A fast asynchronous Markov chain Monte Carlo sampler for sparse Bayesian inference
JOURNAL OF THE ROYAL STATISTICAL SOCIETY SERIES B-STATISTICAL METHODOLOGY(2024)
摘要
We propose a very fast approximate Markov chain Monte Carlo sampling framework that is applicable to a large class of sparse Bayesian inference problems. The computational cost per iteration in several regression models is of order O(n(s+J)), where n is the sample size, s is the underlying sparsity of the model, and J is the size of a randomly selected subset of regressors. This cost can be further reduced by data sub-sampling when stochastic gradient Langevin dynamics are employed. The algorithm is an extension of the asynchronous Gibbs sampler of Johnson et al. [(2013). Analyzing Hogwild parallel Gaussian Gibbs sampling. In Proceedings of the 26th International Conference on Neural Information Processing Systems (NIPS'13) (Vol. 2, pp. 2715-2723)], but can be viewed from a statistical perspective as a form of Bayesian iterated sure independent screening [Fan, J., Samworth, R., & Wu, Y. (2009). Ultrahigh dimensional feature selection: Beyond the linear model. Journal of Machine Learning Research, 10, 2013-2038]. We show that in high-dimensional linear regression problems, the Markov chain generated by the proposed algorithm admits an invariant distribution that recovers correctly the main signal with high probability under some statistical assumptions. Furthermore, we show that its mixing time is at most linear in the number of regressors. We illustrate the algorithm with several models.
更多查看译文
关键词
asynchronous MCMC sampling,Bayesian deep learning,MCMC mixing,sparse Bayesian inference,62Jxx
AI 理解论文
溯源树
样例
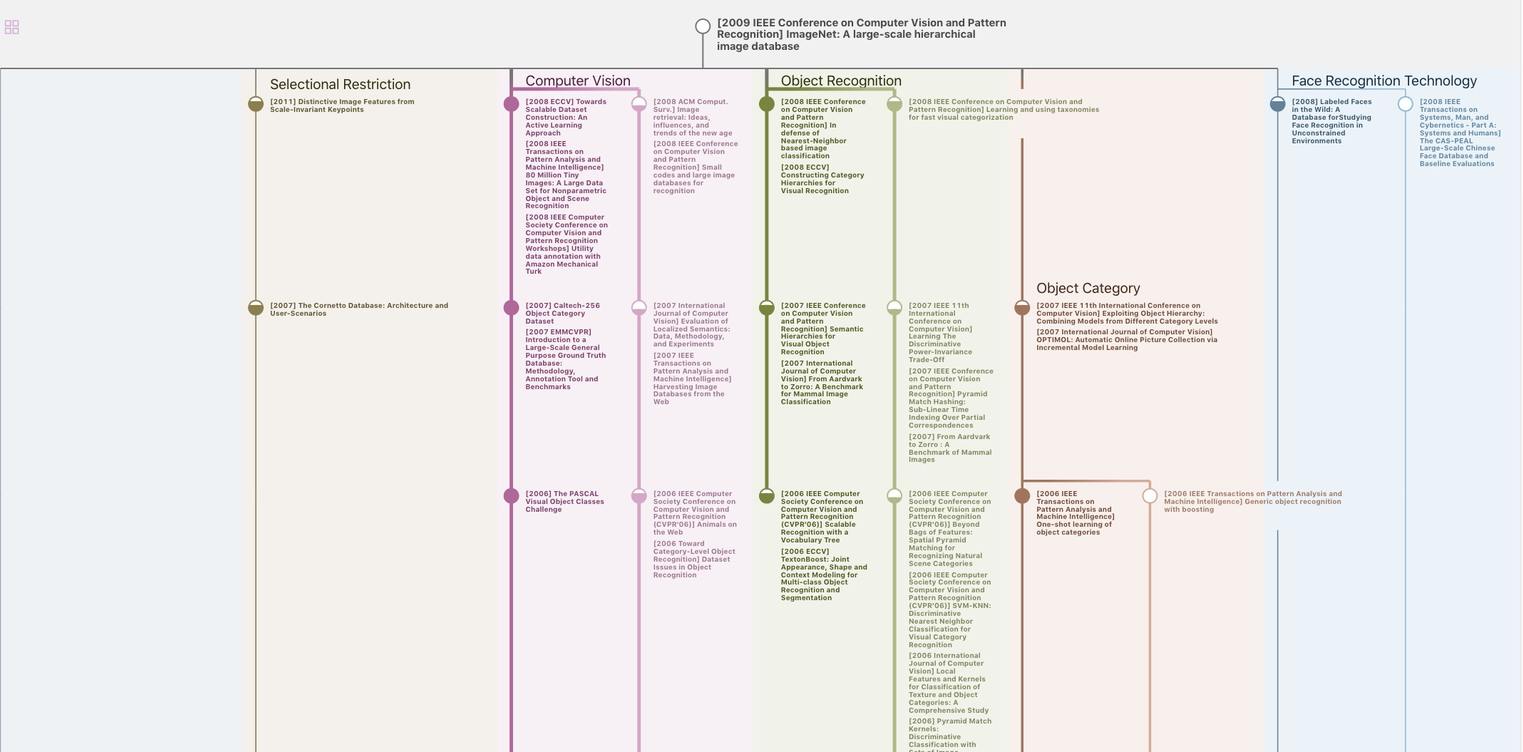
生成溯源树,研究论文发展脉络
Chat Paper
正在生成论文摘要