Paella: Low-latency Model Serving with Software-defined GPU Scheduling
PROCEEDINGS OF THE TWENTY-NINTH ACM SYMPOSIUM ON OPERATING SYSTEMS PRINCIPLES, SOSP 2023(2023)
Abstract
Model serving systems play a critical role in multiplexing machine learning inference jobs across shared GPU infrastructure. These systems have traditionally sat at a high level of abstraction-receiving jobs from clients through a narrow API and relying on black-box GPU scheduling mechanisms when dispatching them. Fundamental limitations in the built-in GPU hardware scheduler, in particular, can lead to inefficiency when executing concurrent jobs. The current abstraction level also incurs system overheads that are similarly most significant when the GPU is heavily shared. In this paper, we argue for co-designing the model compiler, local clients, and the scheduler to bypass the built-in GPU scheduler and enable software control of kernel execution order. Doing so enables the use of arbitrary scheduling algorithms and reduces system overheads throughout the critical path of inference.
MoreTranslated text
Key words
GPUs,low-latency model serving,machine learning inference,scheduling
AI Read Science
Must-Reading Tree
Example
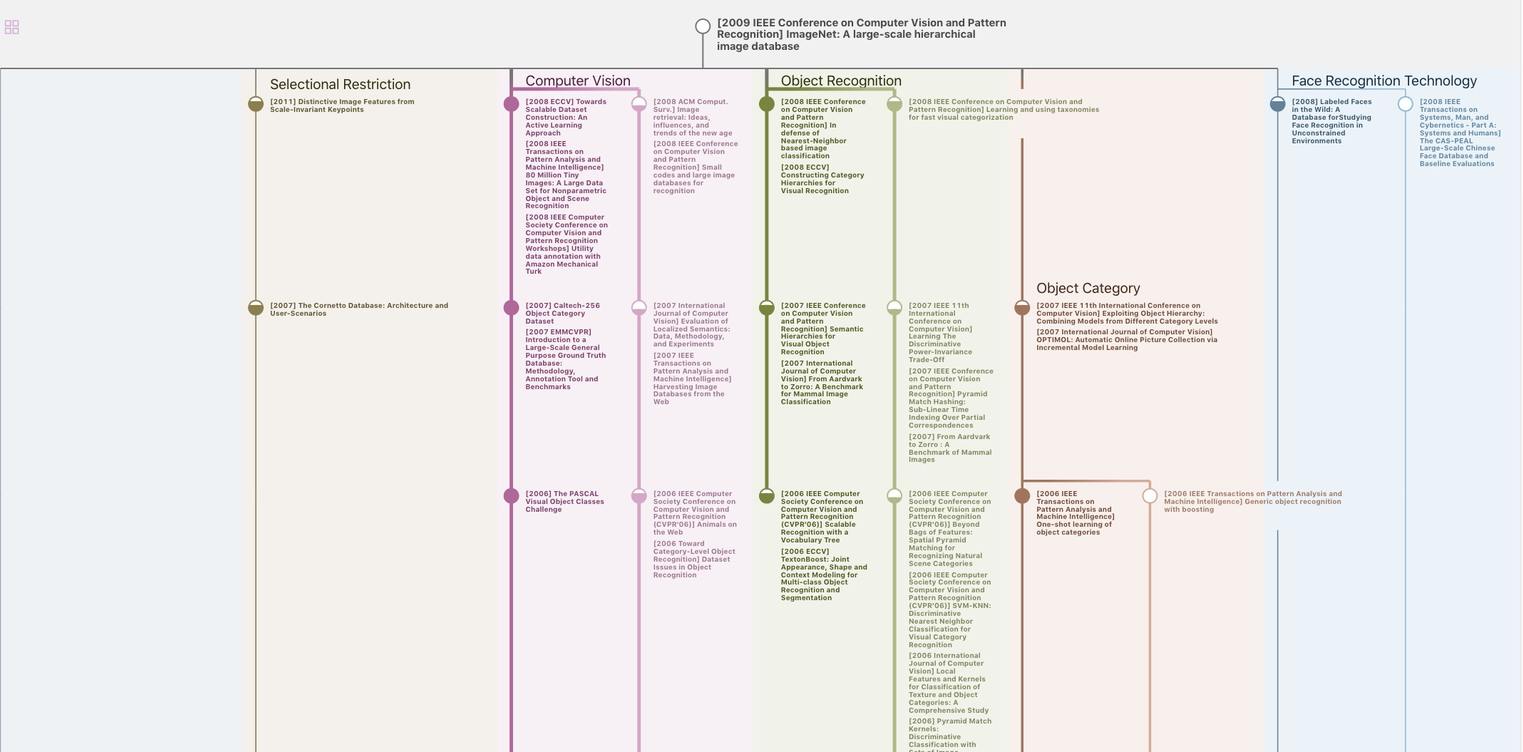
Generate MRT to find the research sequence of this paper
Chat Paper
Summary is being generated by the instructions you defined