How Noisy is Too Noisy? The Impact of Data Noise on Multimodal Recognition of Confusion and Conflict During Collaborative Learning
PROCEEDINGS OF THE 25TH INTERNATIONAL CONFERENCE ON MULTIMODAL INTERACTION, ICMI 2023(2023)
摘要
Intelligent systems to support collaborative learning rely on real-time behavioral data, including language, audio, and video. However, noisy data, such as word errors in speech recognition, audio static or background noise, and facial mistracking in video, often limit the utility of multimodal data. It is an open question of how we can build reliable multimodal models in the face of substantial data noise. In this paper, we investigate the impact of data noise on the recognition of confusion and conflict moments during collaborative programming sessions by 25 dyads of elementary school learners. We measure language errors with word error rate (WER), audio noise with speech-to-noise ratio (SNR), and video errors with frame-by-frame facial tracking accuracy. The results showed that the model's accuracy for detecting confusion and conflict in the language modality decreased drastically from 0.84 to 0.73 when the WER exceeded 20%. Similarly, in the audio modality, the model's accuracy decreased sharply from 0.79 to 0.61 when the SNR dropped below 5 dB. Conversely, the model's accuracy remained relatively constant in the video modality at a comparable level (> 0.70) so long as at least one learner's face was successfully tracked. Moreover, we trained several multimodal models and found that integrating multimodal data could effectively offset the negative effect of noise in unimodal data, ultimately leading to improved accuracy in recognizing confusion and conflict. These findings have practical implications for the future deployment of intelligent systems that support collaborative learning in actual classroom settings.
更多查看译文
关键词
Data Noise,Multimodal Fusion,Collaborative Learning
AI 理解论文
溯源树
样例
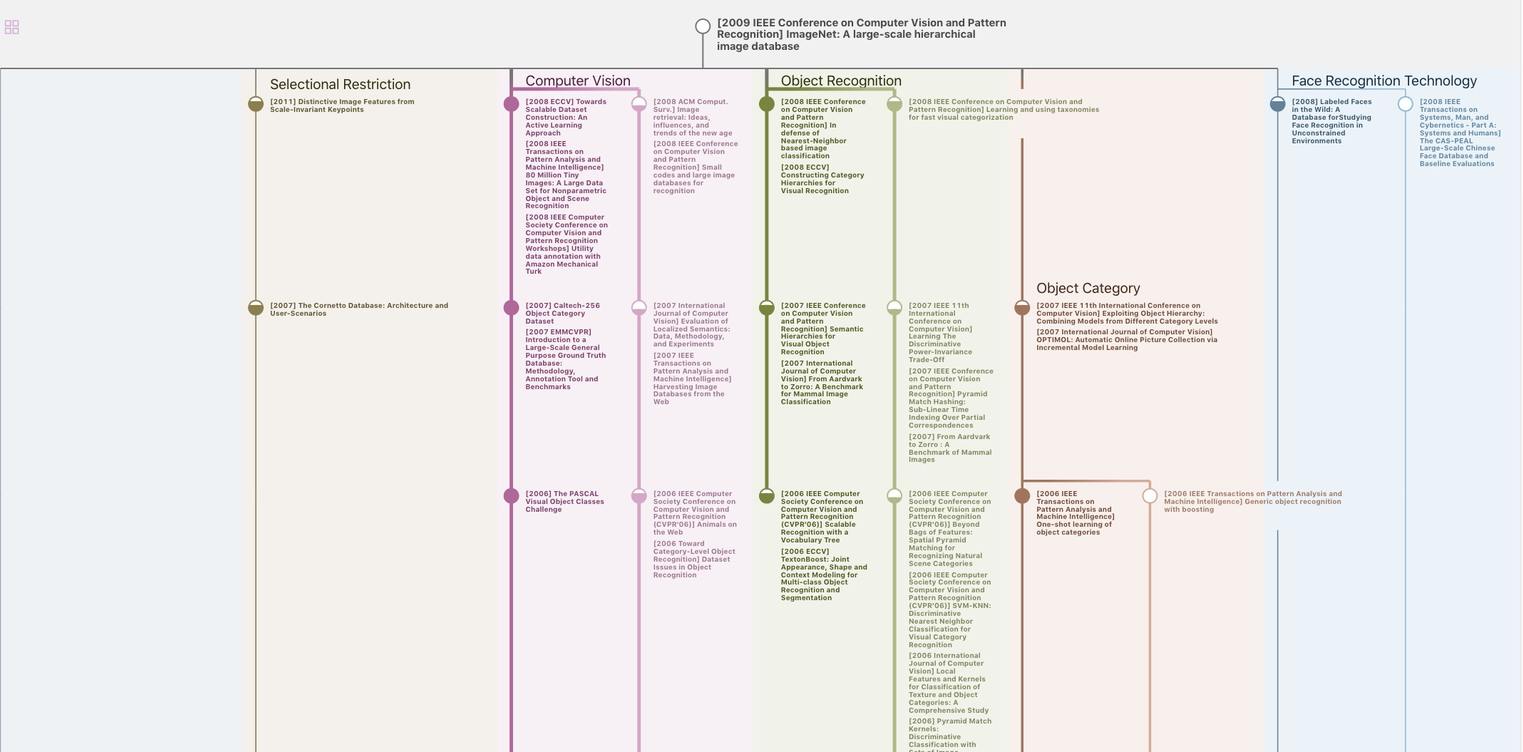
生成溯源树,研究论文发展脉络
Chat Paper
正在生成论文摘要