Classification of Bearing Faults in Induction Motors with the Hilbert-Huang Transform and Feature Selection
2023 IEEE 14th International Symposium on Diagnostics for Electrical Machines, Power Electronics and Drives (SDEMPED)(2023)
摘要
This study proposes a novel approach to detect bearing damage in induction motors during operation. Vibration signals from the Case Western Reserve University dataset of bearings are used to validate this proposal. The data are preprocessed using the Hilbert-Huang transform, and 10 attributes are extracted from both the time and time-frequency domains. Synthetic minority oversampling technique (SMOTE) is applied to remove imbalances in the dataset, the random forest (RF) as a feature selection extractor and the variance inflation factor (VIF) are used to select the proper features for good performance in fault identification. Grid search is employed to find the optimal parameters of five well-known supervised machine learning algorithms to classify two scenarios: i) health and fault bearing, and ii) health and types of bearing faults. The results show that k-nearest neighbor (k-NN) and RF are the most suitable classifiers, with accuracies of 99.7% and 89.34%, respectively.
更多查看译文
关键词
Bearing faults,machine learning,feature selection
AI 理解论文
溯源树
样例
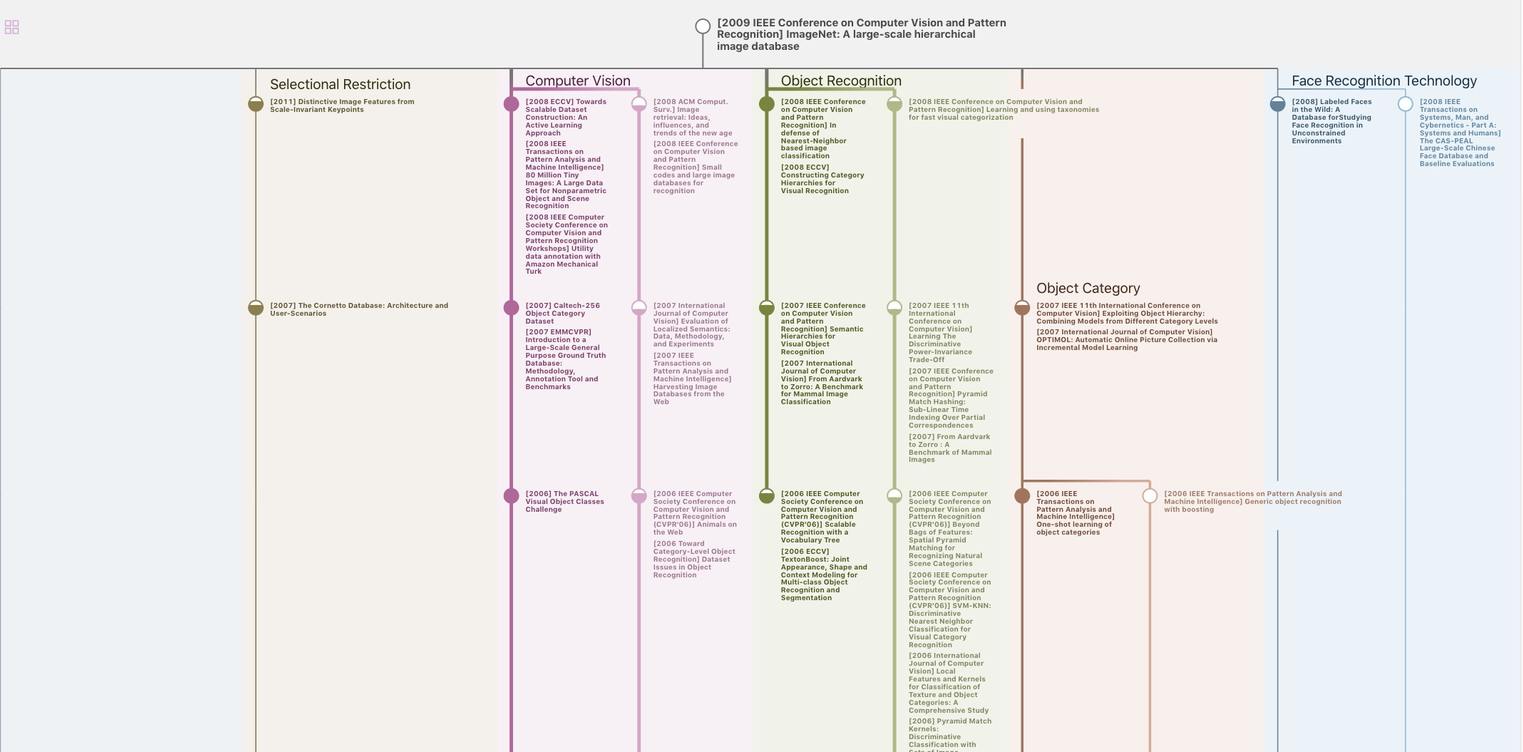
生成溯源树,研究论文发展脉络
Chat Paper
正在生成论文摘要