Graph neural network architecture search for rotating machinery fault diagnosis based on reinforcement learning
MECHANICAL SYSTEMS AND SIGNAL PROCESSING(2023)
Abstract
In order to improve the accuracy of fault diagnosis, researchers are constantly trying to develop new diagnostic models. However, limited by the inherent thinking of human beings, it has always been difficult to build a pioneering architecture for rotating machinery fault diagnosis. In order to solve this problem, this paper uses reinforcement learning algorithm based on adjacency matrix to carry out network architecture search (NAS) of rotating machinery fault diagnosis model. A reinforcement learning agent for deep deterministic policy gradient (DDPG) is developed based on actor–critic neural networks. The observation state of reinforcement learning is used to develop the graph neural network (GNN) diagnosis model, and the diagnosis accuracy is fed back to the agent as a reward for updating the reinforcement learning parameters. The MFPT bearing fault datasets and the developed gear pitting fault experimental data are used to validate the proposed network architecture search method based on reinforcement learning (RL-NAS). The proposed method is proved to be practical and effective in various aspects such as fault diagnosis ability, search space, search efficiency and multi-working condition performance.
MoreTranslated text
Key words
Rotating machinery,Fault diagnosis,Graph neural network,Neural architecture search,Reinforcement learning
AI Read Science
Must-Reading Tree
Example
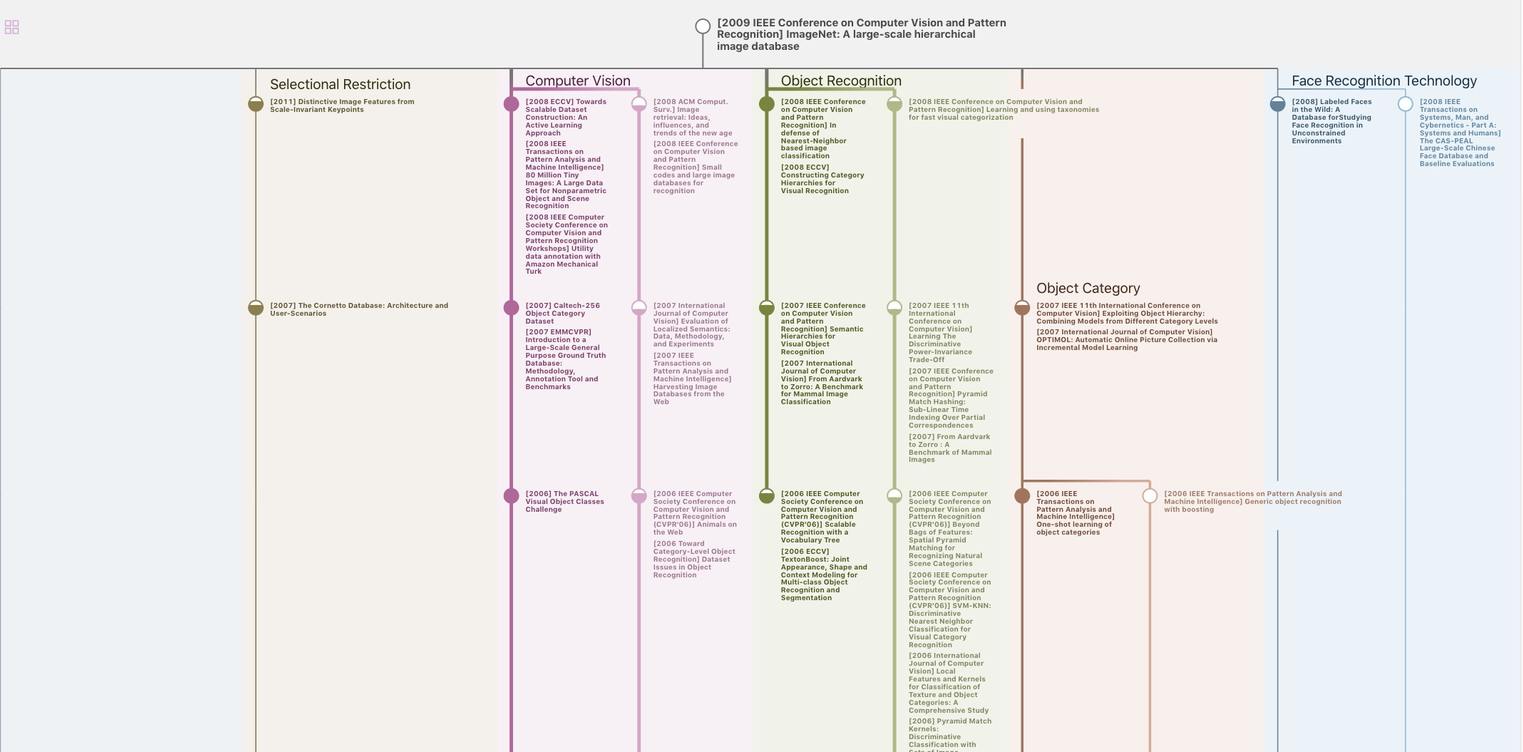
Generate MRT to find the research sequence of this paper
Chat Paper
Summary is being generated by the instructions you defined