Multi-Level Evidence Active Learning for Water Surface Object Detection
2023 8th International Conference on Image, Vision and Computing (ICIVC)(2023)
Abstract
Object detection is a rapidly evolving field, but there remains a challenge that the requirement of a significant amount of labeled data. In the recent five years, several active learning strategies have been proposed to achieve improved performance with reduced annotation cost on object detection tasks. However, these approaches have some limitations, including the incomplete utilization of epistemic uncertainty and bounding box information. To address these limitations, this paper proposes a novel active learning method named Multi-Level Evidence Active Learning (MLEAL) for water surface object detection. We propose to utilize Evidence Deep Learning (EDL) for uncertainty estimation and designed an Evidence Optimization Head (EOH) to adapt EDL for water surface object detection. To compute the information of an image by considering the multi-level information of the bounding box, we introduce to the utilization of Multi-Level Uncertainty Aggregation (MLUA) to represent the information over an image. The proposed MLEAL is valid on the Water Surface Object Detection Dataset (WSODD). The results demonstrate that the MLEAL outperforms existing active learning methods.
MoreTranslated text
Key words
active learning,evidence deep learning,multi-level aggregation,surface object detection
AI Read Science
Must-Reading Tree
Example
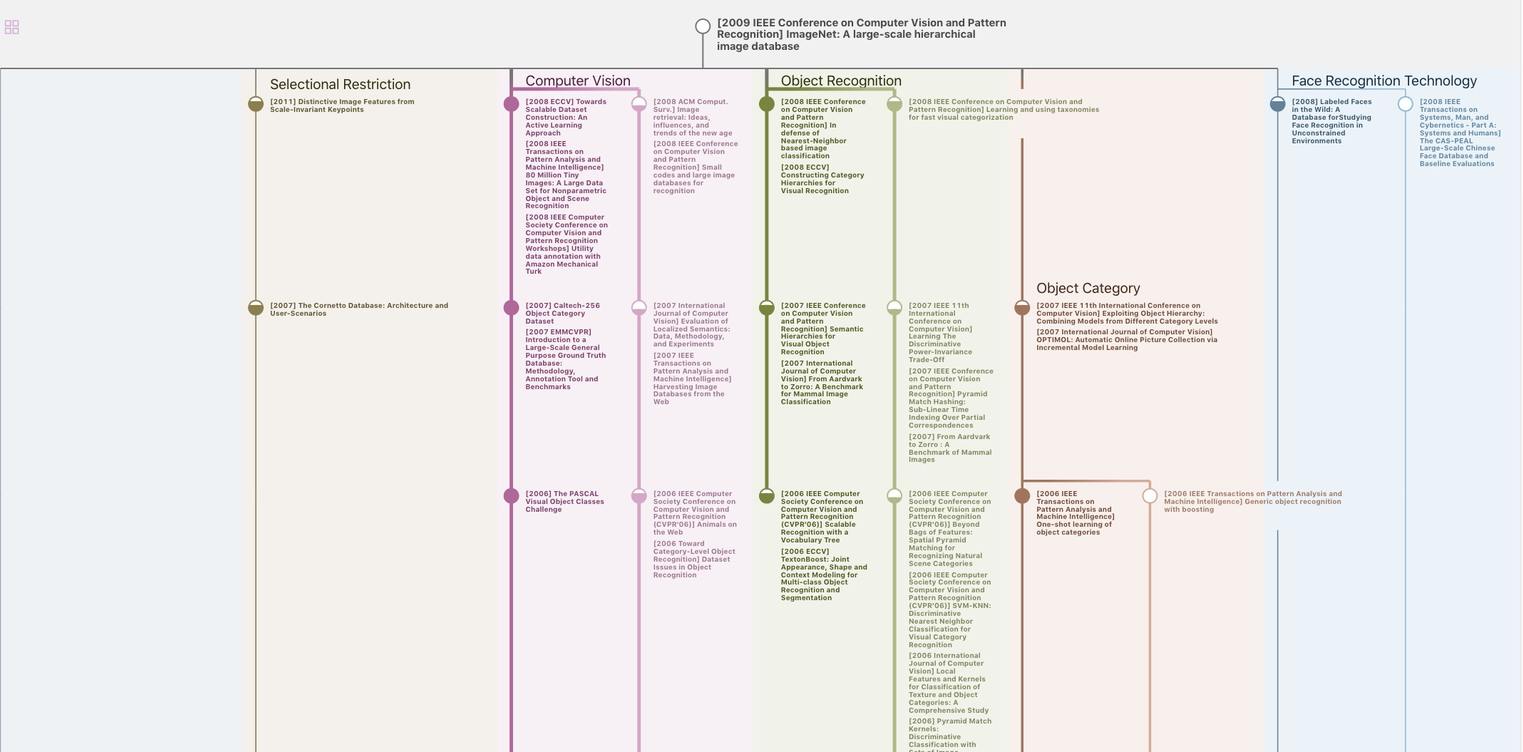
Generate MRT to find the research sequence of this paper
Chat Paper
Summary is being generated by the instructions you defined