Transfer Reinforcement Learning of Robotic Grasping Training using Neural Networks with Lateral Connections
2023 IEEE 12TH DATA DRIVEN CONTROL AND LEARNING SYSTEMS CONFERENCE, DDCLS(2023)
Abstract
Reinforcement learning, as an effective framework for solving continuous decision tasks in machine learning, has been widely used in manipulator decision control. However, for manipulator grasping tasks in complex environments, it is difficult for intelligence to improve performance by exploring to obtain high-quality interaction samples. In addition, the training models of reinforcement learning usually lack task generalization and need to be relearned to adapt to task changes. To address these issues, researchers have proposed transfer learning that uses external prior knowledge to help the target task to improve the reinforcement learning process. In this paper, the transfer of the manipulator grasping source task to the grasping target task based on the deep Q-network algorithm is achieved by constructing lateral connections between fully convolutional neural networks using Densenet. Experimental results in the CoppeliaSim simulation environment show that the methods successfully achieve inter-task transfer by constructing lateral connections between fully convolutional neural networks. The validated transfer reinforcement learning approach improves the effectiveness of task training while reducing the complexity of the network due to lateral connections.
MoreTranslated text
Key words
Transfer learning,deep reinforcement learning,lateral connections,manipulator
AI Read Science
Must-Reading Tree
Example
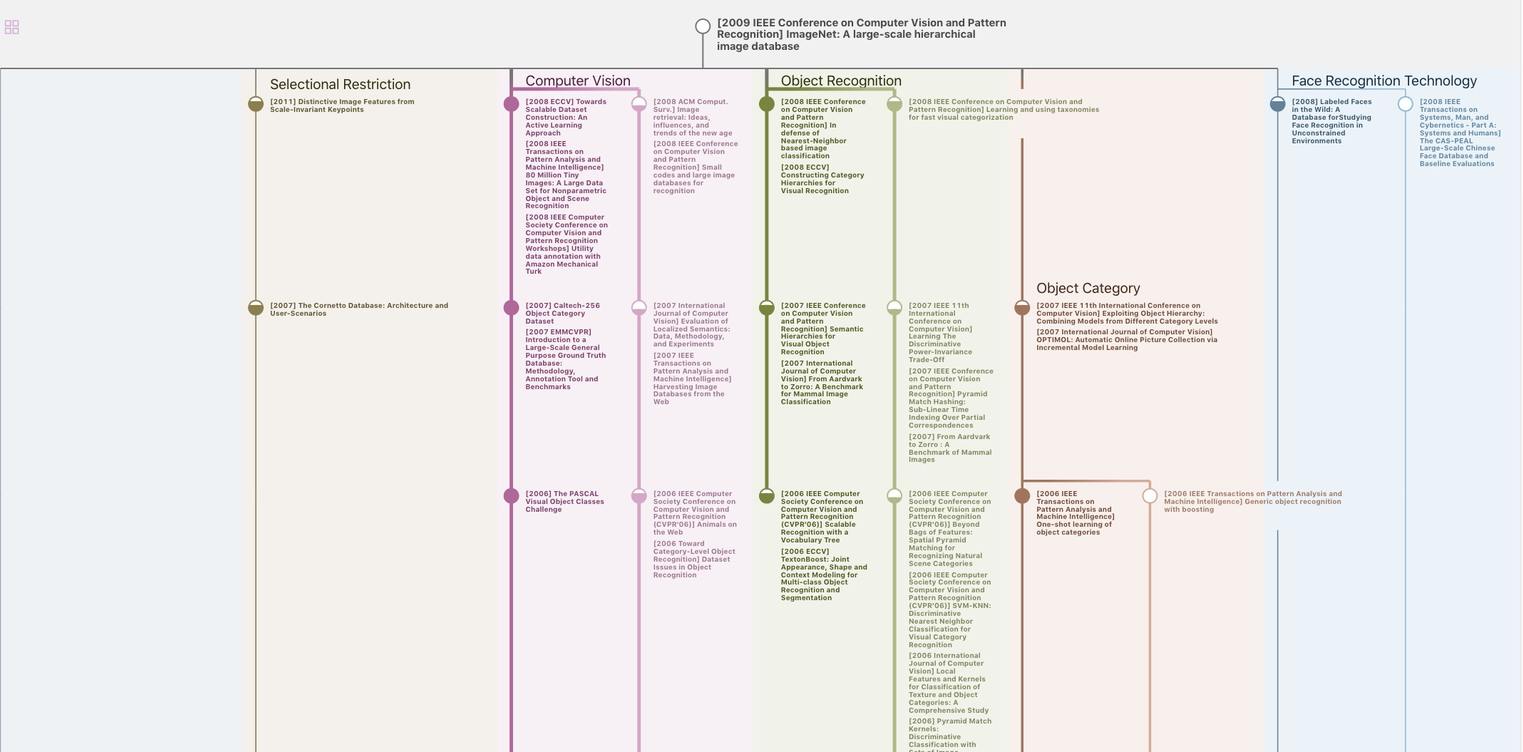
Generate MRT to find the research sequence of this paper
Chat Paper
Summary is being generated by the instructions you defined