Augmenting NIR Spectra in deep regression to improve calibration
CHEMOMETRICS AND INTELLIGENT LABORATORY SYSTEMS(2023)
Abstract
Deep learning, particularly with convolutional neural networks, shows promise in modelling near-infrared spectroscopy (NIRS), but the lack of robust generalisation across instruments often affects performance in practice. Here, we investigate a method to increase the robustness of this approach. The proposed method involves using a simple data augmentation technique during the training process. The performance of convolutional neural network regression is compared to partial least squares regression (PLSR) using kiwifruit data collected from multiple handheld devices over three seasons and mango data collected from a single device over four seasons. The results suggest that data augmentation for NIR spectra can prevent overfitting. In particular, augmenting the training data to mimic spectra collected over multiple devices results in a neural network model with improved performance over PLSR.
MoreTranslated text
Key words
Near-infrared spectroscopy,Convolutional neural networks,Partial least squares regression,Data augmentation
AI Read Science
Must-Reading Tree
Example
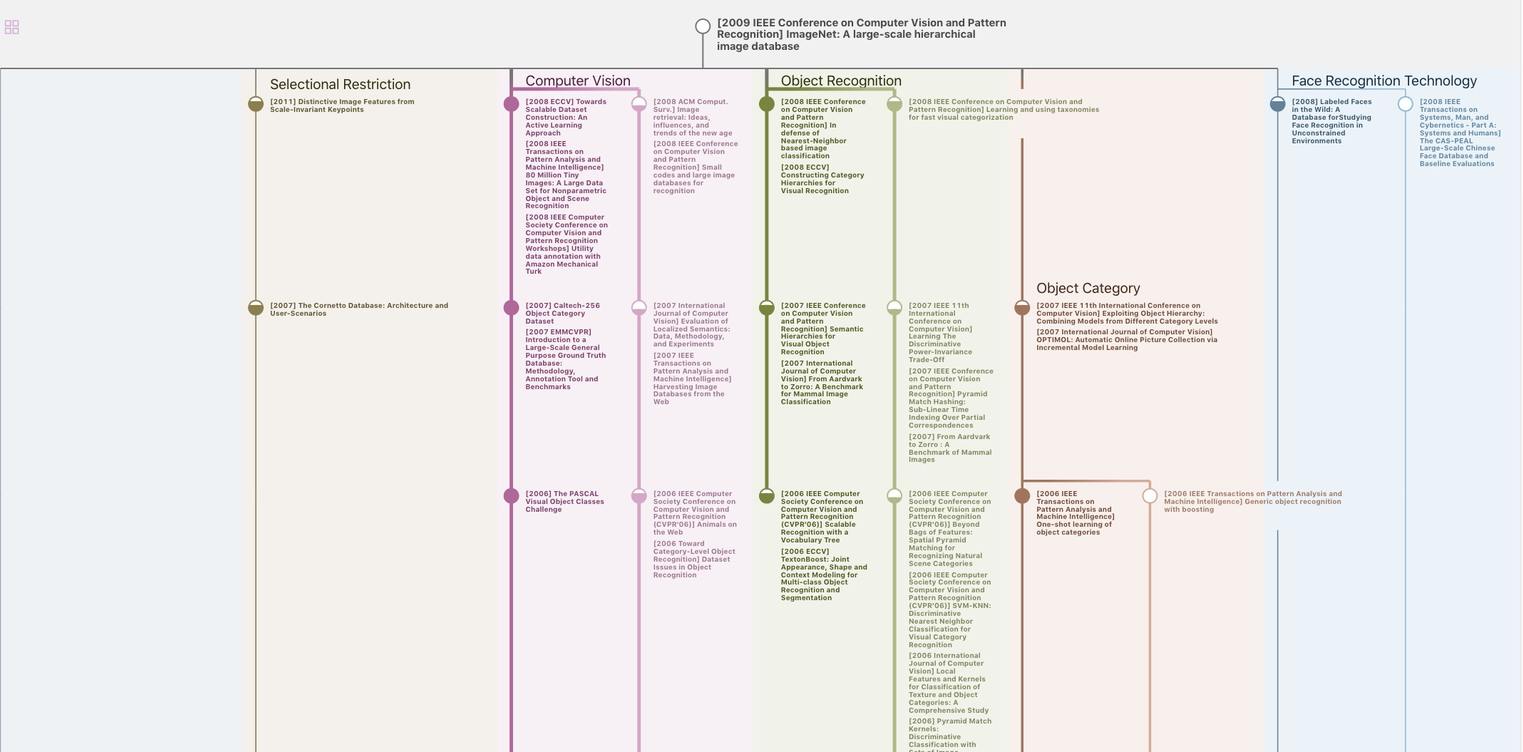
Generate MRT to find the research sequence of this paper
Chat Paper
Summary is being generated by the instructions you defined