A physics-informed CNN-TSE hybrid network for micro-EDM process monitoring and control
MECHANICAL SYSTEMS AND SIGNAL PROCESSING(2023)
Abstract
Despite being a widespread non-conventional processing technique, electrical discharge ma-chining (EDM) has been complicated due to its removal mechanisms and weak process stability for decades. Process monitoring is an enabling technology to offer an indirect insight into the discharge phenomena, understanding the probabilistic anomaly events such as arcs and short circuits. However, the transient high-frequency pulse train poses a great challenge for statistical monitoring and control. Traditional approaches are limited to single pulse analysis, which hinders a deep understanding of the process. To address the challenge, this paper proposes a novel physics-informed deep learning architecture for real-time micro-EDM process monitoring. To prepare condition labels, a series of signal-processing techniques including sensory fusion, pulse-shape filtering and pulse segmentation are employed, followed by observation diagrams on two critical indicators, i.e. discharge energy and discharge time interval. A decision logic that allows embedding of domain knowledge is formulated, yielding physics-informed labels on each pulse train. For online monitoring applications, a hybrid hierarchical structure that combines a 1D convolutional neural network (1D-CNN) and a Transformer encoder (TSE) is designed to simultaneously extract local pulse features and temporal dependencies between pulse sequences. This deep learning model achieves a classification accuracy of 96% on the validation dataset and reaches precision and recall scores of more than 92% on the test dataset with varying processing parameters. Two model interpretation approaches, i.e. gradient-weighted class activation mapping (Grad-CAM) and attention visualization, are adopted. Consequently, it is found that the CNN module assigns high feature importance to the breakdown and maintaining stages of one pulse and the TSE module improves the feature salience and reliance on the condition of distinct events. This monitoring network is further demonstrated on a control application for adaptive regulation of the micro-EDM drilling process. The results of deep-hole drilling suggest that the CNN-TSE model can highly improve the process stability and hence the machining quality.
MoreTranslated text
Key words
Process monitoring,Process control,Signal processing,Deep learning,Self-attention,Transformer,CNN,Time series,EDM
AI Read Science
Must-Reading Tree
Example
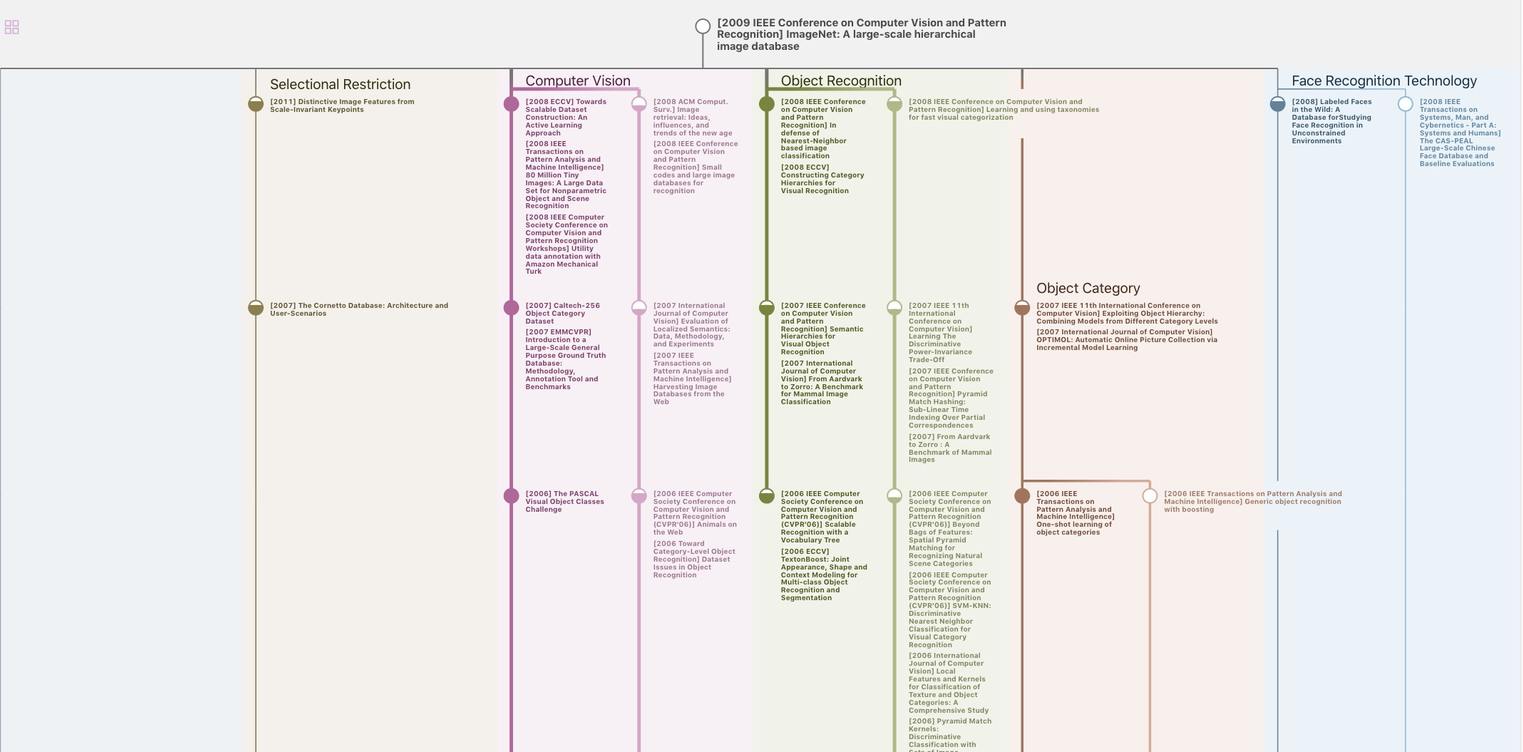
Generate MRT to find the research sequence of this paper
Chat Paper
Summary is being generated by the instructions you defined