Generalized Neural Collapse for a Large Number of Classes
CoRR(2023)
摘要
Neural collapse provides an elegant mathematical characterization of learned last layer representations (a.k.a. features) and classifier weights in deep classification models. Such results not only provide insights but also motivate new techniques for improving practical deep models. However, most of the existing empirical and theoretical studies in neural collapse focus on the case that the number of classes is small relative to the dimension of the feature space. This paper extends neural collapse to cases where the number of classes are much larger than the dimension of feature space, which broadly occur for language models, retrieval systems, and face recognition applications. We show that the features and classifier exhibit a generalized neural collapse phenomenon, where the minimum one-vs-rest margins is maximized.We provide empirical study to verify the occurrence of generalized neural collapse in practical deep neural networks. Moreover, we provide theoretical study to show that the generalized neural collapse provably occurs under unconstrained feature model with spherical constraint, under certain technical conditions on feature dimension and number of classes.
更多查看译文
关键词
classes
AI 理解论文
溯源树
样例
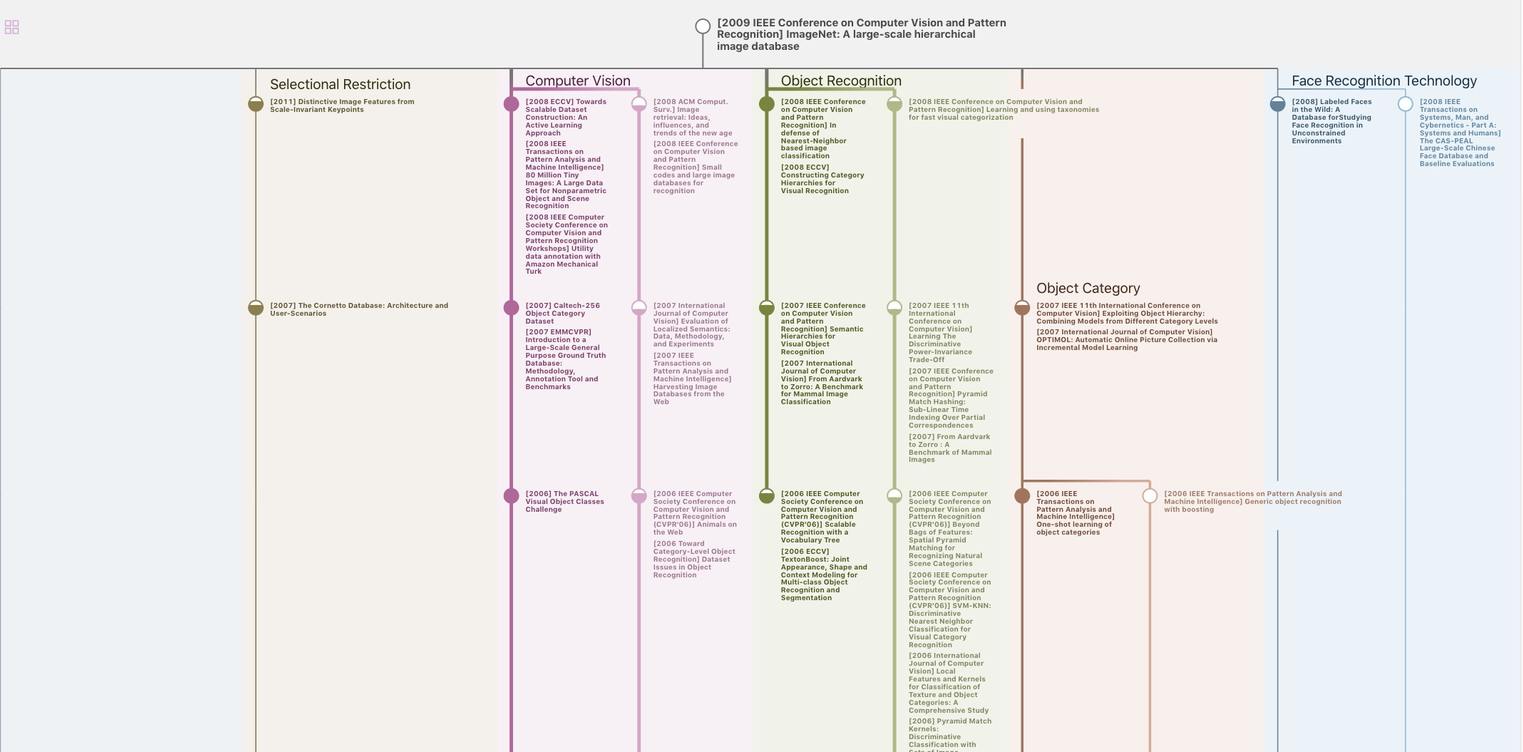
生成溯源树,研究论文发展脉络
Chat Paper
正在生成论文摘要