Two-layer coordinated reinforcement learning for traffic signal control in traffic network
EXPERT SYSTEMS WITH APPLICATIONS(2024)
摘要
Intersection traffic signal control considering vehicle emissions has become an important topic, however, the decision complexity of traffic signal control increases dramatically in a dynamic traffic environment with multiintersections. It is a severe challenge to coordinate traffic signals at multi-intersections based on Internet of Things information to improve the traffic condition of the road network. This paper proposes a two-layer coordination algorithm based on multi-agent reinforcement learning-Multi-agent Coordinated Policy Optimization (MACoPO), for solving traffic signal control at multi-intersections. MACoPO consists of local cooperation, which adjusts the weights of individual rewards and neighborhood agents' rewards by using local cooperation factors (LCF), and global coordination, which updates the LCF to maximize global rewards. The state and reward functions are designed in terms of the current state of the signal, waiting queue length, vehicle density and emission concentration in the lane, vehicle delay, and vehicle emissions, thus making full use of the intersection state information. The proposed method is extensively assessed through simulation experiments using artificial and real road networks and the numerical results confirm its effectiveness in complex and dynamic real-time traffic environments with multi-intersections.
更多查看译文
关键词
Smart transportation,Deep reinforcement learning,Multi-agent,Traffic light control
AI 理解论文
溯源树
样例
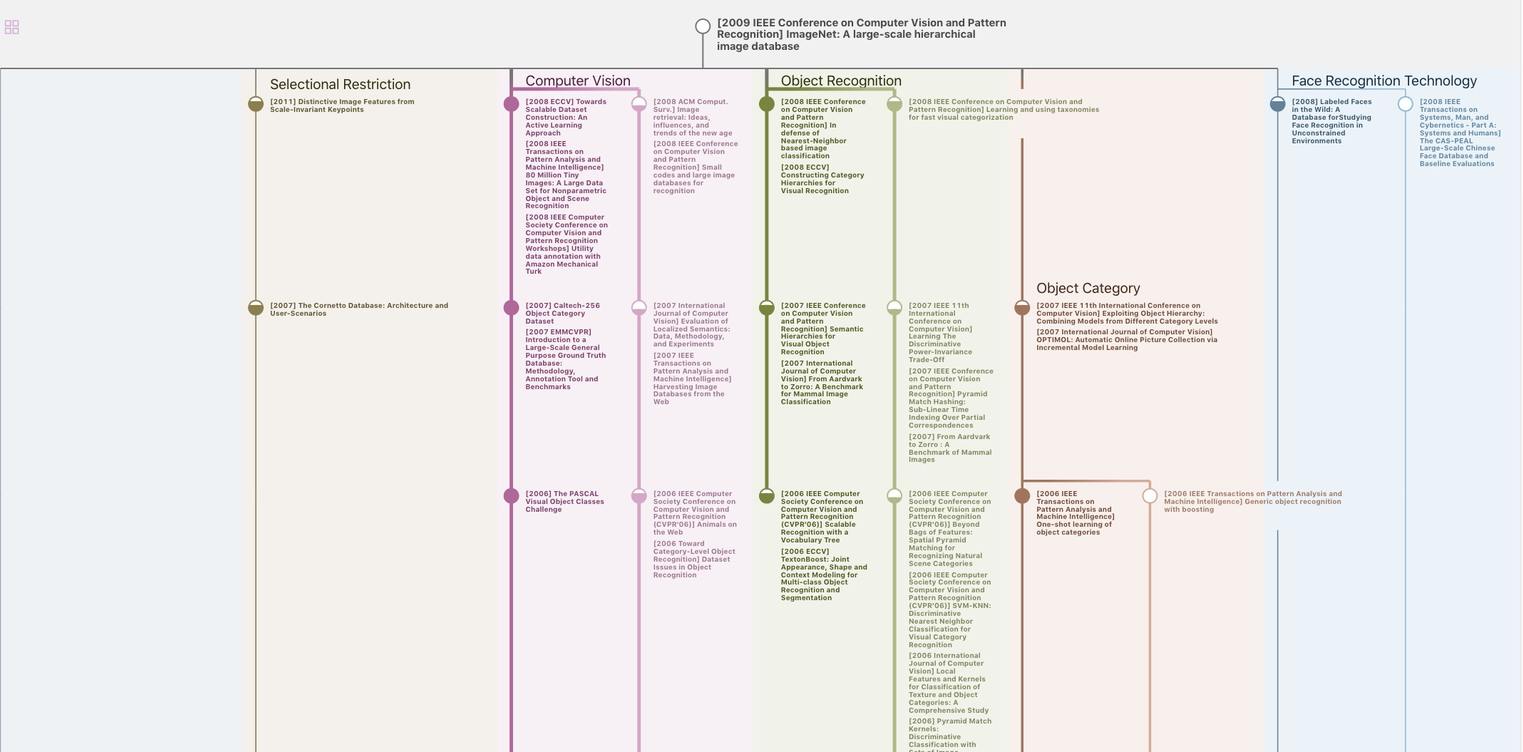
生成溯源树,研究论文发展脉络
Chat Paper
正在生成论文摘要