Optimizing Data Cleaning Methods for Remotely Collected Electronic Weight Measurements
DIABETES(2023)
摘要
Background: Digital smart scales transmit weight measurements collected outside of clinical settings to medical and research teams. Statistical packages such as SAS %TPF (TrueProfileFinder) can ensure weights belong to persons of interest instead of others who may use the scale; however, validation is required. Methods: We obtained 32,917 remotely collected weights from 222 postpartum participants using BodyTrace scales in the Pregnancy, Environment, and Lifestyle Study-2 (PETALS-2). To assess the SAS %TPF macro's performance, we applied 18 combinations of its three modifiable parameters (number of observations in a moving window, importance of weights from clinic visits, and prediction band) to a random subsample (N=16). In parallel, two manual reviewers independently classified weights as ‘belonging’ or ‘not belonging’ to participants; Cohen's κ ≥0.91 confirmed adequate interrater agreement. Outputs from the 18 models were then compared to the manual review. Results: As hypothesized, adjusting parameters impacted the macro's ability to identify weights truly belonging to participants (percent true calls). Prediction band, the predicted range for new observations based on a linear regression model, was the only parameter associated with macro performance. A prediction band of 15 lbs. was associated with a 2.71% (95% CI: 0.16%, 5.25%) increase in percent true call and 5.57% (95% CI: 1.77%, 9.38%) increase in sensitivity vs. a band of 10 lbs. Percent true call for a prediction band of 15 lbs. was 2.95% higher, but non-significant (p=0.18) vs. a band of 20 lbs. A prediction band of 15 lbs. was highly accurate (>97% true call) and sensitive (>98%). Conclusion: Weight is often assessed in interventions for glycemic control and diabetes prevention. Precise data cleaning can increase accuracy of unsupervised, remotely collected measurements. Clinicians and researchers using smart scales can apply this data cleaning method to identify SAS %TPF macro parameters that maximize accuracy. Disclosure S.Daredia: None. B.P.Smith: None. H.Serrato bandera: None. A.Millman: None. C.Quesenberry: None. A.Ferrara: None. S.D.Brown: None. Funding National Institutes of Health (R01HL142996)
更多查看译文
关键词
data cleaning methods,weight
AI 理解论文
溯源树
样例
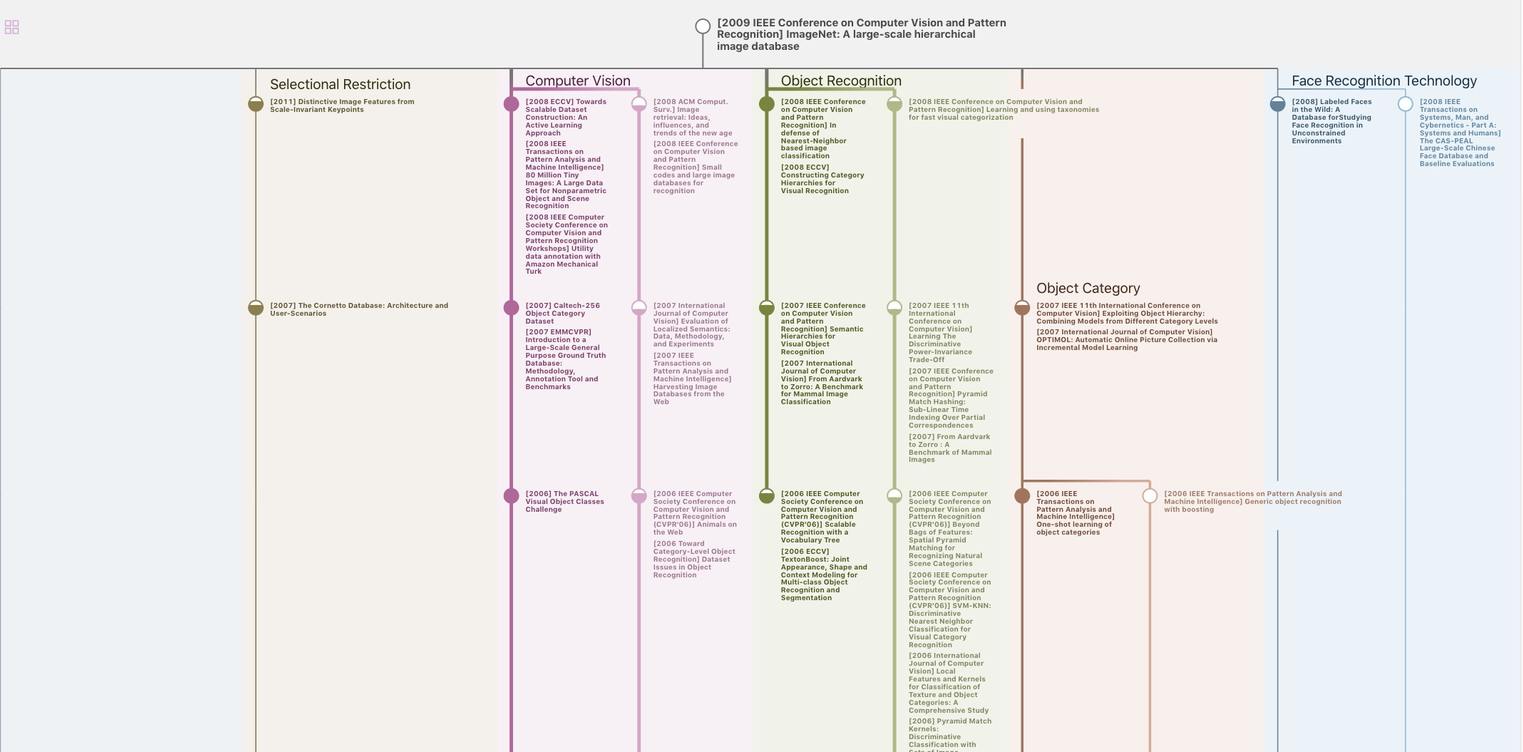
生成溯源树,研究论文发展脉络
Chat Paper
正在生成论文摘要