A Learning-Based Framework for Safe Human-Robot Collaboration with Multiple Backup Control Barrier Functions
ICRA 2024(2024)
摘要
Ensuring robot safety in complex environments is a difficult task due to actuation limits, such as torque bounds. This paper presents a safety-critical control framework that leverages learning-based switching between multiple backup controllers to formally guarantee safety under bounded control inputs while satisfying driver intention. By leveraging {em backup controllers} designed to uphold safety and input constraints, textit{backup control barrier functions} (BCBFs) construct implicitly defined control invariant sets via a feasible quadratic program (QP). However, BCBF performance largely depends on the design and conservativeness of the chosen backup controller, especially in our setting of human-driven vehicles in complex, e.g, off-road, conditions. While conservativeness can be reduced by using multiple backup controllers, determining when to switch is an open problem. Consequently, we develop a broadcast scheme that estimates driver intention and integrates BCBFs with multiple backup strategies for human-robot interaction. An LSTM classifier uses data inputs from the robot, human, and safety algorithms to continually choose a backup controller in real-time. We demonstrate our method's efficacy on a dual-track robot in obstacle avoidance scenarios. Our framework guarantees robot safety while adhering to driver intention.
更多查看译文
关键词
Robot Safety,Safety in HRI,Human-Robot Collaboration
AI 理解论文
溯源树
样例
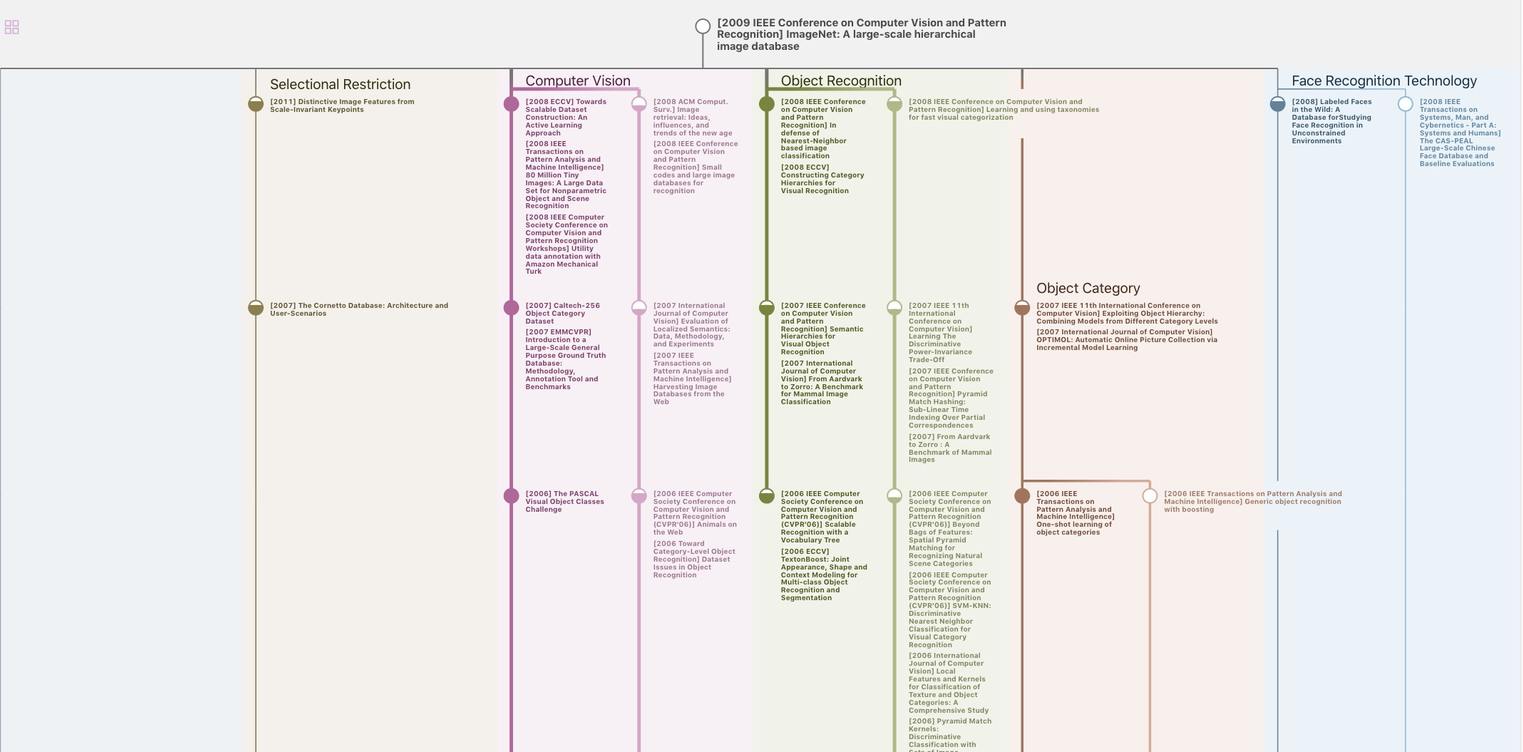
生成溯源树,研究论文发展脉络
Chat Paper
正在生成论文摘要