Dynamic contrastive learning guided by class confidence and confusion degree for medical image segmentation?
PATTERN RECOGNITION(2024)
Abstract
This work proposes an intra-Class-confidence and inter-Class-confusion guided Dynamic Contrastive (CCDC) learning framework for medical image segmentation. A core contribution is to dynamically select the most expressive pixels to build positive and negative pairs for contrastive learning at different training phases. For the positive pairs, dynamically adaptive sampling strategies are introduced for sampling different sets of pixels based on their hardness (namely the easiest, easy, and hard pixels). For the negative pairs, to efficiently learn from the classes with high confusion degree w.r.t a query class (i.e., a class containing the query pixels), a new hard class mining strategy is presented. Furthermore, pixel-level representations are extended to the neighbourhood region to leverage the spatial consistency of adjacent pixels. Extensive experiments on the three public datasets demonstrate that the proposed method significantly surpasses the state-of-the-art.
MoreTranslated text
Key words
Class confusion degree,Dynamic contrastive learning,Medical image segmentation
AI Read Science
Must-Reading Tree
Example
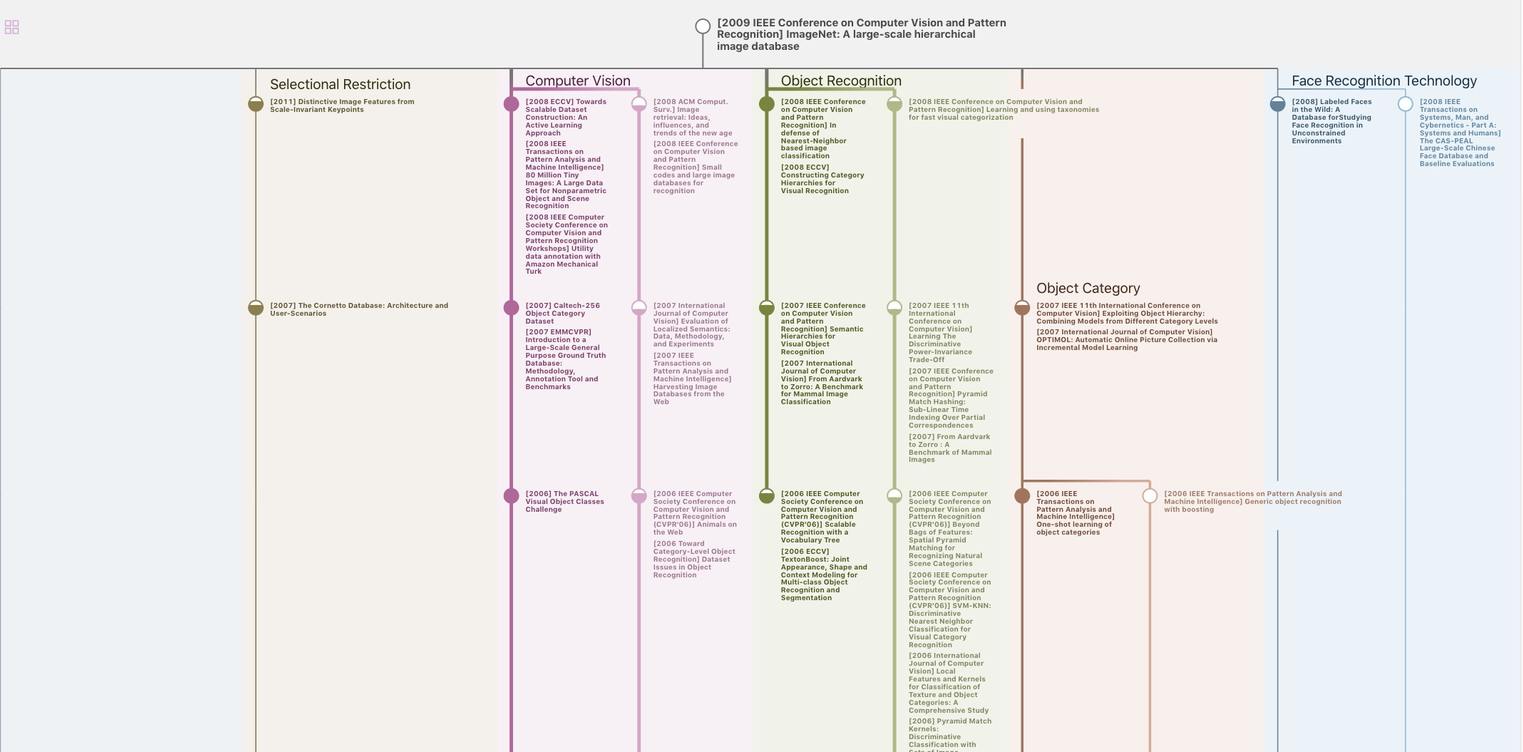
Generate MRT to find the research sequence of this paper
Chat Paper
Summary is being generated by the instructions you defined