Offloading Machine Learning to Programmable Data Planes: A Systematic Survey
ACM COMPUTING SURVEYS(2024)
摘要
The demand for machine learning (ML) has increased significantly in recent decades, enabling several applications, such as speech recognition, computer vision, and recommendation engines. As applications become more sophisticated, the models trained become more complex while also increasing the amount of data used for training. Several domain-specific techniques can be helpful to scale machine learning to large amounts of data and more complex models. Among the methods employed, of particular interest is offloading machine learning functionality to the network infrastructure, which is enabled by the use of emerging programmable data plane hardware, such as SmartNICs and programmable switches. As such, offloading machine learning to programmable network hardware has attracted considerable attention from the research community in the last few years. This survey presents a study of programmable data planes applied to machine learning, also highlighting how in-network computing is helping to speed up machine learning applications. In this article, we provide various concepts and propose a taxonomy to classify existing research. Next, we systematically review the literature that offloads machine learning functionality to programmable data plane devices, classifying it based on our proposed taxonomy. Finally, we discuss open challenges in the field and suggest directions for future research.
更多查看译文
关键词
In-network computing,Programmable NICs,programmable switches,offloading,ML training,ML inference
AI 理解论文
溯源树
样例
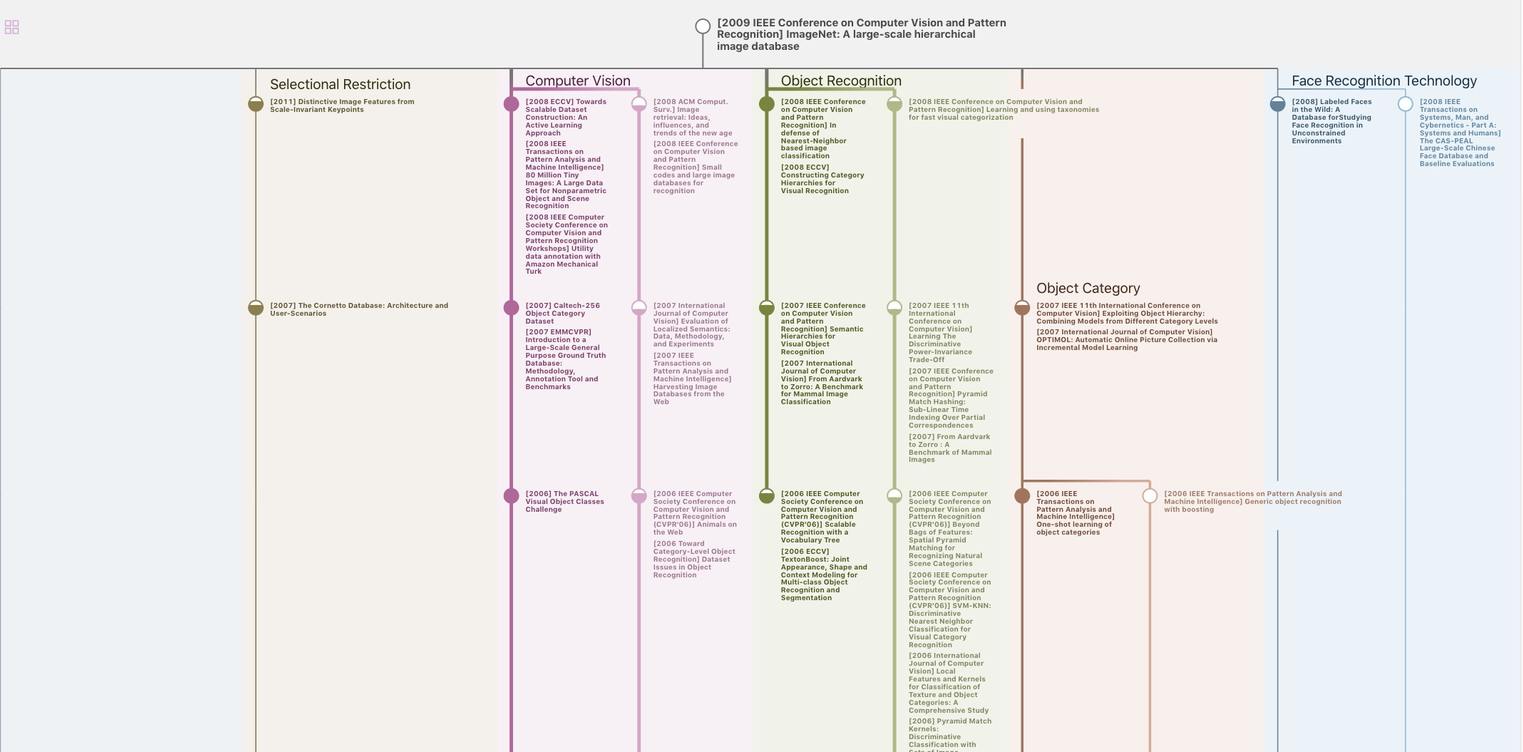
生成溯源树,研究论文发展脉络
Chat Paper
正在生成论文摘要