Modeling and Evaluation of Soft Force Sensors using Recurrent and Feed-Forward Neural Networks and Exponential Methods to Compensate for Force Measurement Error in Curved Conditions
ISWC '23: Proceedings of the 2023 International Symposium on Wearable Computers(2023)
摘要
A wide variety of applications of wearable technology require information about forces and pressures exerted on the body, either by a device (e.g. to sense active wearing periods or to provide feedback to a force-sensitive therapy like compression) or by other objects or body parts (e.g. for bedsore prevention or force-based gait monitoring). However, typical force sensing mechanisms are often difficult to translate to the wearable environment because the geometry and mechanics of body tissues introduce error into the sensor response. Previous studies have shown that soft force sensors are significantly affected by deformation leading to erroneous force measurement, but no effort has been made yet to rectify force data estimated by soft textile-based sensors under deformed conditions. In this study, we model the responses of three low-cost textile-based sensors and one off-the-shelf force-sensitive resistor using an Exponential model, a Recurrent Neural Network (RNN) and a Multi-Layer Perceptron (MLP) Network. Results show that RNN outperforms in modelling hysteresis with an RMSE of 4.2%. Further, we evaluate sensor performance in human-like curvatures, and refine the models by fusing the degree of curvature. Results show that the refined models can reduce measurement error from 46% to 6.8% and from 26.33 to 1.06% in some cases.
更多查看译文
AI 理解论文
溯源树
样例
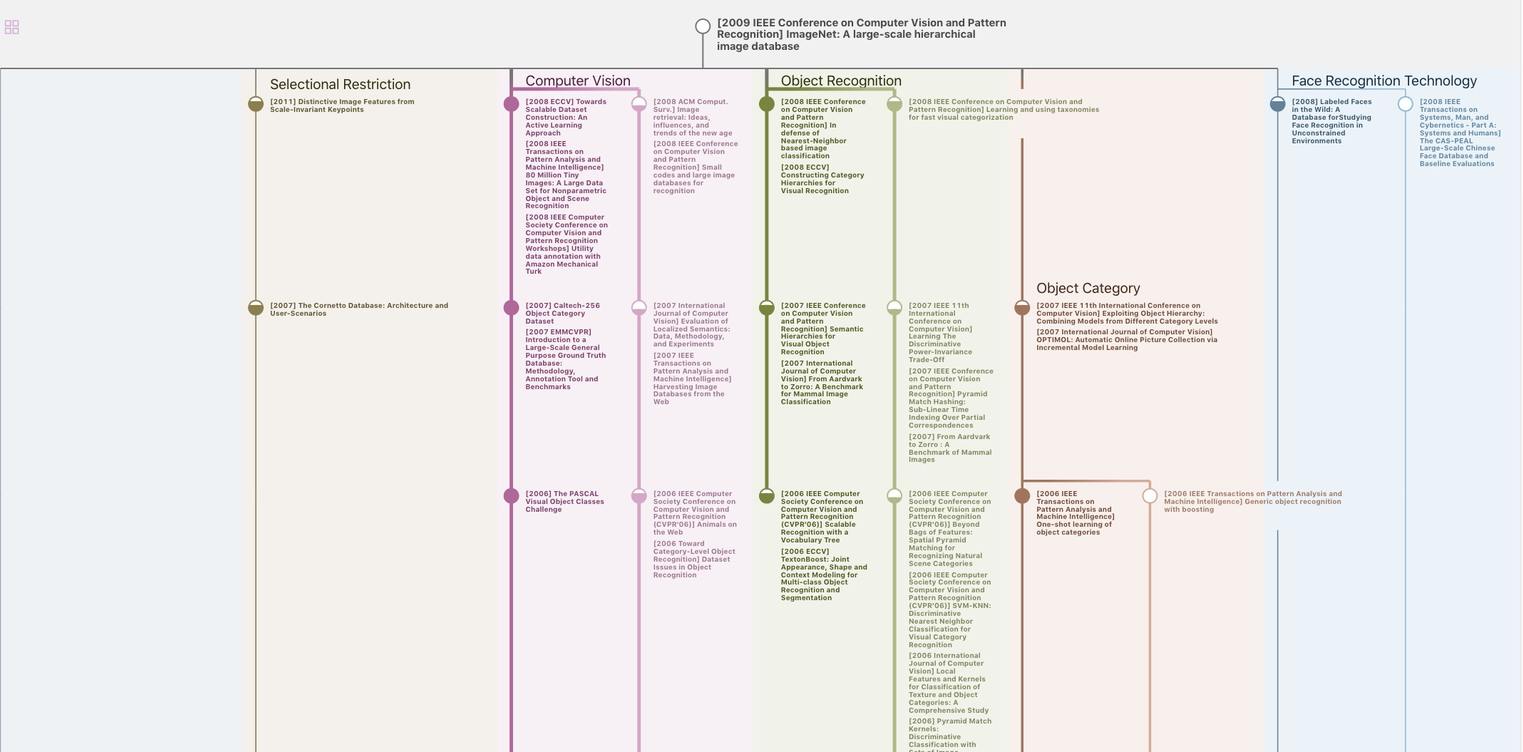
生成溯源树,研究论文发展脉络
Chat Paper
正在生成论文摘要