Simultaneous estimation of multiple soil properties under moist conditions using fractional-order derivative of vis-NIR spectra and deep learning
GEODERMA(2023)
摘要
The application of visible and near-infrared (vis-NIR) spectroscopy for predicting soil properties presents a cost-effective and time-efficient approach for evaluating various soil properties. However, the accuracy of these predictions can be adversely affected by soil moisture. Consequently, previous research focused on removing moisture effects from the spectra, resulting in the loss of valuable information on soil moisture. In this study, we propose a novel approach to directly predict both soil moisture and a range of soil properties using vis-NIR spectra. To achieve this, we employed the fractional-order derivative (FOD) technique in combination with a one-dimensional convolutional neural network (1D-CNN) to mitigate the influence of soil moisture and enhance the prediction accuracy of soil properties. We calibrated 1D-CNN, partial least squares regression (PLSR) and support vector machine (SVM) models using FOD spectra with various orders (ranging from 0 to 2 with 0.1 intervals). The results demonstrated that the FOD spectra consistently outperformed the original and integer-order differential spectra. Moreover, the 1D-CNN models exhibited superior performance compared to PLSR and SVM models in predicting soil moisture content (SMC), soil organic matter (SOM), sand, silt, clay, and iron contents. However, it is challenging to predict all six soil properties using a single 1D-CNN model with spectra of a single FOD order. Our findings identified the optimal FOD order for different soil properties. Specifically, a FOD order of 0.5 yielded the best results for predicting SMC and SOM, while an order of 0.7 was optimal for iron oxides, clay, silt, and sand contents. Consequently, we propose the combined use of FOD and 1D-CNN to estimate multiple soil properties simultaneously.
更多查看译文
关键词
Vis-NIR spectra, Soil properties, Fractional-order derivative, Deep learning
AI 理解论文
溯源树
样例
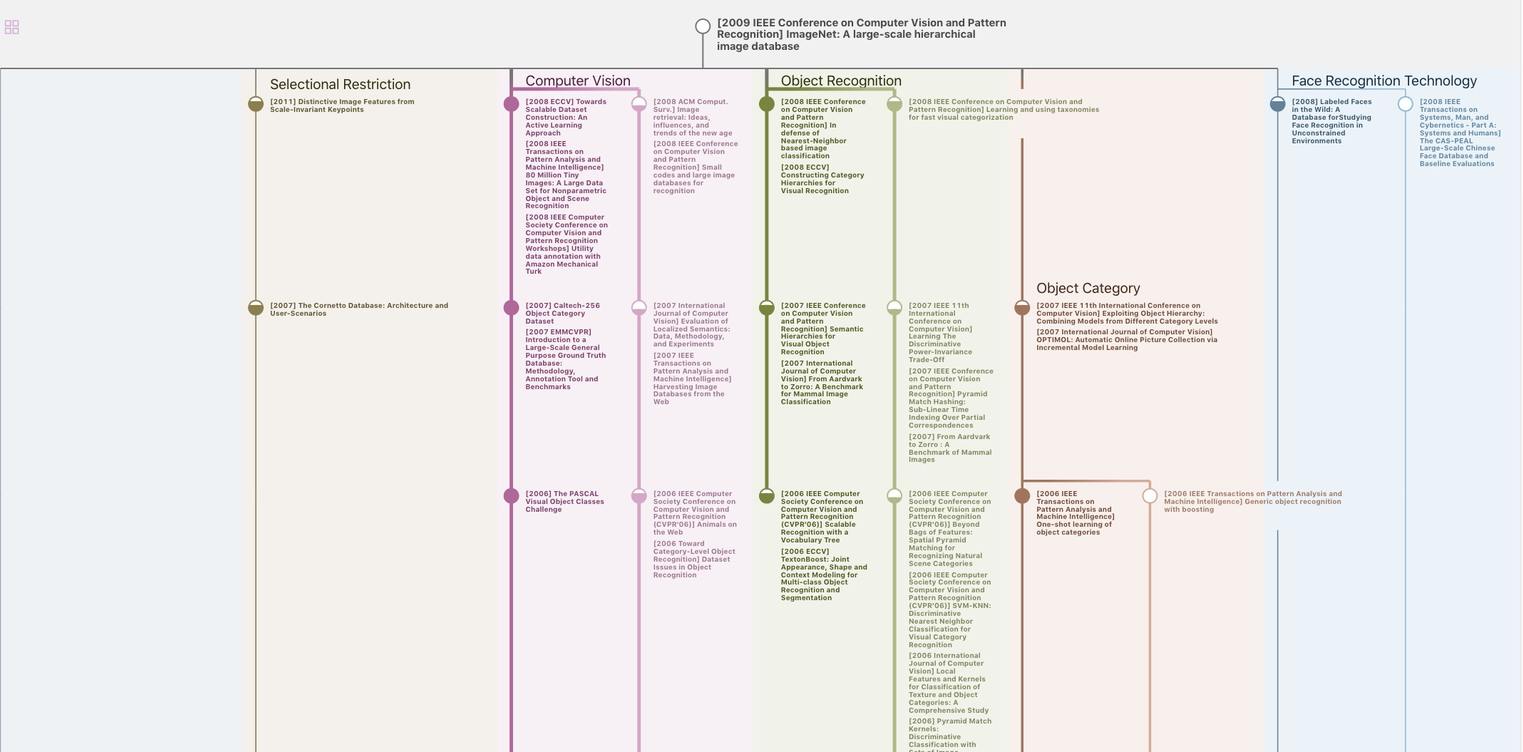
生成溯源树,研究论文发展脉络
Chat Paper
正在生成论文摘要