Waste Not, Want Not: Service Migration-Assisted Federated Intelligence for Multi-Modality Mobile Edge Computing
PROCEEDINGS OF THE 2023 INTERNATIONAL SYMPOSIUM ON THEORY, ALGORITHMIC FOUNDATIONS, AND PROTOCOL DESIGN FOR MOBILE NETWORKS AND MOBILE COMPUTING, MOBIHOC 2023(2023)
摘要
Future mobile edge computing (MEC) is envisioned to provide federated intelligence to delay-sensitive learning tasks with multi-modal data. Conventional horizontal federated learning (FL) suffers from high resource demand in response to complicated multi-modal models. Multi-modal FL (MFL), on the other hand, offers a more efficient approach for learning from multi-modal data. In MFL, the entire multi-modal model is split into several sub-models with each tailored to a specific data modality and trained on a designated edge. As sub-models are considerably smaller than the multi-modal model, MFL requires fewer computation resources and reduces communication time. Nevertheless, deploying MFL over MEC faces the challenges of device mobility and edge heterogeneity, which, if not addressed, could negatively impact MFL performance. In this paper, we investigate an Service Migration-assisted Mobile Multi-modal Federated Learning (SM3FL) framework, where the service migration for sub-models between edges is enabled. To effectively utilize both communication and computation resources without extravagance in SM3FL, we develop the optimal strategies of service migration and data sample collection to minimize the wall-clock time, defined as the required training time to reach the learning target. Our experiment results show that the proposed SM3FL framework demonstrates remarkable performance, surpassing other state-of-art FL frameworks via substantially reducing the computing demand by 17.5% and dramatically decreasing the wall-clock time by 25.3%.
更多查看译文
关键词
Mobile edge computing,Multi-modality data,Federated learning
AI 理解论文
溯源树
样例
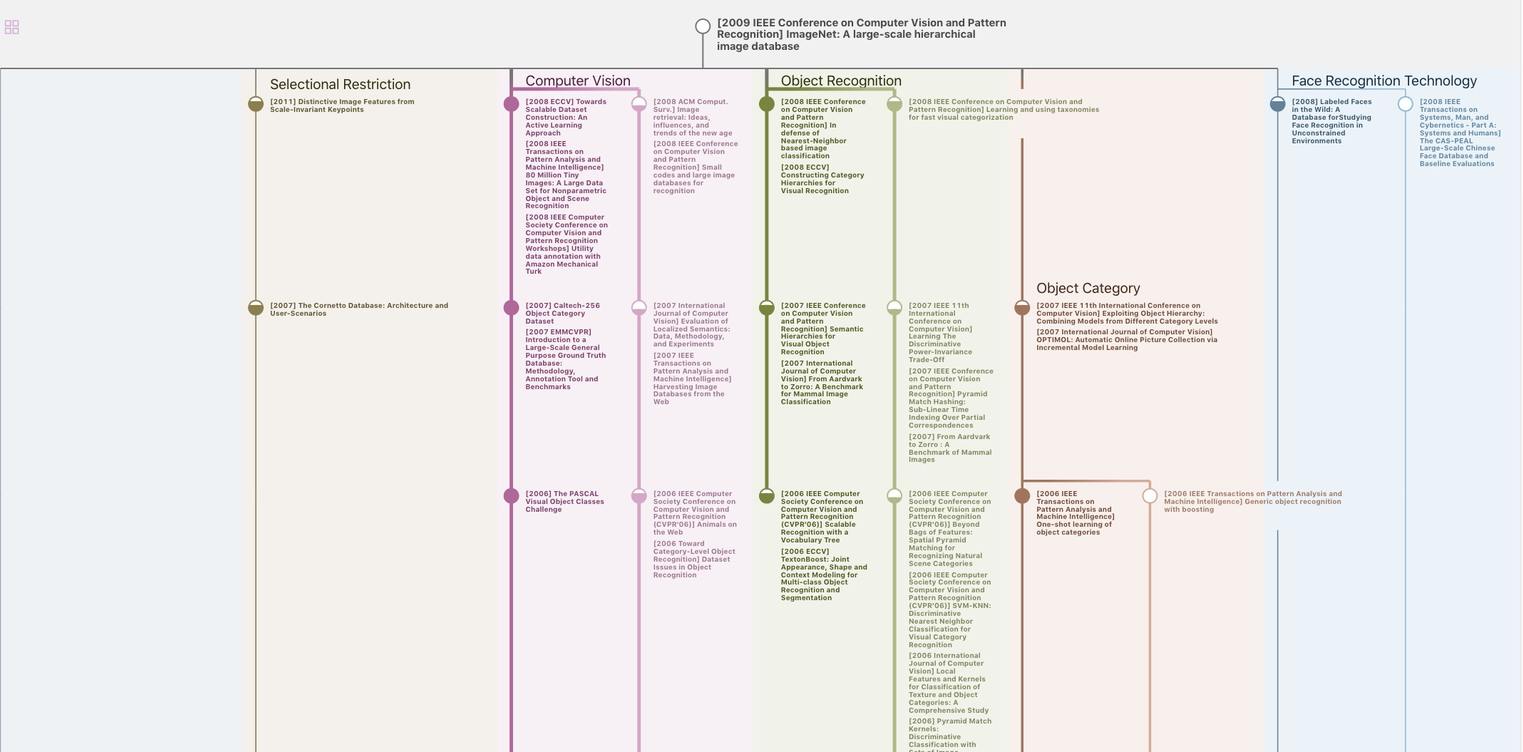
生成溯源树,研究论文发展脉络
Chat Paper
正在生成论文摘要