A PPA Study of Reinforced Placement Parameter Autotuning: Pseudo-3D vs. True-3D Placers
ACM Transactions on Design Automation of Electronic Systems(2023)
摘要
D Place and Route (P&R) flows either involve true-3D placement algorithms or use commercial 2D tools to transform a 2D design into a 3D design. Irrespective of the nature of the placers, several placement parameters in these tools affect the quality of the final 3D designs. Different parameter settings work well with different circuits, and it is impossible to manually tune them for a particular circuit. Automated approaches involving reinforcement learning have been shown to adapt and learn the parameter settings and create trained models. However, their effectiveness depends on the input dataset quality. Using a set of 10 netlists and 10–21 handpicked placement parameters in P&R flows involving pseudo-3D or true-3D placement, the dataset quality is analyzed. The datasets are the design metrics obtained through different P&R stages, such as placement optimization, clock tree synthesis, or 3D partitioning and global routing. The training runtime and the quality of the final design metrics are compared. On a pseudo-3D flow, the training takes around 126–290 hours, whereas, on a true-3D placer-based flow, it takes around 305–410 hours. It is observed that the datasets obtained from different stages lead to drastically different final design results. With the RL-based training processes, the quality of results in 3D designs improves by up to 23.7% compared to their corresponding untrained P&R flows.
更多查看译文
关键词
reinforced placement parameter autotuning
AI 理解论文
溯源树
样例
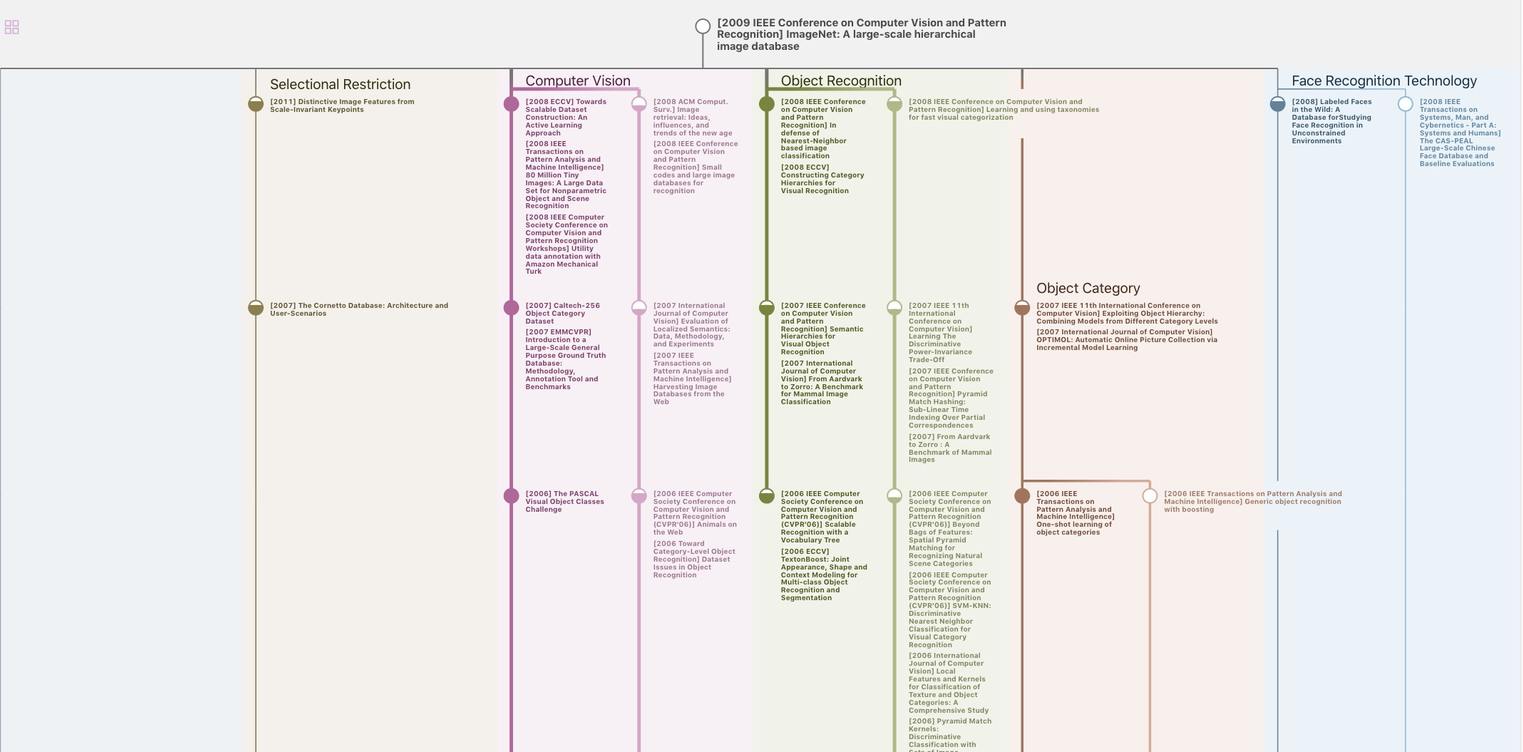
生成溯源树,研究论文发展脉络
Chat Paper
正在生成论文摘要