Position‐aware spatio‐temporal graph convolutional networks for skeleton‐based action recognition
IET Computer Vision(2023)
摘要
Abstract Graph Convolutional Networks (GCNs) have been widely used in skeleton‐based action recognition. Though significant performance has been achieved, it is still challenging to effectively model the complex dynamics of skeleton sequences. A novel position‐aware spatio‐temporal GCN for skeleton‐based action recognition is proposed, where the positional encoding is investigated to enhance the capacity of typical baselines for comprehending the dynamic characteristics of action sequence. Specifically, the authors’ method systematically investigates the temporal position encoding and spatial position embedding, in favour of explicitly capturing the sequence ordering information and the identity information of nodes that are used in graphs. Additionally, to alleviate the redundancy and over‐smoothing problems of typical GCNs, the authors’ method further investigates a subgraph mask, which gears to mine the prominent subgraph patterns over the underlying graph, letting the model be robust against the impaction of some irrelevant joints. Extensive experiments on three large‐scale datasets demonstrate that our model can achieve competitive results comparing to the previous state‐of‐art methods.
更多查看译文
关键词
computer vision,convolutional neural nets,graph theory
AI 理解论文
溯源树
样例
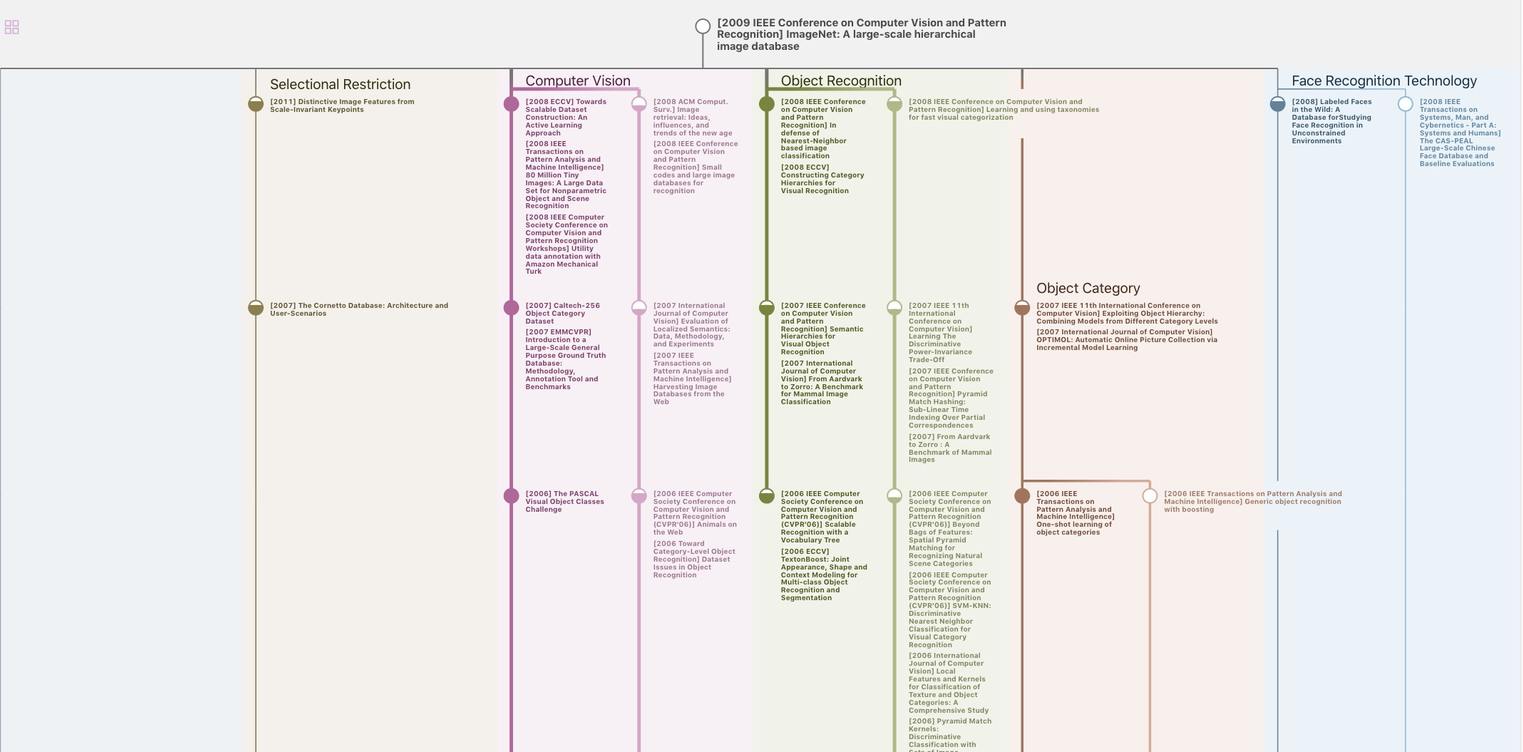
生成溯源树,研究论文发展脉络
Chat Paper
正在生成论文摘要