Assessing Performance and Limitations of a Device-Level Machine Learning Approach for Perovskite Solar Cells with an Application to Hole Transport Materials
SOLAR RRL(2023)
摘要
Machine learning models have become widespread in materials science research. An open-access and community-driven database containing over 40 000 perovskite photovoltaic devices has been recently published. This resource enables the application of predictive data-driven models to correlate device structure with photovoltaic performance, whereas the literature usually focuses on specific device layers. Herein, the concept of device-level performance prediction is explored using gradient-boosted regression trees as the core algorithm and Shapley values analysis to interpret and rationalize the results. The main pitfalls and conceptual limitations of the approach are discussed and correlated with the database structure and dimension, by comparing the performance of different choices of descriptors and dataset size. Evidence suggests that the additional features introduced herein, in particular chemical descriptors of perovskite additives, can boost regression performance at a device level. A specific model is finally trained to predict the performance of unseen devices and tested on experimental data from the literature. This task is found to be particularly challenging, as the ability of the model to generalize to a new chemical space is limited by several factors, including the amount and the quality of available data.
更多查看译文
关键词
gradient-boosted decision trees,machine learning,perovskite solar cells
AI 理解论文
溯源树
样例
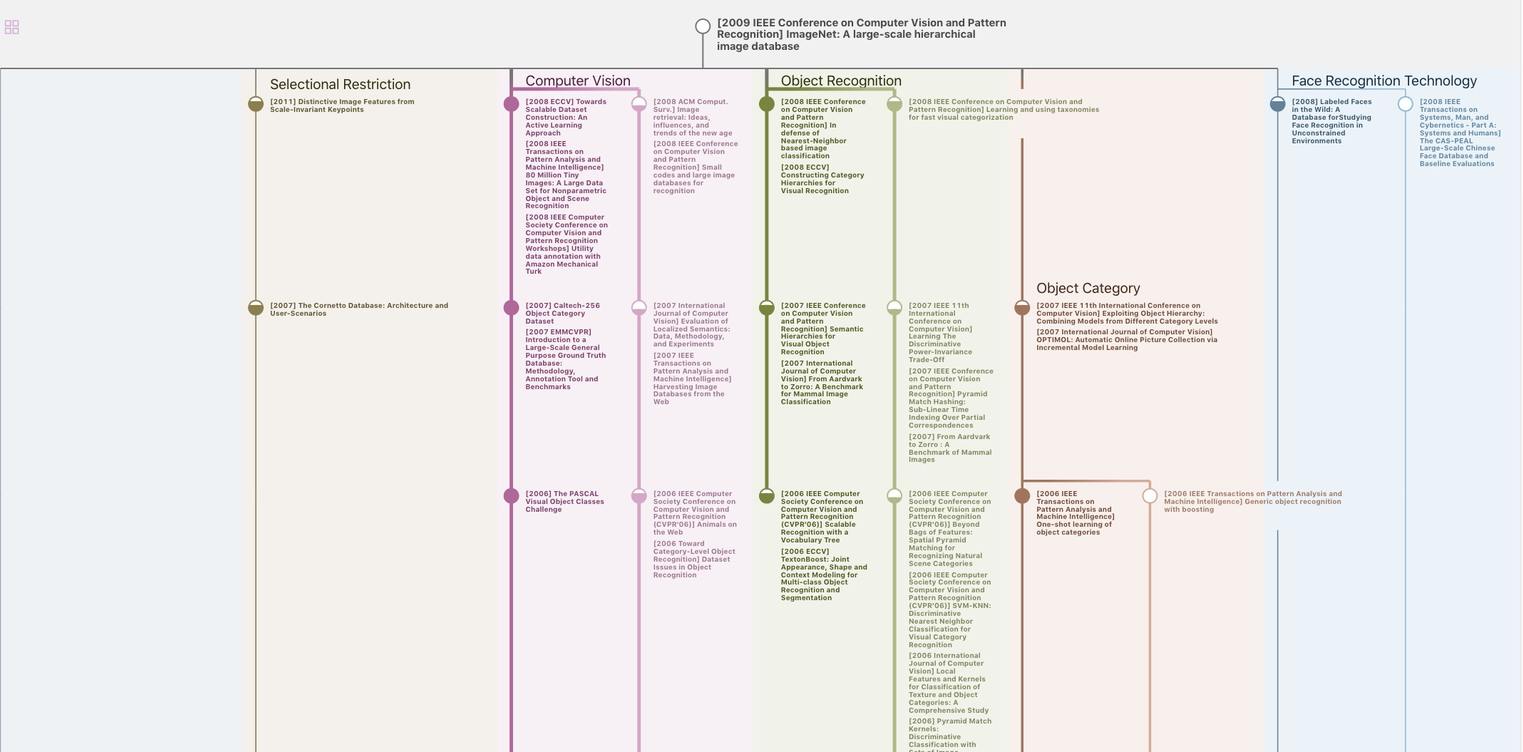
生成溯源树,研究论文发展脉络
Chat Paper
正在生成论文摘要