MSVEC: A Multidomain Testing Dataset for Scientific Claim Verification
PROCEEDINGS OF THE 2023 INTERNATIONAL SYMPOSIUM ON THEORY, ALGORITHMIC FOUNDATIONS, AND PROTOCOL DESIGN FOR MOBILE NETWORKS AND MOBILE COMPUTING, MOBIHOC 2023(2023)
摘要
The increase of disinformation in scientific news across a variety of domains has generated an urgency for a robust and generalizable approach to automated scientific claim verification (SCV). Available methods of SCV are limited in either domain adaptability or scalability. To facilitate building and evaluating more robust models on SCV we propose MSVEC, a multidomain dataset containing 200 pairs of verified scientific news claims with evidence research papers. To understand the capability of large language models on the SCV task, we evaluated GPT-3.5 against MSVEC. While methods of fact-checking exist for specific domains (e.g., political and health), the use of large language models exhibits better generalizability across multiple domains and is potentially compared with state-of-the-art models based on word embeddings. The data and software used and developed for this project are available at https://github.com/lamps-lab/msvec.
更多查看译文
关键词
benchmark datasets,natural language processing,large language models,machine learning
AI 理解论文
溯源树
样例
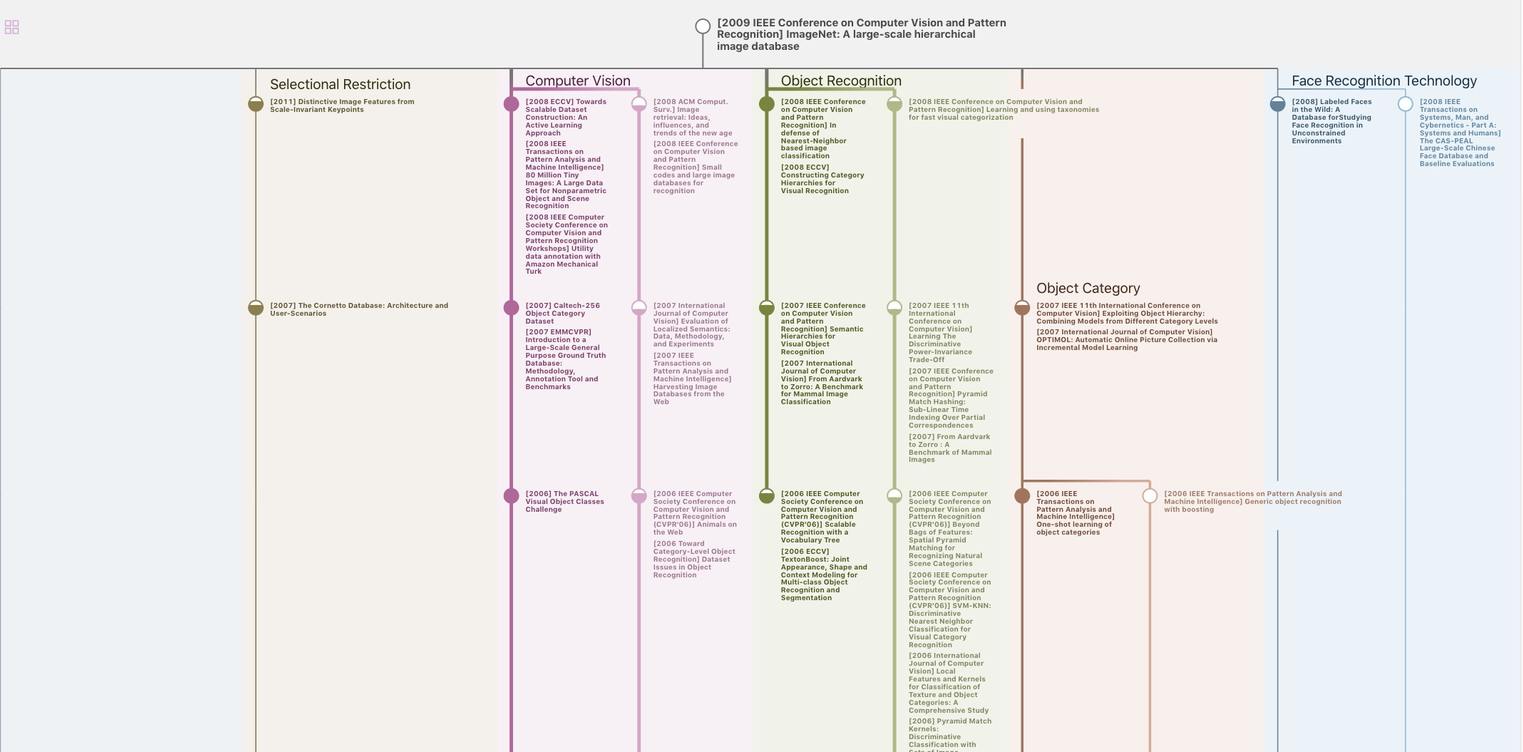
生成溯源树,研究论文发展脉络
Chat Paper
正在生成论文摘要