Comparative Analysis of Similarity Methods in High-Dimensional Vectors: A Review
2023 International Conference on Artificial Intelligence Science and Applications in Industry and Society (CAISAIS)(2023)
摘要
With the increasing availability of high-dimensional data in diverse fields as well as the need to process this data, the accurate measurement of similarity plays a crucial role in many applications. Specifically, the machine learning models that check for similarity between given items need methods that are both time efficient and accurate. The exploration of high-dimensional data has become increasingly crucial in diverse fields, including natural language processing, computer vision, bioinformatics, and more. This paper presents a comprehensive comparison of various similarity methods in high-dimensional vectors. This paper is concerned with evaluating and analyzing a number of different similarity methods, considering their effectiveness, efficiency, and robustness in capturing similarities within high-dimensional datasets. The methods shown were tested on a total of 11 open source benchmark data sets The experimental results on various datasets demonstrate the strengths and limitations of each method, providing valuable insights for researchers and practitioners in selecting appropriate similarity measures for their specific needs.
更多查看译文
关键词
HD computing,Machine Learning,Similarity methods
AI 理解论文
溯源树
样例
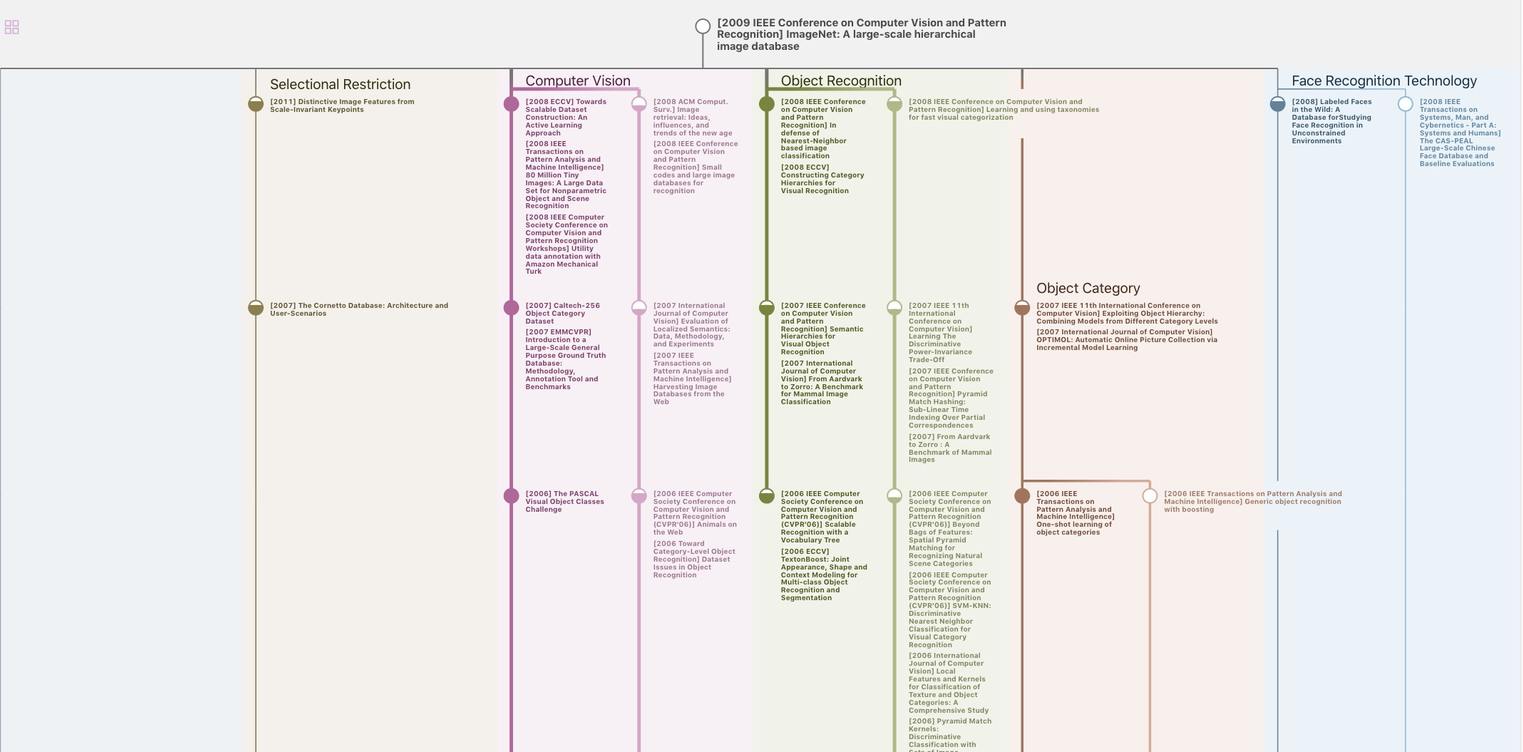
生成溯源树,研究论文发展脉络
Chat Paper
正在生成论文摘要