate the actual OOD performance of a model. Our study delves into a more nuanced evaluation setting that covers a broad range of shift degrees. We show that the robustness of models can be quite brittle and inconsistent under different degrees of distribution shifts, and therefore one should be more cautious when drawing conclusions from evaluations under a limited range of degrees. In addition, we observe that large-scale pre-trained models, such as CLIP, are sensitive to even minute distribution shifts of novel downstream tasks. This indicates that while pre-trained representations may help improve downstream in-distribution performance, they could have minimal or even adverse effects on generalization in certain OOD scenarios of the downstream task if not used properly. In light of these findings, we encourage future research to conduct evaluations across a broader range of shift degrees whenever possible.
更多查看译文
关键词
robustness,brittle,distribution
AI 理解论文
溯源树
样例
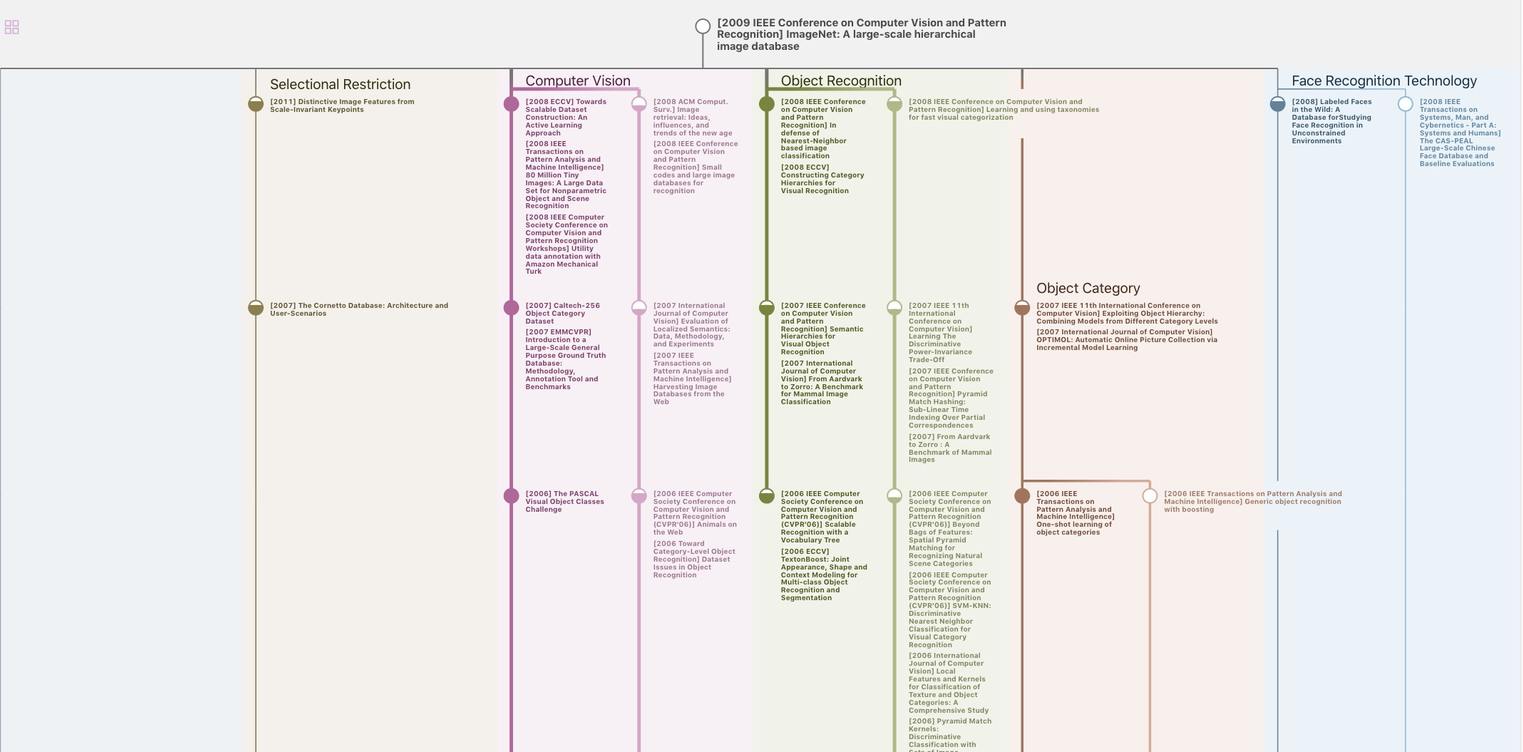
生成溯源树,研究论文发展脉络
Chat Paper
正在生成论文摘要