Communication Resources Limited Decentralized Learning with Privacy Guarantee through Over-the-Air Computation
PROCEEDINGS OF THE 2023 INTERNATIONAL SYMPOSIUM ON THEORY, ALGORITHMIC FOUNDATIONS, AND PROTOCOL DESIGN FOR MOBILE NETWORKS AND MOBILE COMPUTING, MOBIHOC 2023(2023)
摘要
In this paper, we propose a novel decentralized learning algorithm, namely DLLR-OA, for resource-constrained over-the-air computation with formal privacy guarantee. Theoretically, we characterize how the limited resources induced model-components selection error and compound communication errors jointly impact decentralized learning, making the iterates of DLLR-OA converge to a contraction region centered around a scaled version of the errors. In particular, the convergence rate of the DLLR-OA algorithm in the error-free case O(1/root nT) achieves the state-of-the-arts. Besides, we formulate a power control problem and decouple it into two sub-problems of transmitter and receiver to accelerate the convergence of the DLLR-OA algorithm. Furthermore, we provide quantitative privacy guarantee for the proposed over-the-air computation approach. Interestingly, we show that network noise can indeed enhance privacy of aggregated updates while over-the-air computation can further protect individual updates. Finally, the extensive experiments demonstrate that DLLR-OA performs well in the communication resources constrained setting. In particular, numerical results on CIFAR-10 dataset shows nearly 30% communication cost reduction over state-of-the-art baselines with comparable learning accuracy even in resource constrained settings.
更多查看译文
关键词
decentralized learning,over-the-air computation,resource allocation,privacy-preserving
AI 理解论文
溯源树
样例
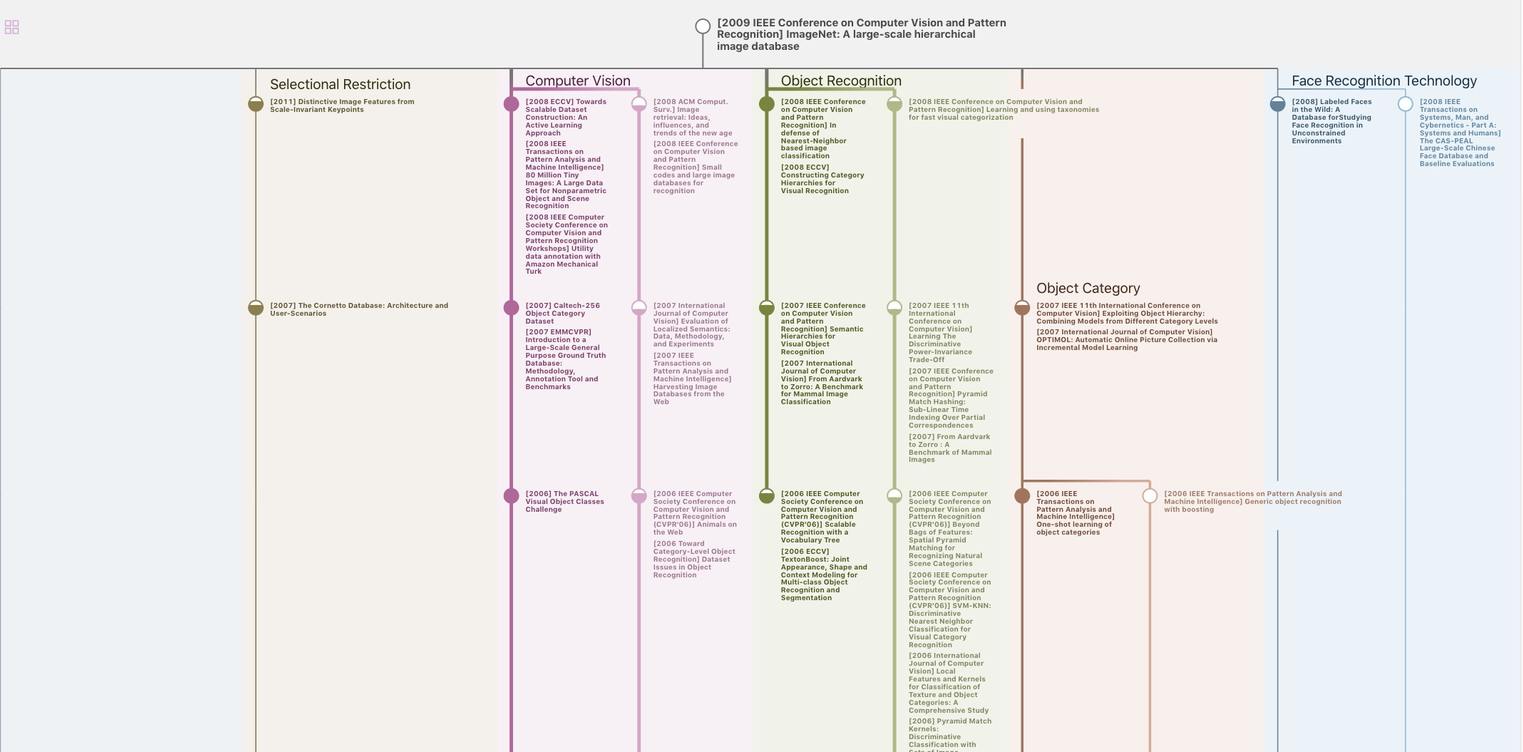
生成溯源树,研究论文发展脉络
Chat Paper
正在生成论文摘要