A multi-stage recognizer for nested named entity with weakly labeled data
JOURNAL OF SUPERCOMPUTING(2024)
摘要
Existing weakly supervised named entity recognition (NER) research only deals with flat entities and ignores nested entities. This paper proposes a multi-stage nested entity recognition method (MNR) that utilizes weakly labeled data to recognize nested entities. However, weak labels generated through external knowledge bases have two problems: incompleteness and labeling bias. To address this challenge, the MNR comprises two models. First, we propose a neural transition-based attention model (NTAM) to solve the problem of weak-label incompleteness by learning the correlation between words. Simultaneously, the NTAM obtains candidate entities, including nested entities. Second, we propose a multi-marker fusion attention judgment model (MAJM) for selecting candidate entities through context semantics, candidate entities' meanings, and their boundary information, thereby solving the labeling bias problem. The boundary information of candidate entities is enhanced by fusing their type markers. To our knowledge, we are the first to recognize nested entities under weak supervision by alleviating the noise of weakly labeled data. Experiments on three public nested NER datasets prove the effectiveness of our proposed method under weak supervision and demonstrate that the method outperforms previous state-of-the-art models under supervision.
更多查看译文
关键词
Nested named entity recognition,Weakly supervised NER,Weakly labeled data,Neural transition-based model,Multi-marker fusion
AI 理解论文
溯源树
样例
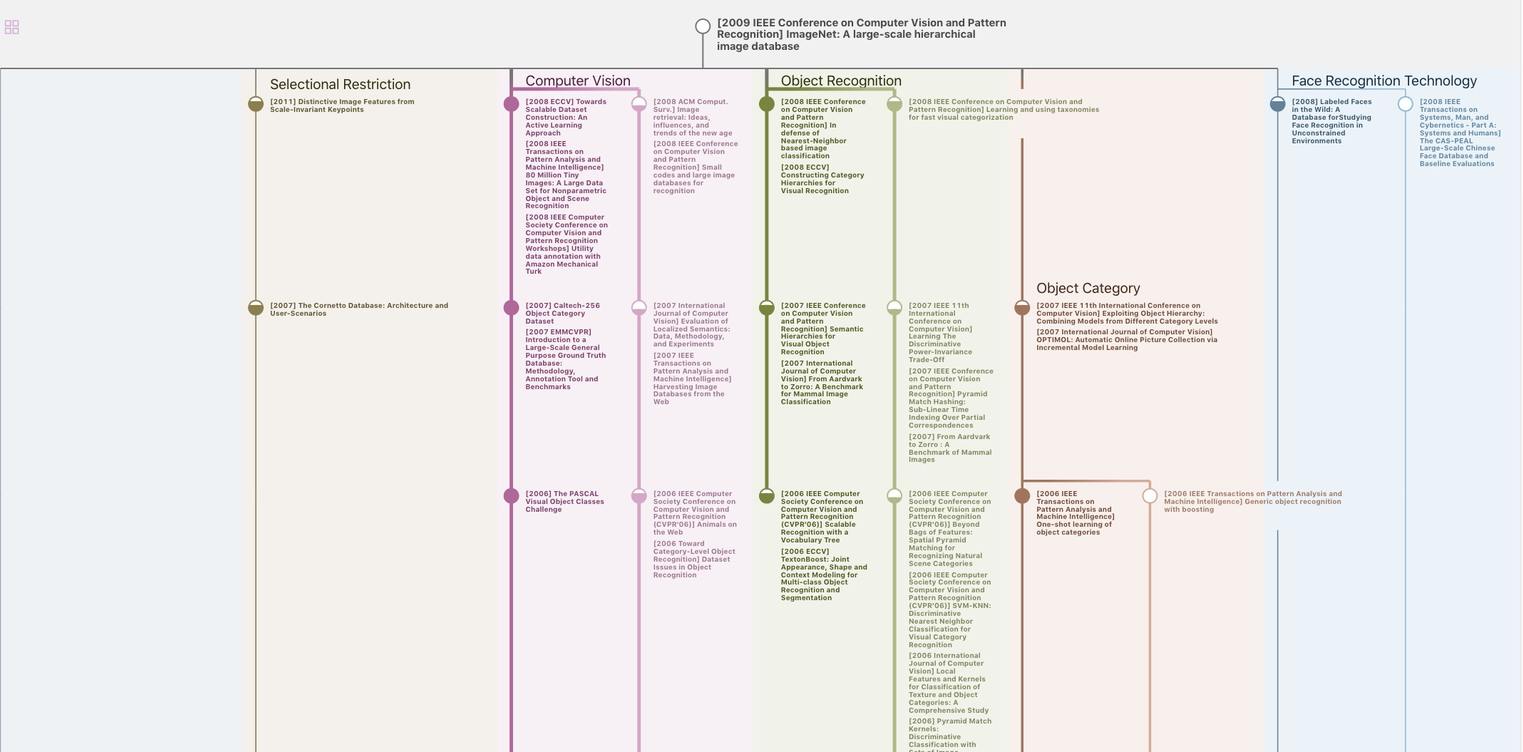
生成溯源树,研究论文发展脉络
Chat Paper
正在生成论文摘要