${\rm H{\scriptsize ALO}F{\scriptsize LOW}}$ I: Neural Inference of Halo Mass from Galaxy Photometry and Morphology
arXiv (Cornell University)(2023)
摘要
We present ${\rm H{\scriptsize ALO}F{\scriptsize LOW}}$, a new machine learning approach for inferring the mass of host dark matter halos, $M_h$, from the photometry and morphology of galaxies. ${\rm H{\scriptsize ALO}F{\scriptsize LOW}}$ uses simulation-based inference with normalizing flows to conduct rigorous Bayesian inference. It is trained on state-of-the-art synthetic galaxy images from Bottrell et al. (2023; arXiv:2308.14793) that are constructed from the IllustrisTNG hydrodynamic simulation and include realistic effects of the Hyper Suprime-Cam Subaru Strategy Program (HSC-SSP) observations. We design ${\rm H{\scriptsize ALO}F{\scriptsize LOW}}$ to infer $M_h$ and stellar mass, $M_*$, using $grizy$ band magnitudes, morphological properties quantifying characteristic size, concentration, and asymmetry, total measured satellite luminosity, and number of satellites. We demonstrate that ${\rm H{\scriptsize ALO}F{\scriptsize LOW}}$ infers accurate and unbiased posteriors of $M_h$. Furthermore, we quantify the full information content in the photometric observations of galaxies in constraining $M_h$. With magnitudes alone, we infer $M_h$ with $\sigma_{\log M_h} \sim 0.115$ and 0.182 dex for field and group galaxies. Including morphological properties significantly improves the precision of $M_h$ constraints, as does total satellite luminosity: $\sigma_{\log M_h} \sim 0.095$ and 0.132 dex. Compared to the standard approach using the stellar-to-halo mass relation, we improve $M_h$ constraints by $\sim$40\%. In subsequent papers, we will validate and calibrate ${\rm H{\scriptsize ALO}F{\scriptsize LOW}}$ with galaxy-galaxy lensing measurements on real observational data.
更多查看译文
关键词
galaxy photometry,halo
AI 理解论文
溯源树
样例
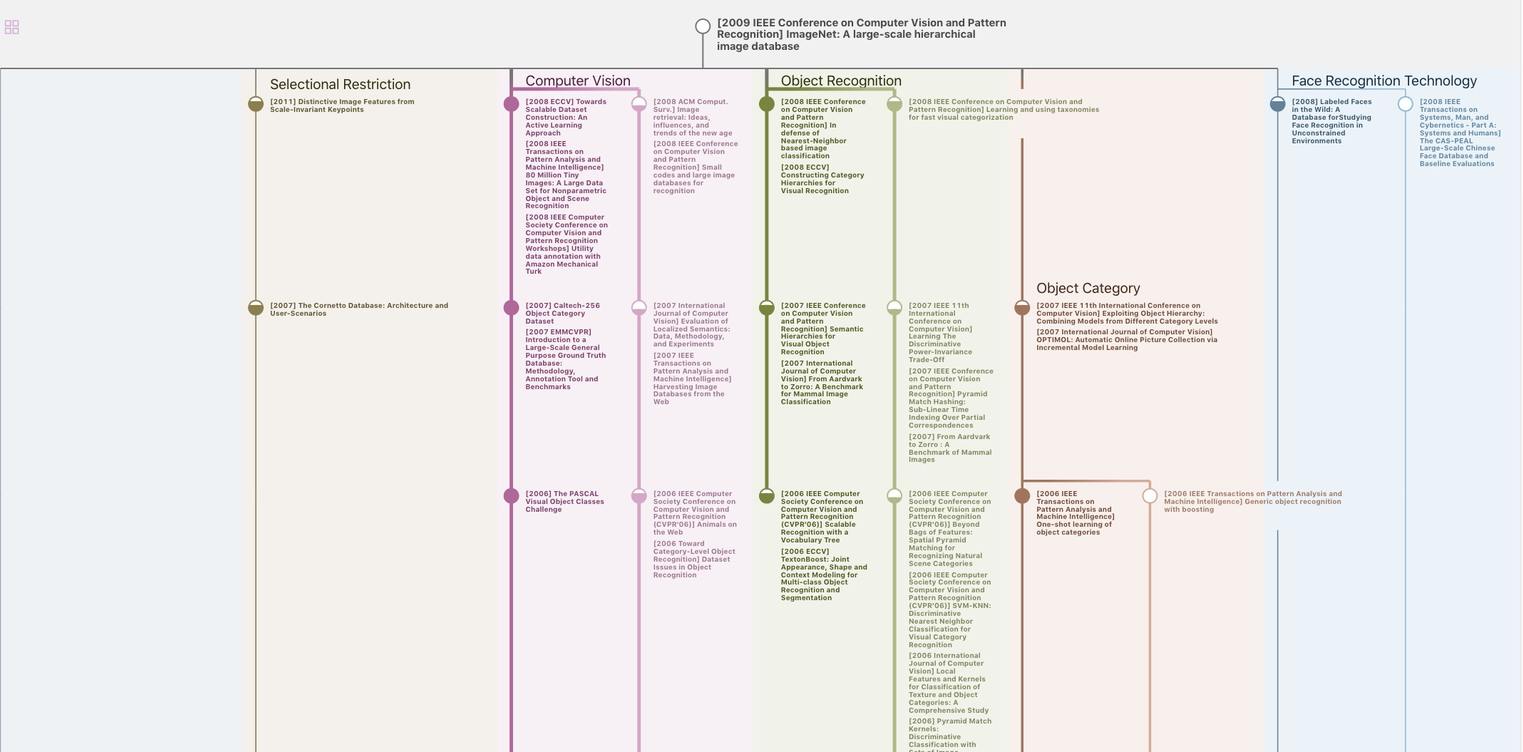
生成溯源树,研究论文发展脉络
Chat Paper
正在生成论文摘要