Global Convergence of Policy Gradient Methods in Reinforcement Learning, Games and Control
CoRR(2023)
摘要
Policy gradient methods, where one searches for the policy of interest by maximizing the value functions using first-order information, become increasingly popular for sequential decision making in reinforcement learning, games, and control. Guaranteeing the global optimality of policy gradient methods, however, is highly nontrivial due to nonconcavity of the value functions. In this exposition, we highlight recent progresses in understanding and developing policy gradient methods with global convergence guarantees, putting an emphasis on their finite-time convergence rates with regard to salient problem parameters.
更多查看译文
关键词
policy gradient methods,reinforcement learning
AI 理解论文
溯源树
样例
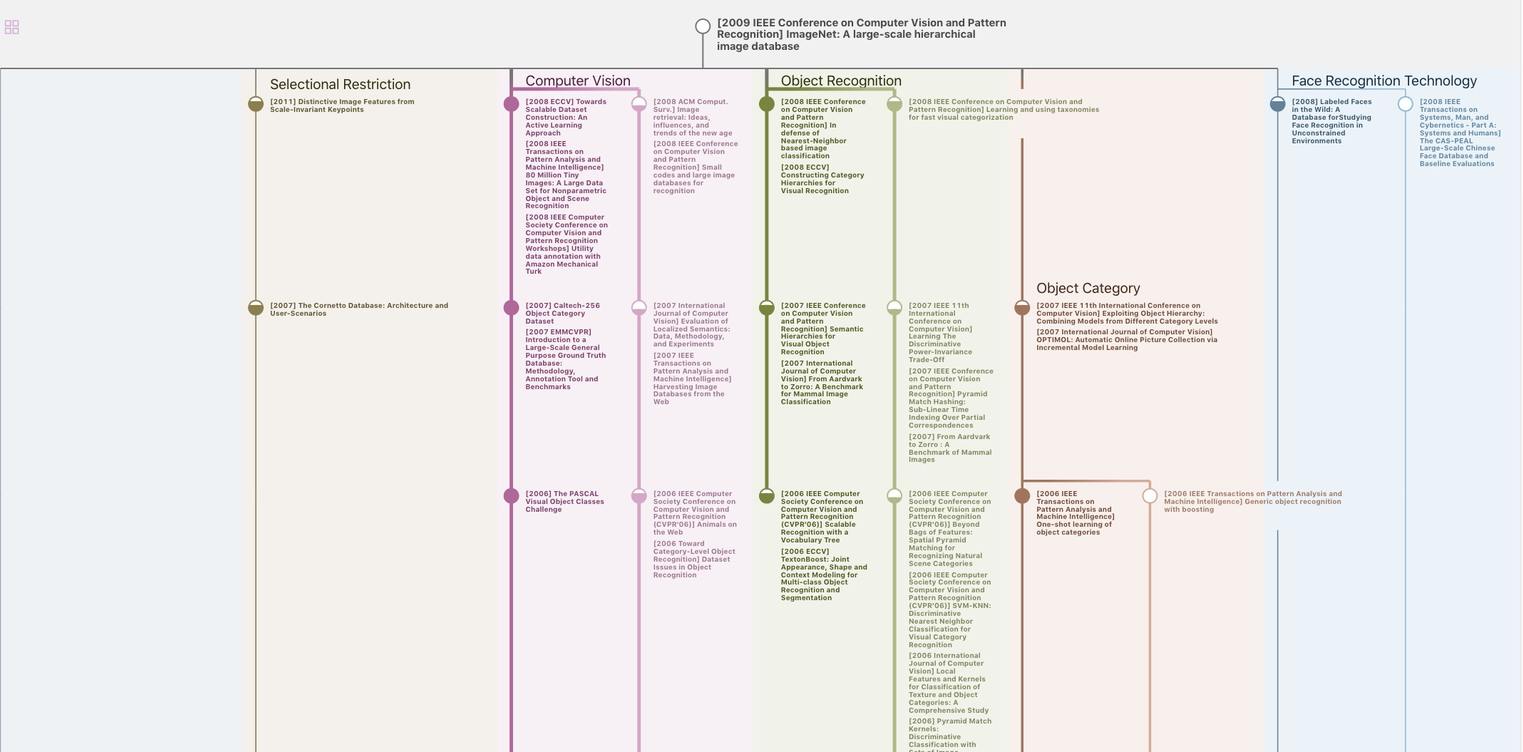
生成溯源树,研究论文发展脉络
Chat Paper
正在生成论文摘要