Provable Compositional Generalization for Object-Centric Learning
ICLR 2024(2023)
Abstract
Learning representations that generalize to novel compositions of known concepts is crucial for bridging the gap between human and machine perception. One prominent effort is learning object-centric representations, which are widely conjectured to enable compositional generalization. Yet, it remains unclear when this conjecture will be true, as a principled theoretical or empirical understanding of compositional generalization is lacking. In this work, we investigate when compositional generalization is guaranteed for object-centric representations through the lens of identifiability theory. We show that autoencoders that satisfy structural assumptions on the decoder and enforce encoder-decoder consistency will learn object-centric representations that provably generalize compositionally. We validate our theoretical result and highlight the practical relevance of our assumptions through experiments on synthetic image data.
MoreTranslated text
Key words
compositional generalization,identifiability,object-centric learning,generalization,OOD generalization,unsupervised learning,slot attention,disentanglement,autoencoders,representation learning
AI Read Science
Must-Reading Tree
Example
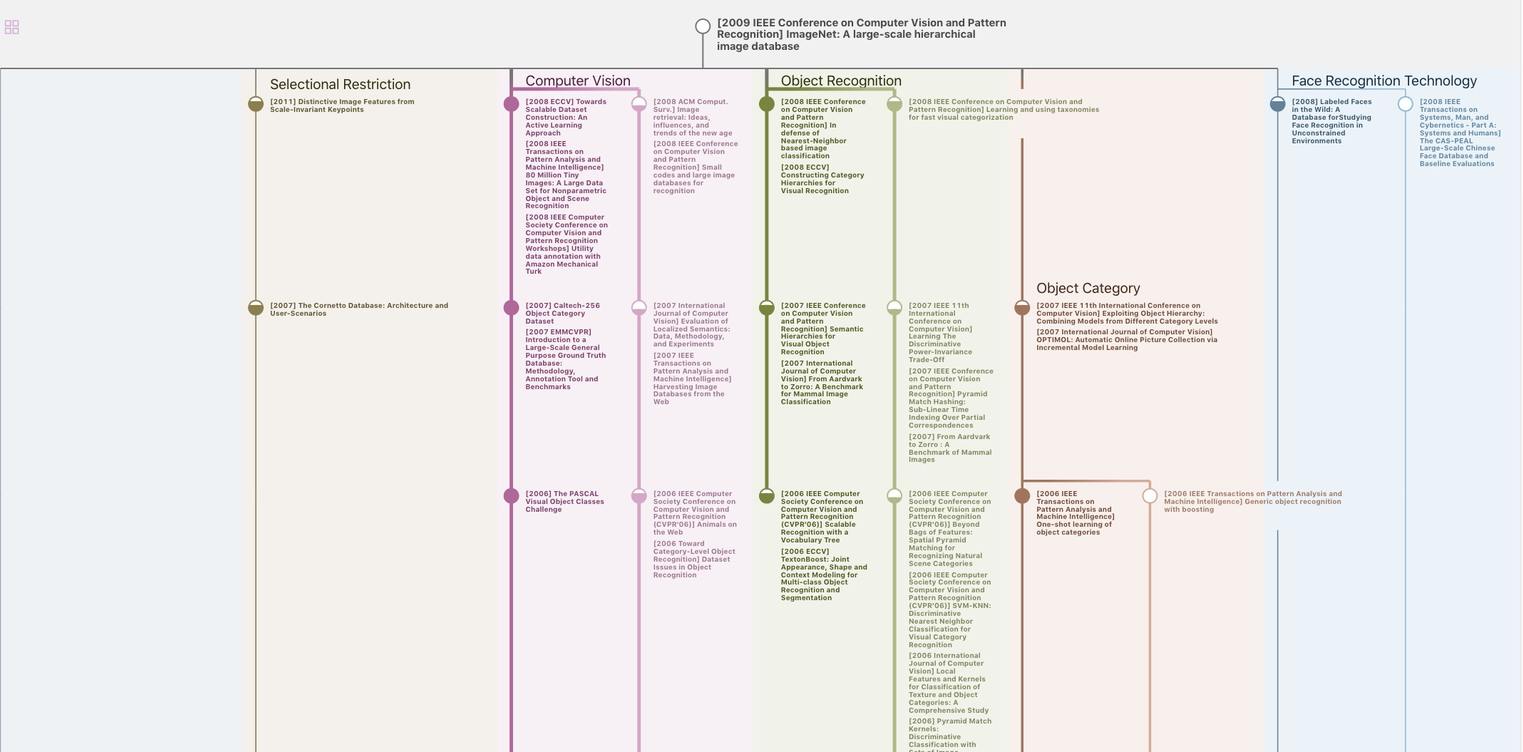
Generate MRT to find the research sequence of this paper
Chat Paper
Summary is being generated by the instructions you defined