Gaussian copula function-on-scalar regression in reproducing kernel Hilbert space
JOURNAL OF MULTIVARIATE ANALYSIS(2023)
摘要
To relax the linear assumption in function-on-scalar regression, we borrow the strength of copula and propose a novel Gaussian copula function-on-scalar regression. Our model is more flexible to characterize the dynamic relationship between functional response and scalar predictors. Estimation, prediction, and inference are fully investigated. We develop a closed form for the estimator of coefficient functions in a reproducing kernel Hilbert space without the knowledge of marginal transformations. Valid, distribution-free, finite-sample prediction bands are constructed via conformal prediction. Theoretically, we establish the optimal convergence rate on the estimation of coefficient functions and show that our proposed estimator is rate-optimal under fixed and random designs. The finite-sample performance is investigated through simulations and illustrated in real data analysis.& COPY; 2023 Elsevier Inc. All rights reserved.
更多查看译文
关键词
Copula model,Function-on-scalar regression,Image analysis,Optimal rate of convergence,Statistical inference
AI 理解论文
溯源树
样例
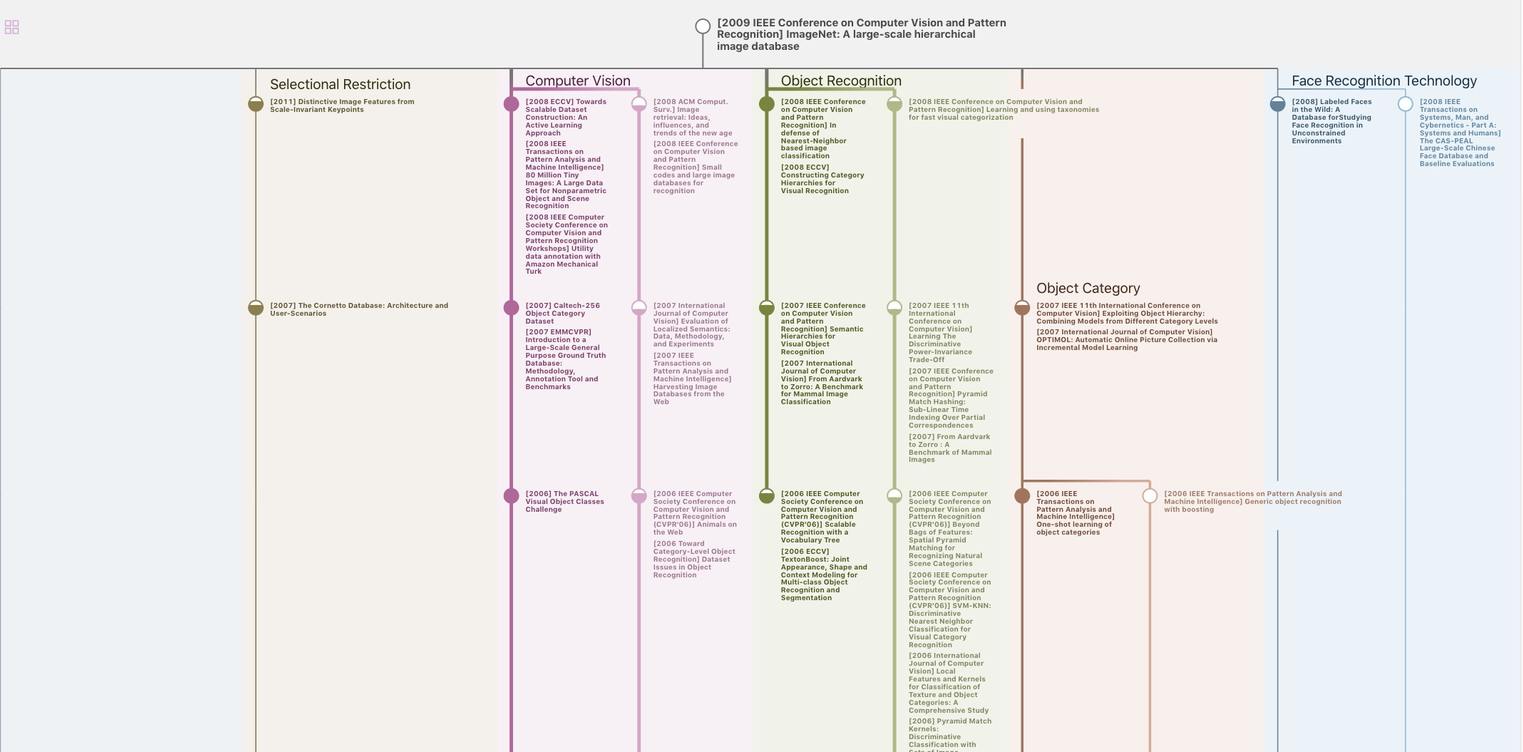
生成溯源树,研究论文发展脉络
Chat Paper
正在生成论文摘要