Automatic tuning of robust model predictive control in iterative tasks using efficient Bayesian optimization
TRANSACTIONS OF THE INSTITUTE OF MEASUREMENT AND CONTROL(2024)
Abstract
Robust model predictive control (RMPC) is an effective technology for controlling uncertain systems while robustly handling constraints, and its closed-loop performance heavily relies on the selection of objective functions. However, the objective functions are typically chosen to be close to the real control objectives, despite an objective function that leads to less conservative constraints often provides better closed-loop performance. In this paper, we propose an automatic tuning framework for RMPC in iterative tasks. In particular, we parameterize RMPC and develop a Bayesian optimization (BO) method to tune it by solving a black-box optimization problem. We then introduce an efficient transfer learning framework within BO, which speeds up the searching process and enhances the controller performance. The effectiveness of the proposed tuning framework is illustrated on numerical examples.
MoreTranslated text
Key words
Robust model predictive control,Bayesian optimization,iterative learning control
AI Read Science
Must-Reading Tree
Example
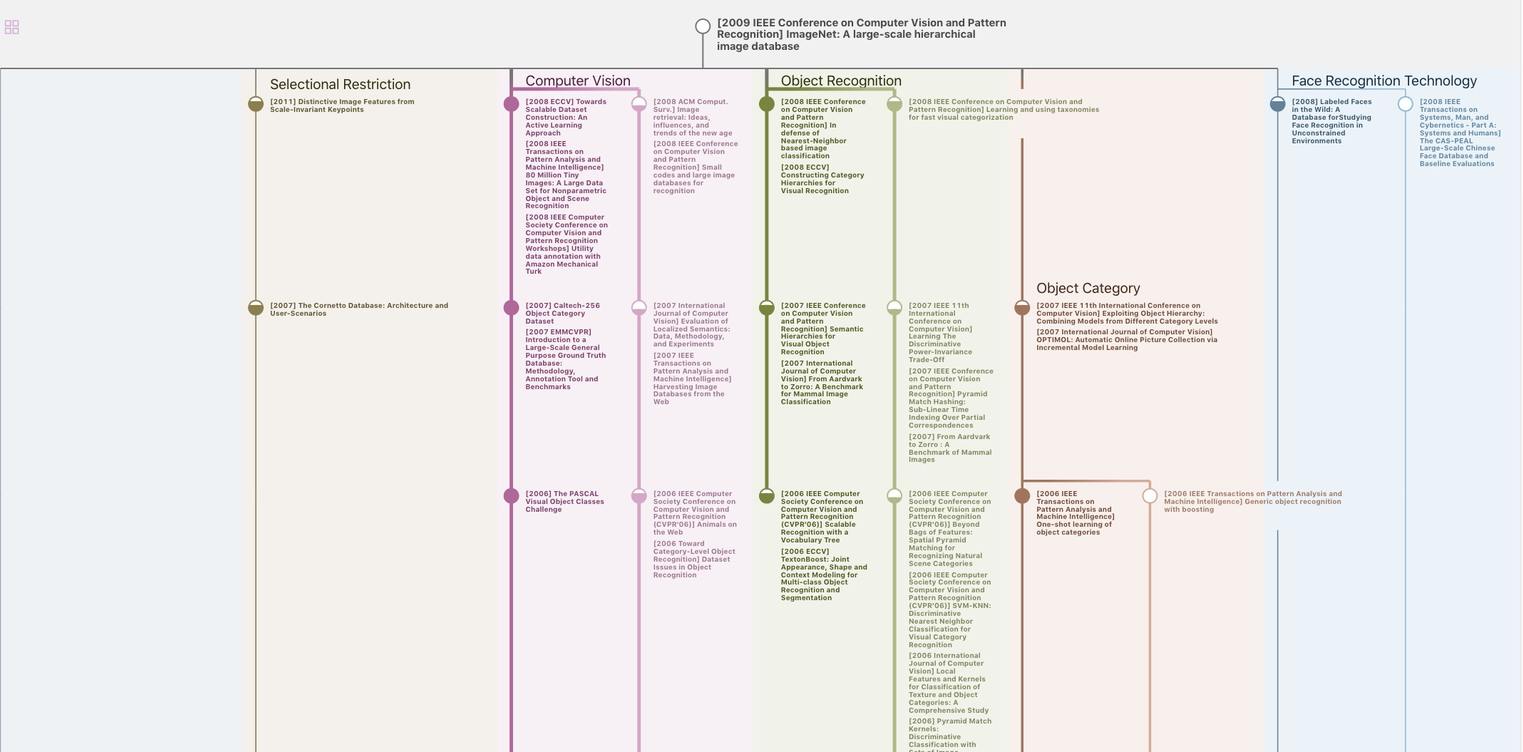
Generate MRT to find the research sequence of this paper
Chat Paper
Summary is being generated by the instructions you defined