Robust Hashing via Global and Local Invariant Features for Image Copy Detection
ACM TRANSACTIONS ON MULTIMEDIA COMPUTING COMMUNICATIONS AND APPLICATIONS(2024)
摘要
Robust hashing is a powerful technique for processing large-scale images. Currently, many reported image hashing schemes do not perform well in balancing the performances of discrimination and robustness, and thus they cannot efficiently detect image copies, especially the image copies with multiple distortions. To address this, we exploit global and local invariant features to develop a novel robust hashing for image copy detection. A critical contribution is the global feature calculation by gray level co-occurrence moment learned from the saliency map determined by the phase spectrum of quaternion Fourier transform, which can significantly enhance discrimination without reducing robustness. Another essential contribution is the local invariant feature computation via Kernel Principal Component Analysis (KPCA) and vector distances. As KPCA can maintain the geometric relationships within image, the local invariant features learned with KPCA and vector distances can guarantee discrimination and compactness. Moreover, the global and local invariant features are encrypted to ensure security. Finally, the hash is produced via the ordinal measures of the encrypted features for making a short length of hash. Numerous experiments are conducted to show efficiency of our scheme. Compared with some well-known hashing schemes, our scheme demonstrates a preferable classification performance of discrimination and robustness. The experiments of detecting image copies with multiple distortions are tested and the results illustrate the effectiveness of our scheme.
更多查看译文
关键词
Robust hashing,saliency map,invariant features,image copy detection,dimension reduction
AI 理解论文
溯源树
样例
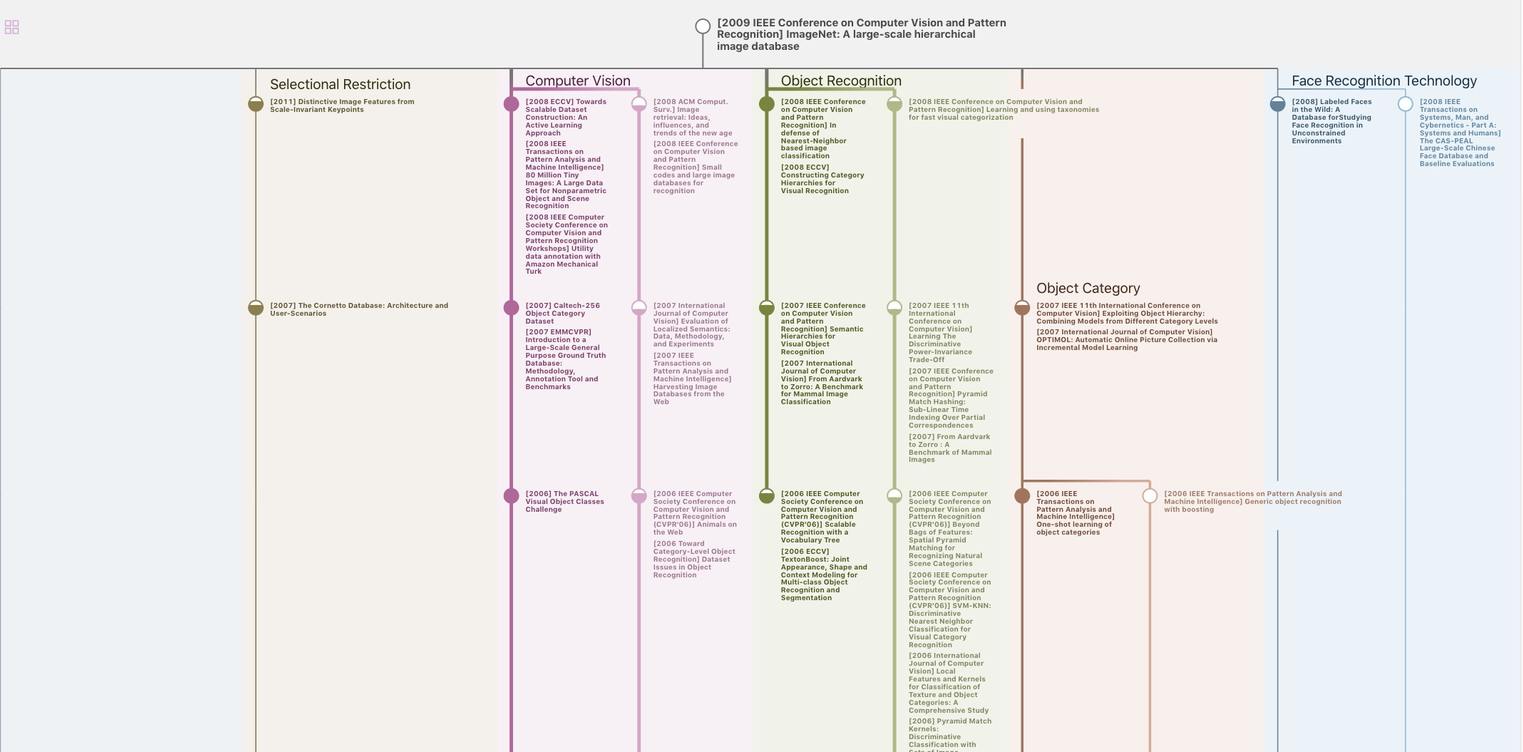
生成溯源树,研究论文发展脉络
Chat Paper
正在生成论文摘要