A Comprehensive Performance Study of Large Language Models on Novel AI Accelerators
CoRR(2023)
摘要
Artificial intelligence (AI) methods have become critical in scientific applications to help accelerate scientific discovery. Large language models (LLMs) are being considered as a promising approach to address some of the challenging problems because of their superior generalization capabilities across domains. The effectiveness of the models and the accuracy of the applications is contingent upon their efficient execution on the underlying hardware infrastructure. Specialized AI accelerator hardware systems have recently become available for accelerating AI applications. However, the comparative performance of these AI accelerators on large language models has not been previously studied. In this paper, we systematically study LLMs on multiple AI accelerators and GPUs and evaluate their performance characteristics for these models. We evaluate these systems with (i) a micro-benchmark using a core transformer block, (ii) a GPT- 2 model, and (iii) an LLM-driven science use case, GenSLM. We present our findings and analyses of the models' performance to better understand the intrinsic capabilities of AI accelerators. Furthermore, our analysis takes into account key factors such as sequence lengths, scaling behavior, sparsity, and sensitivity to gradient accumulation steps.
更多查看译文
关键词
large language models,novel ai
AI 理解论文
溯源树
样例
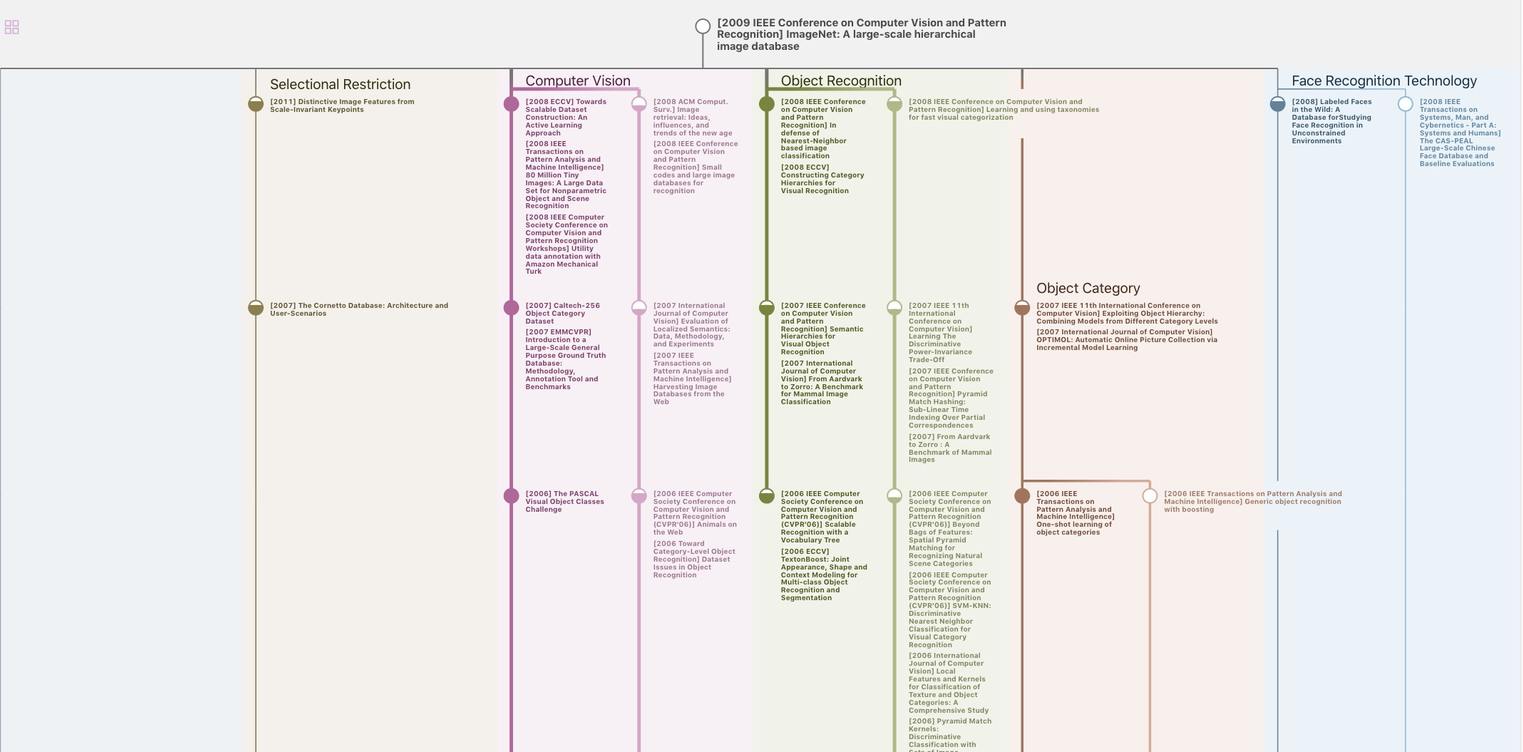
生成溯源树,研究论文发展脉络
Chat Paper
正在生成论文摘要