Adaptive Quasi-Newton Algorithm for Remote Sensing
2023 IEEE 12TH DATA DRIVEN CONTROL AND LEARNING SYSTEMS CONFERENCE, DDCLS(2023)
摘要
Recurrent neural networks (RNNs) have been widely applied to model time series and temporal data due to inherent memory storing past information. However, the optimization algorithms for RNNs either only exploit the first-order information with low computational complexity or gain the second-order information with heavy per-iteration cost. In this paper, an adaptive quasi-Newton algorithm (ADA-L-BFGS) is proposed on the basis of limited-memory BFGS algorithm whose memory size can be determined adaptively during the process of iteration and used to train deep neural networks for extracting deep features of hyperspectral data. Our algorithm can retain low per-iteration cost and ensure fast convergence. It is emphasized that ADA-L-BFGS has two appealing features. One is that multiple memory sizes are selected to construct hybrid search direction for strengthening the usage of recent curvature information. Another is that a promising calculation criterion for step size is introduced to achieve efficient training of deep neural networks for hyperspectral images classification. Experimental results clearly illustrate that the proposed ADA-L-BFGS can achieve faster convergence and better performance than previous methods.
更多查看译文
关键词
Adaptive Quasi-Newton Algorithm,Remote Sensing,Hyperspectral Images,Recurrent Neural Networks,Hybrid Search Direction
AI 理解论文
溯源树
样例
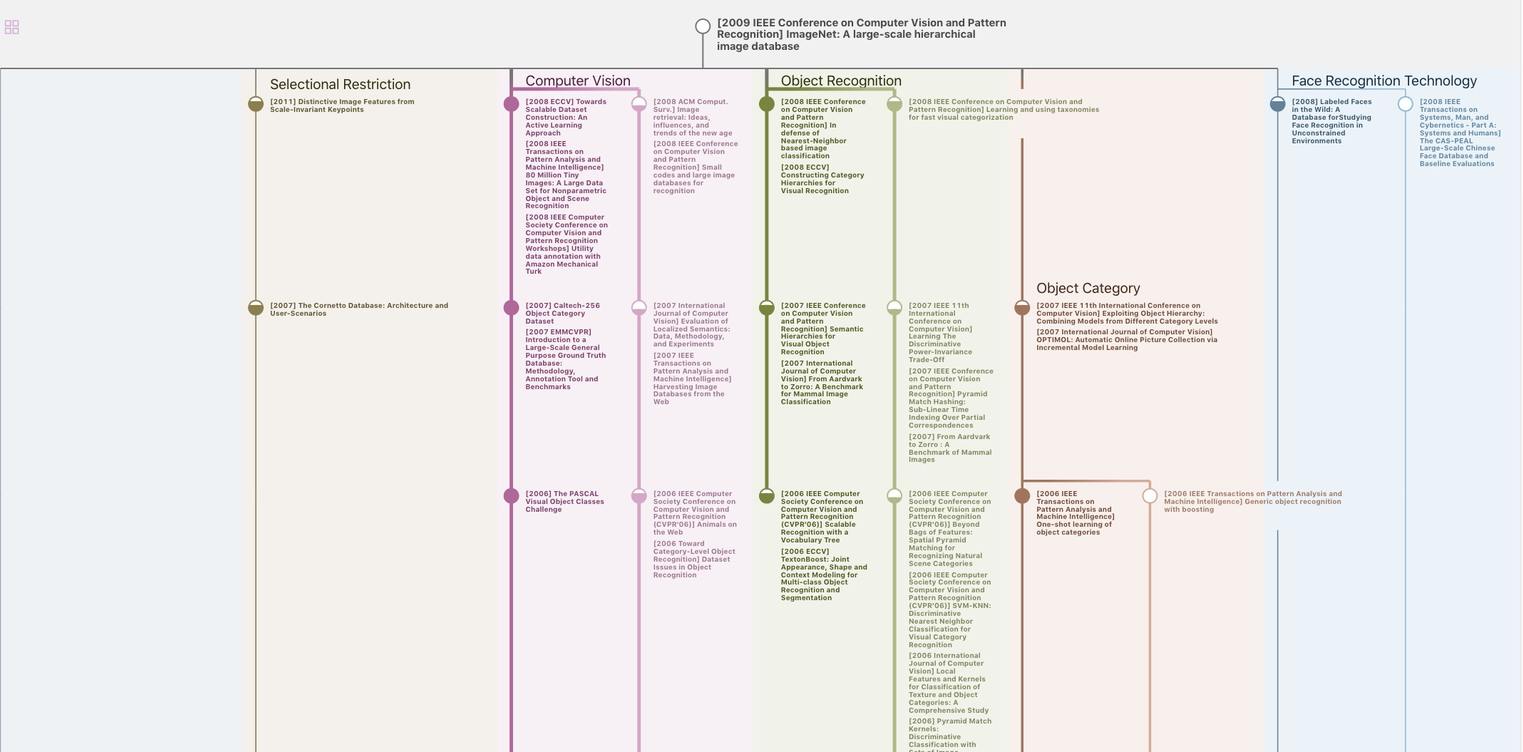
生成溯源树,研究论文发展脉络
Chat Paper
正在生成论文摘要