Long-term forecast of heatwave incidents in China based on numerical weather prediction
THEORETICAL AND APPLIED CLIMATOLOGY(2024)
Abstract
Climate change increases the frequency, duration, and intensity of extreme heatday (HD) and heatwave (HW) events. Accurate forecasting of HD and HW is crucial for disaster risk reduction. This study aimed to forecast HD and HW in 51 major cities across China by using the Global Ensemble Forecasting System (GEFS) v2 data and employing statistical downscaling. The results showed that extreme gradient boosting (XGBoost) and equidistant cumulative distribution function matching (EDCDFm) significantly enhanced the accuracy of GEFS-v2 data. However, the forecast performance declined with lead time, with a slowdown observed after 8 days. EDCDFm demonstrated the best performance in forecasting HD (1-16 days) with average values of probability of detection (POD), false alarm ratio (FAR), and critical success index (CSI) at 49.2%, 44.3%, and 35.4%, respectively, reaching an average POD as high as 70.12% in some regions. For HW (number (HWN), the longest duration (HWD), frequency (HWF), and EDCDFm achieved the most accurate forecasts (1-16 days), with average POD, FAR, and CSI values of 53.9%, 20.9%, and 45.4% for HWN; 50.6%, 22.8%, and 41.4% for HWD; and 55.6%, 18.6%, and 48.5% for HWF, respectively. Some regions achieved a minimum POD of 81.2% and a minimum CSI of 67.9%. Finally, EDCDFm exhibited even more pronounced improvements in forecasting HD and HW in the later period (9-16 days). Therefore, the use of EDCDFm enabled the effective prediction of HD and HW.
MoreTranslated text
AI Read Science
Must-Reading Tree
Example
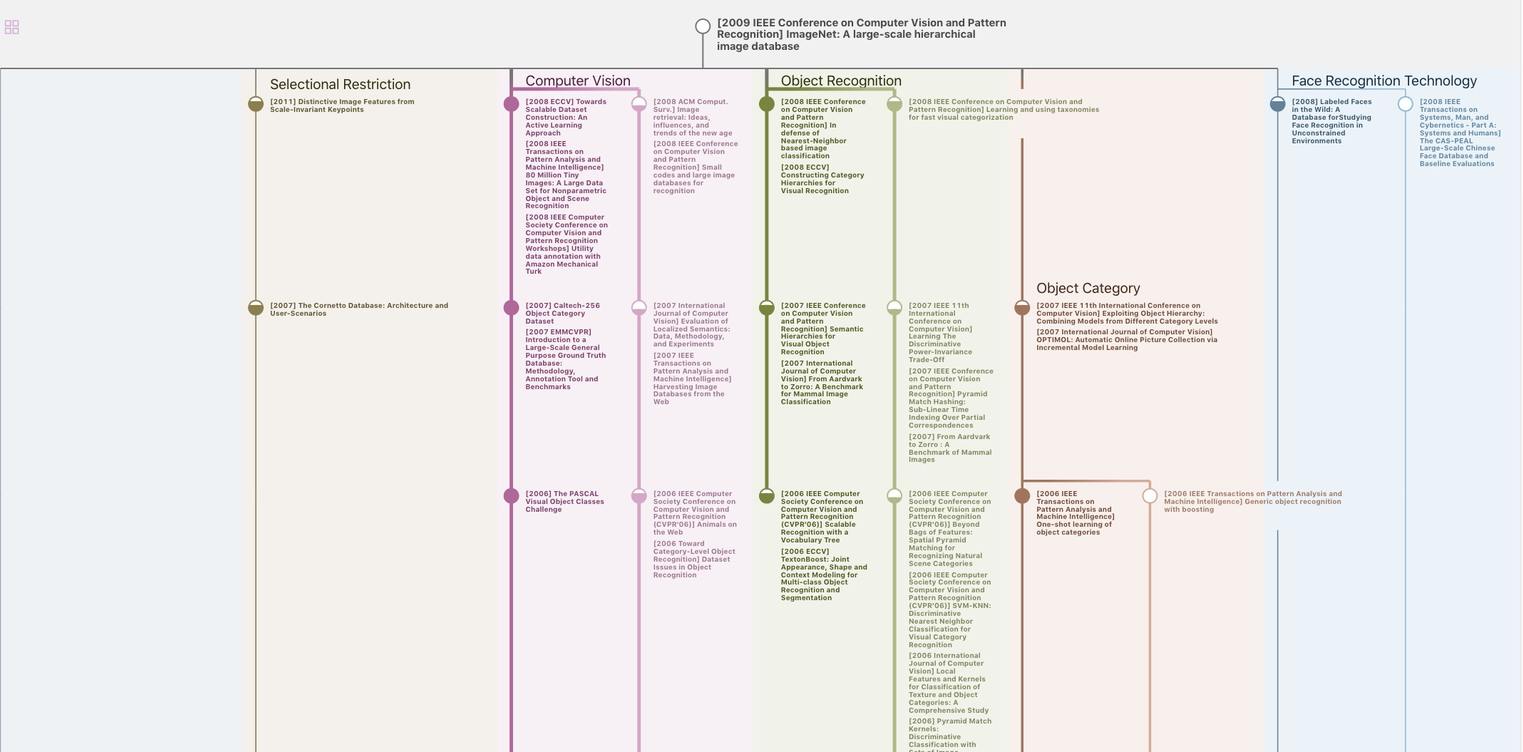
Generate MRT to find the research sequence of this paper
Chat Paper
Summary is being generated by the instructions you defined