Smart manufacturing under limited and heterogeneous data: a sim-to-real transfer learning with convolutional variational autoencoder in thermoforming
INTERNATIONAL JOURNAL OF COMPUTER INTEGRATED MANUFACTURING(2024)
摘要
Data in advanced manufacturing are often sparse and collected from various sensory devices in a heterogeneous and multi-modal fashion. Thus, for such intricate input spaces, learning robust and reliable predictive models for product quality assessments entails implementing complex nonlinear models such as deep learning. However, these 'data-greedy' models require massive datasets for training, and they tend to exhibit poor generalization performance otherwise. To address the data paucity and the data heterogeneity in smart manufacturing applications, this paper introduces a sim-to-real transfer-learning framework. Specifically, using a unified wide-and-deep learning approach, the model pre-processes structured sensory data (wide) as well as high-dimensional thermal images (deep) separately, and then passes the respective concatenated features to a regressor for predicting product quality metrics. Convolutional variational autoencoder (ConvVAE) is utilized to learn concise representations of thermal images in an unsupervised fashion. ConvVAE is trained via a sim-to-real transfer learning approach, backed by theory-based heat transfer simulations. The proposed metamodeling framework was evaluated in an industrial thermoforming process case study. The results suggested that ConvVAE outperforms conventional dimensionality reduction methods despite limited data. A model explainability analysis was conducted and the resulting SHAP values demonstrated the agreement between the model's predictions, theoretical expectations, and data correlation statistics.
更多查看译文
关键词
Intelligent manufacturing,transfer learning,convolutional variational autoencoder,thermoforming,model explainability
AI 理解论文
溯源树
样例
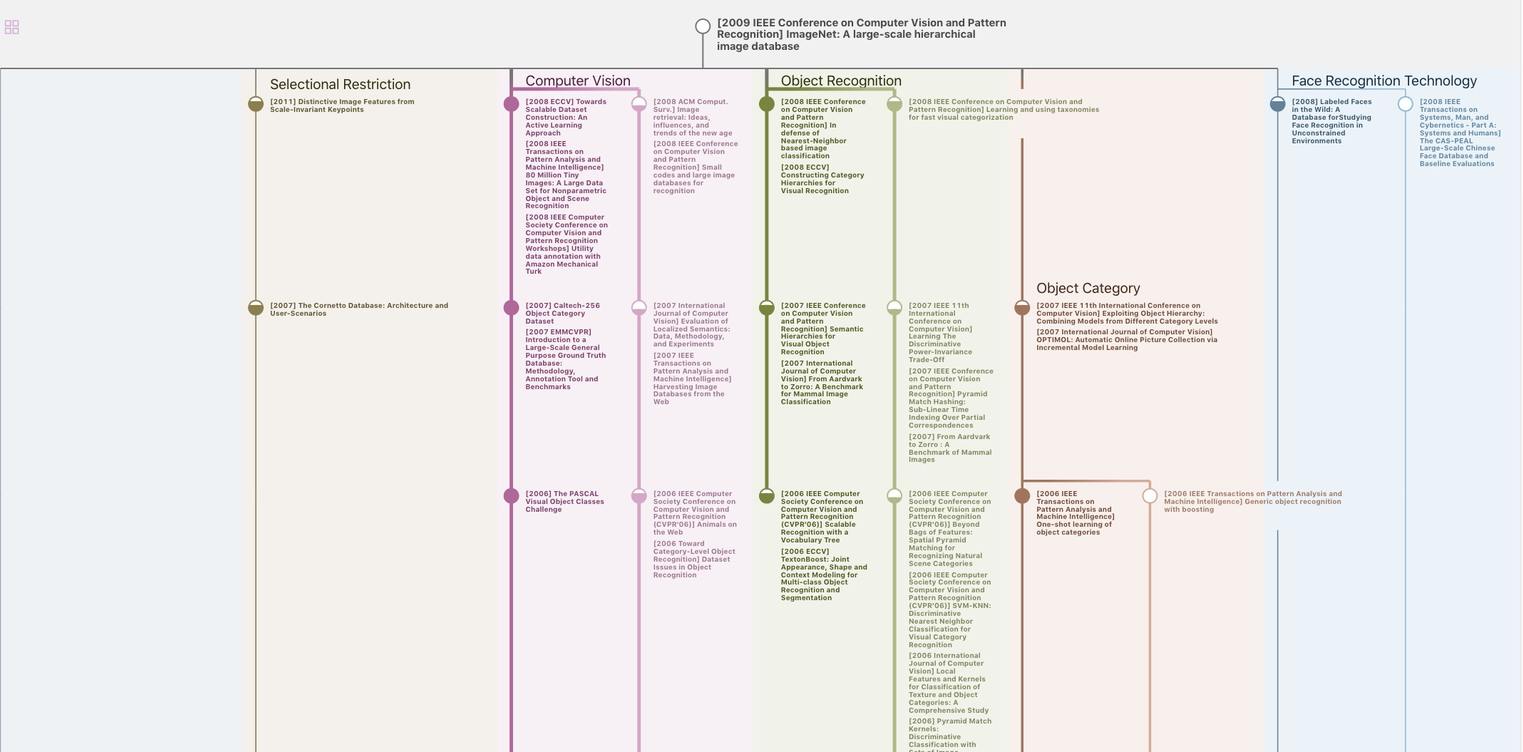
生成溯源树,研究论文发展脉络
Chat Paper
正在生成论文摘要