Query-dominant User Interest Network for Large-Scale Search Ranking
PROCEEDINGS OF THE 32ND ACM INTERNATIONAL CONFERENCE ON INFORMATION AND KNOWLEDGE MANAGEMENT, CIKM 2023(2023)
摘要
Historical behaviors have shown great effect and potential in various prediction tasks, including recommendation and information retrieval. The overall historical behaviors are various but noisy while search behaviors are always sparse. Most existing approaches in personalized search ranking adopt the sparse search behaviors to learn representation with bottleneck, which do not sufficiently exploit the crucial long-term interest. In fact, there is no doubt that user long-term interest is various but noisy for instant search, and how to exploit it well still remains an open problem. To tackle this problem, in this work, we propose a novel model named Query-dominant user Interest Network (QIN), including two cascade units to filter the raw user behaviors and reweigh the behavior subsequences. Specifically, we propose a relevance search unit (RSU), which aims to search a subsequence relevant to the query first and then search the sub-subsequences relevant to the target item. These items are then fed into an attention unit called Fused Attention Unit (FAU). It should be able to calculate attention scores from the ID field and attribute field separately, and then adaptively fuse the item embedding and content embedding based on the user engagement of past period. Extensive experiments and ablation studies on real-world datasets demonstrate the superiority of our model over state-of-the-art methods. The QIN now has been successfully deployed on Kuaishou search, an online video search platform, and obtained 7.6% improvement on CTR.
更多查看译文
关键词
Personalized Search Ranking,User Interest Network,Attention Mechanism
AI 理解论文
溯源树
样例
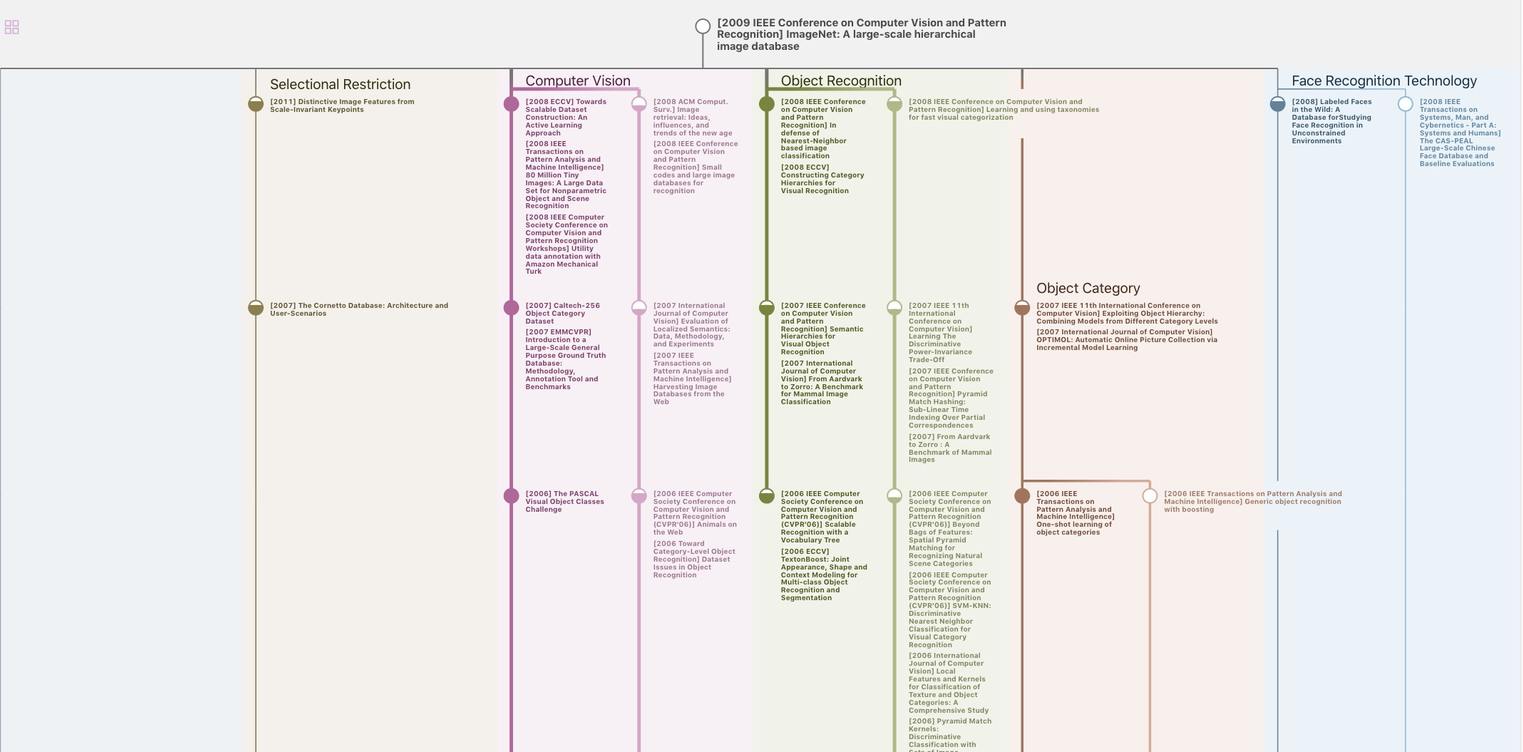
生成溯源树,研究论文发展脉络
Chat Paper
正在生成论文摘要