Control policy transfer of deep reinforcement learning based intelligent forced heat convection control
INTERNATIONAL JOURNAL OF THERMAL SCIENCES(2024)
摘要
Deep reinforcement learning (DRL) has gradually emerged as a novel and effective method for intelligent control of conjugate heat transfer. Through proper training, DRL agent usually can find a better control strategy than the one optimized manually. For numerous numerical simulation-based investigations, the promising results have only been obtained on 2-dimensional models due to the heavy burden of data acquisition. This paper proposes a novel strategy of transferring the control policy learned in 2-dimensional environment into a new 3-dimensional environment. PDD-DQN (Prioritized Dueling Double Deep Q-Networks) algorithm is used to recognize the underlying relationship between the forced fluid flow and heat transfer performance to produce a control strategy. The DRL controller is first trained on a simple 2-dimensional cavity with one heat source, and the DRL controller is able to reduce the maximum temperature to 315 K which is 2 K lower than the manually optimized control strategy; then the control strategy is transferred to 3-dimensional models where the maximum temperature is further reduced to 308 K; and compared to training the DRL agent directly on 3-dimensional model, the policytransfer strategy requires only 10% computational expenditure. The proposed strategy is then applied to a more complex testbed with multiple heat sources for further investigating its ability; in both 2D and 3D environment, the DRL controller can cool the maximum temperature to be 307 K which is 8 K lower than the manually optimized control policy; and the computational cost drop to be 3%. The generalization ability of the trained DRL agent in inter-dimensional geometries is confirmed. For the cases with huge grid size, the policy-transfer strategy can economize exponential computational cost, which is of great significance for applying DRL methods to practical thermal control problems.
更多查看译文
关键词
Deep reinforcement learning,Active thermal control,Forced heat convection,Heat transfer enhancement,Machine learning
AI 理解论文
溯源树
样例
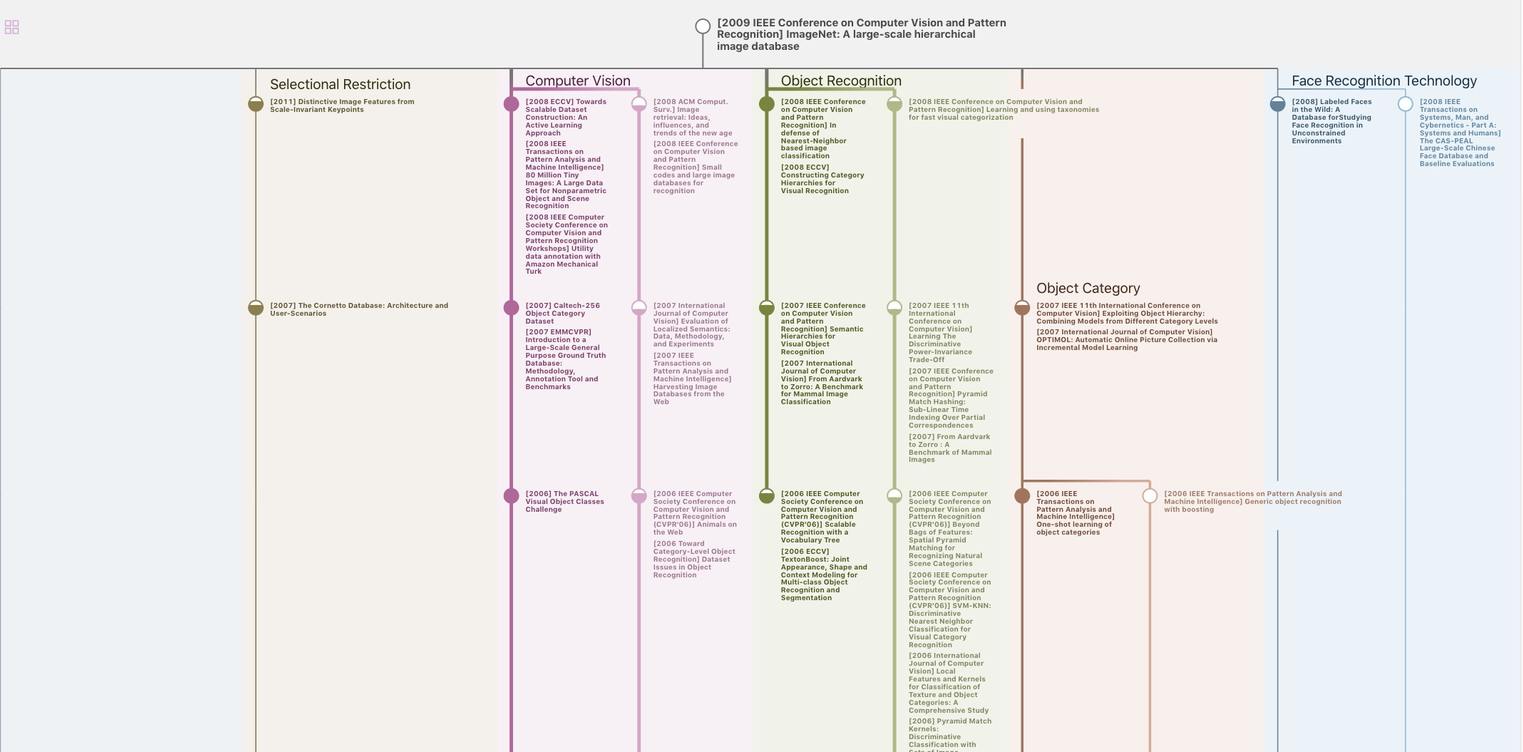
生成溯源树,研究论文发展脉络
Chat Paper
正在生成论文摘要