Granger-Based Root Cause Diagnosis with Improved Backward-in-Time Selection
2023 IEEE 12TH DATA DRIVEN CONTROL AND LEARNING SYSTEMS CONFERENCE, DDCLS(2023)
摘要
With the emergence of data science and large number of sensor data, data-based disturbance root diagnosis methods have attracted widespread attention. Among these methods, the Granger causality test is one of the most common methods which can infer causal associations between signals based on temporal precedence. However, the causal effect between process variables often has some lag characteristics, but the response variable does not depend on all lag of the cause variable. The Granger causality analysis method based on VAR model does not consider the different lag dependency structures in the multivariate time series, which may lead to inaccurate causality test. In view of the above problems, this paper constructs the regression model through Improved Backward-in-Time Selection algorithm, realizes the supervised stepwise selection guided by the order of variable lag, obtains the optimal lag structure of the regression model through stepwise evaluation, and tests the Granger causality on this basis. The effectiveness of the proposed method is further proved by the fault source diagnosis experiment of Tennessee Eastman process.
更多查看译文
关键词
Granger causality, Cointegration, Error correction model, Non-stationary time series
AI 理解论文
溯源树
样例
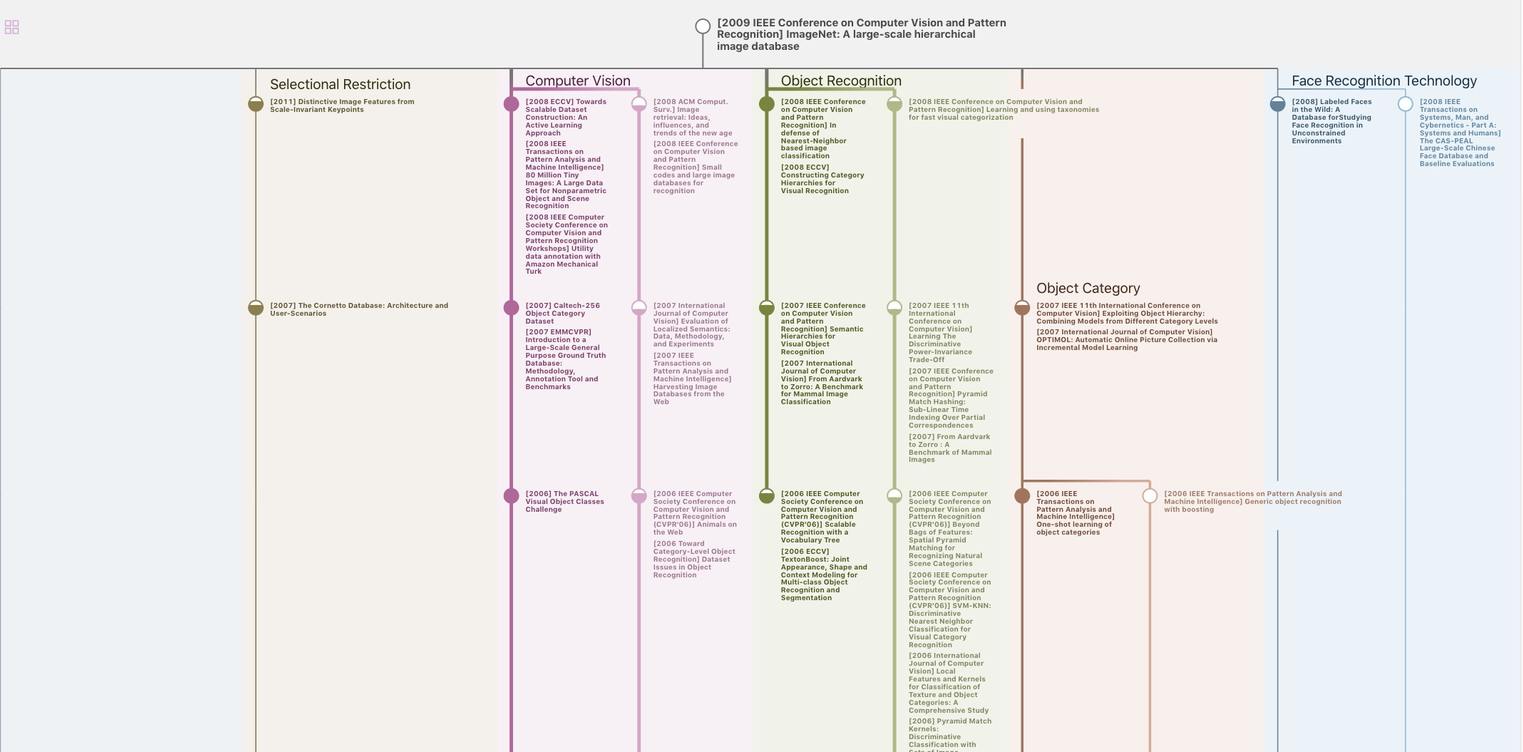
生成溯源树,研究论文发展脉络
Chat Paper
正在生成论文摘要