Cascading Blend Network for Image Inpainting
ACM TRANSACTIONS ON MULTIMEDIA COMPUTING COMMUNICATIONS AND APPLICATIONS(2024)
摘要
Image inpainting refers to filling in unknown regions with known knowledge, which is in full flourish accompanied by the popularity and prosperity of deep convolutional networks. Current inpainting methods have excelled in completing small-sized corruption or specifically masked images. However, for large-proportion corrupted images, most attention-based and structure-based approaches, though reported with state-of-the-art performance, fail to reconstruct high-quality results due to the short consideration of semantic relevance. To relieve the above problem, in this paper, we propose a novel image inpainting approach, namely cascading blend network (CBNet), to strengthen the capacity of feature representation. As a whole, we introduce an adjacent transfer attention (ATA) module in the decoder, which preserves contour structure reasonably from the deep layer and blends structure-texture information from the shadow layer. In a coarse to delicate manner, a multi-scale contextual blend (MCB) block is further designed to felicitously assemble the multi-stage feature information. In addition, to ensure a high qualified hybrid of the feature information, extra deep supervision is applied to the intermediate features through a cascaded loss. Qualitative and quantitative experiments on the Paris StreetView, CelebA, and Places2 datasets demonstrate the superior performance of our approach compared with most state-of-the-art algorithms.
更多查看译文
关键词
Image inpainting,deep convolutional networks,large-proportion corrupted images,attention-based,multi-scale context blend
AI 理解论文
溯源树
样例
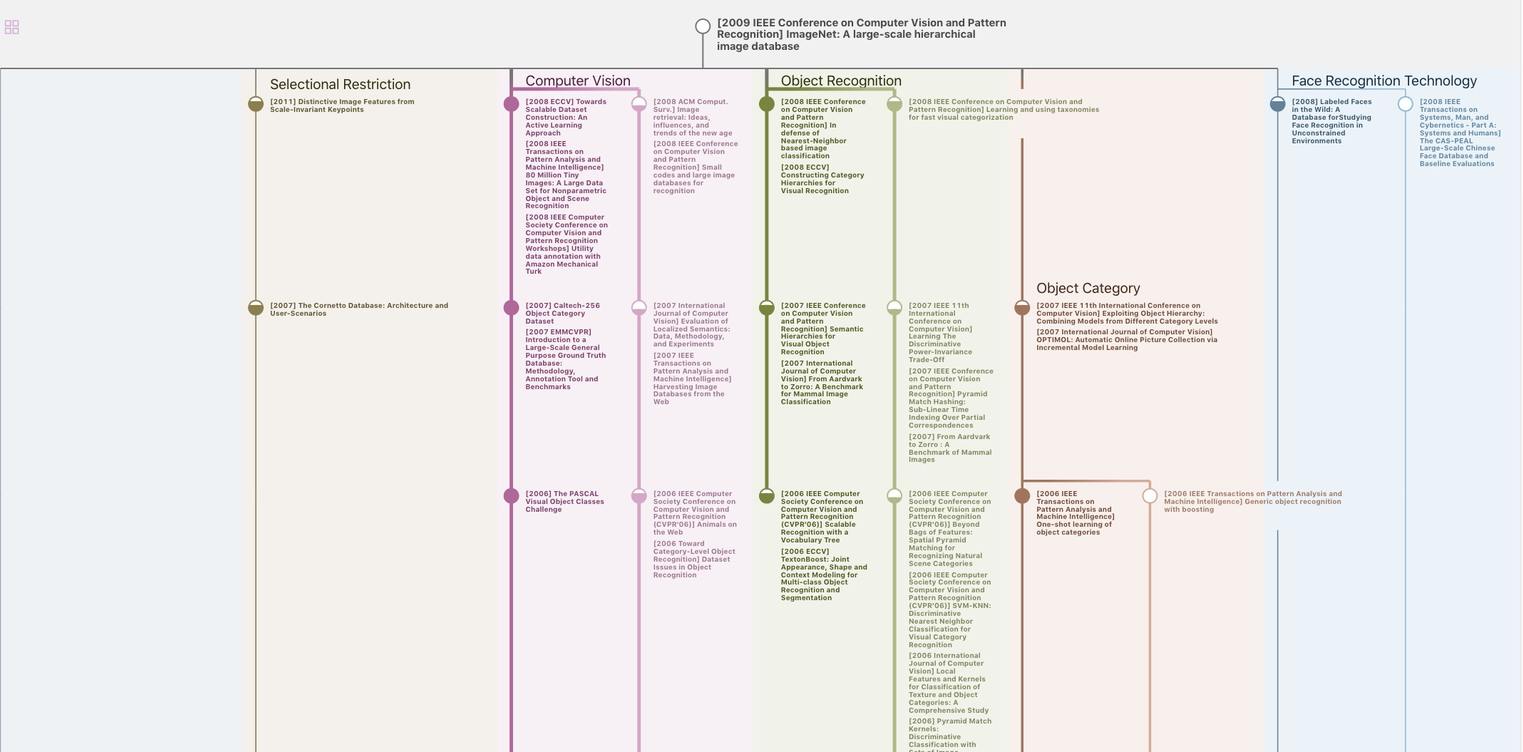
生成溯源树,研究论文发展脉络
Chat Paper
正在生成论文摘要