PMP: A partition-match parallel mechanism for DNN inference acceleration in cloud-edge collaborative environments
JOURNAL OF NETWORK AND COMPUTER APPLICATIONS(2023)
摘要
To address the challenges of delay-sensitive deep learning tasks, Deep Neural Network (DNN) models are often partitioned and deployed to the cloud-edge environment for parallel and collaborative inference. However, existing parallel coordination mechanisms are not suitable for the cloud-edge environment, as the high inter-layer dependence of DNNs can increase transmission latency and wait times for inference, which contradicts the advantage of low latency in edge computing. To resolve this contradiction, the PMP mechanism takes into account the inter-layer transfer dependence of partitioning solutions and employs a multi-objective equalization algorithm to derive DNN model partitioning strategies suitable for multi-way parallel computing. Moreover, the mechanism establishes a DNN inference time prediction model based on these partitions and utilizes an iterative matching algorithm to approximate an optimal DNN inference workflow. Extensive evaluations of the proposed mechanism are conducted using various DNN models, and the results demonstrate its superiority over existing schemes, including local, CoEdge, and EdgeFlow. Notably, PMP achieves significant reductions in total inference latency compared to these schemes, with reductions of 80.9%, 37.9%, and 9.1%, respectively.
更多查看译文
关键词
Edge computing (EC),Deep neural networks (DNNs),Parallel computing,Offloading,Cloud–edge collaboration
AI 理解论文
溯源树
样例
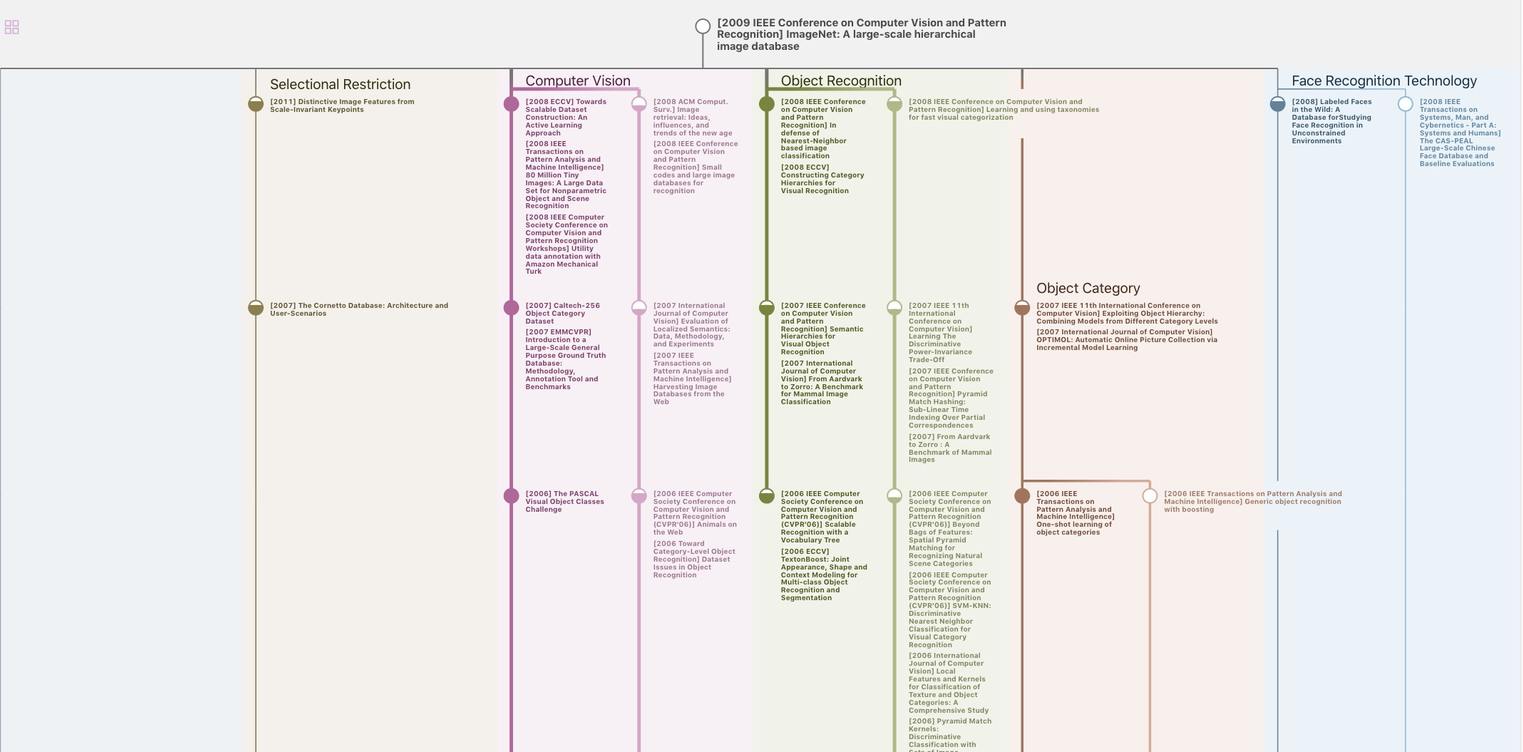
生成溯源树,研究论文发展脉络
Chat Paper
正在生成论文摘要