Baldur: Bayesian Hierarchical Modeling for Label-Free Proteomics with Gamma Regressing Mean-Variance Trends
MOLECULAR & CELLULAR PROTEOMICS(2023)
摘要
Label -free proteomics is a fast-growing methodology to infer abundances in mass spectrometry proteomics. Extensive research has focused on spectral quantification and peptide identification. However, research toward modeling and understanding quantitative proteomics data is scarce. Here we propose a Bayesian hierarchical decision model (Baldur) to test for differences in means between conditions for proteins, peptides, and posttranslational modifications. We developed a Bayesian regression model to characterize local mean -variance trends in data, to estimate measurement uncertainty and hyperparameters for the decision model. A key contribution is the development of a new gamma regression model that describes the mean -variance dependency as a mixture of a common and a latent trend-allowing for localized trend estimates. We then evaluate the performance of Baldur, limma-trend, and t test on six benchmark datasets: five total proteomics and one posttranslational modification dataset. We find that Baldur drastically improves the decision in noisier post -translational modification data over limma-trend and t test. In addition, we see significant improvements using Baldur over the other methods in the total proteomics datasets. Finally, we analyzed Baldur's performance when increasing the number of replicates and found that the method always increases precision with sample size, while showing robust control of the false positive rate. We conclude that our model vastly improves over popular data analysis methods (limma-trend and t test) in several spike -in datasets by achieving a high true positive detection rate, while greatly reducing the false -positive rate.
更多查看译文
关键词
proteomics,Bayesian hierarchical model,empirical Bayes,mean-variance trends,latent gamma mixture regression,uncertainty modeling,label-free quantification,data-independent acquisition
AI 理解论文
溯源树
样例
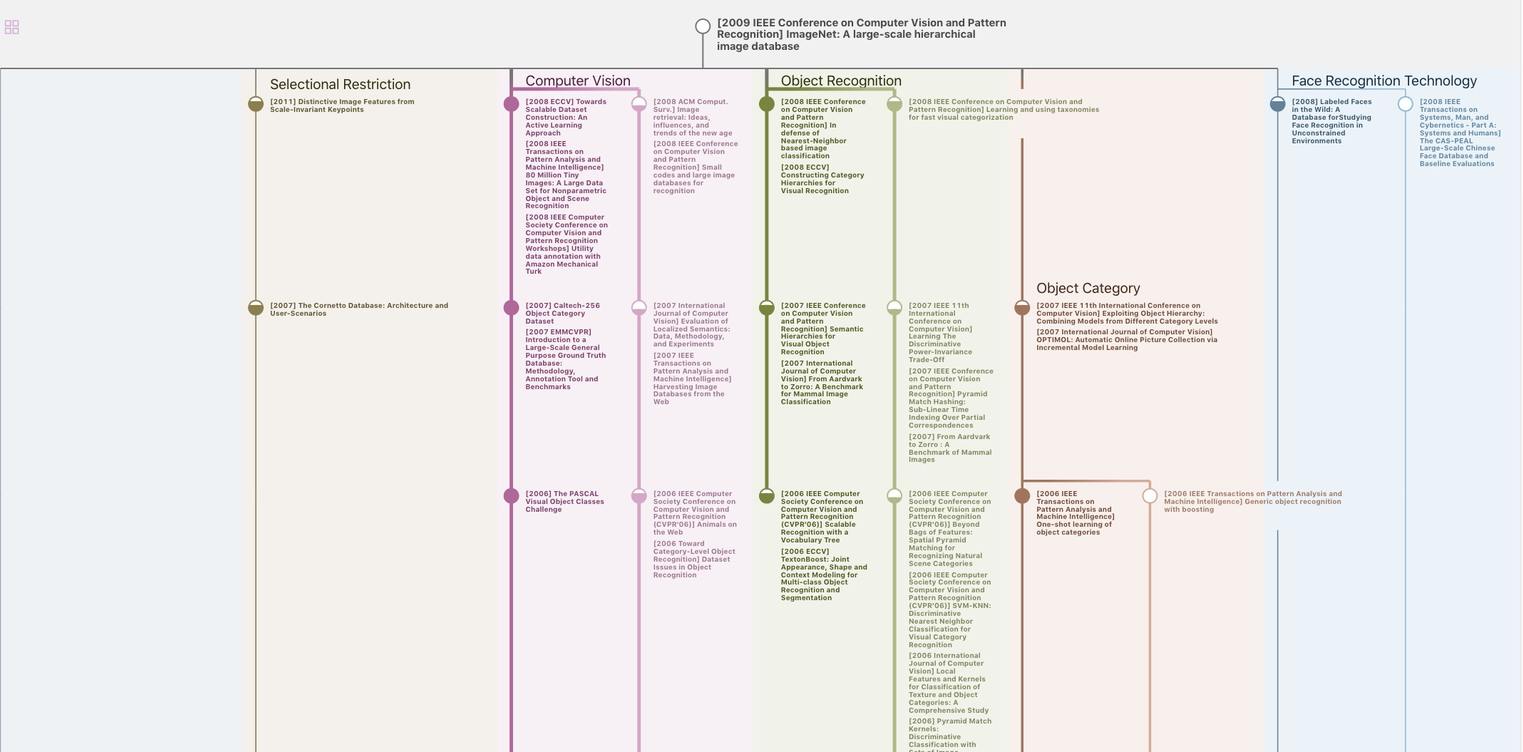
生成溯源树,研究论文发展脉络
Chat Paper
正在生成论文摘要