Progressive decision-making framework for power system topology control
EXPERT SYSTEMS WITH APPLICATIONS(2024)
摘要
Topology control is an efficient and low-cost measure to adjust the power flow to ensure the security and economic operation of power systems. Since topology control involves large-scale action space and is difficult to solve by traditional optimization methods, the reinforcement learning paradigm has been regarded as a promising solution in recent years. However, the effective action space of topology control is too sparse to explore a useful policy for conventional reinforcement learning. To explicitly utilize the hierarchical semantic information of different actions to reduce the exploration space, this paper presents a novel progressive decision-making framework that enables the agent to carry out an effective action of topology control in multiple stages like human operators. The proposed framework uses a graph convolution network to extract the node-level and graph-level features, that explicitly account for the topological information with different granularity concealed in the power system. Based on the features of different receptive field precision from coarse to fine, the framework distinguishes whether the power system is secure and requires intervention in the first stage. Then the pivotal node is located in the second stage, and the corresponding bus assignment action is performed on this node in the last stage. Simulation results on two different power systems show that the proposed framework significantly reduces the action space of topology control and improves performance compared with several state-of-the-art reinforcement learning methods.
更多查看译文
关键词
Deep reinforcement learning,Graph neural networks,Progressive decision-making,Power systems,Topology control
AI 理解论文
溯源树
样例
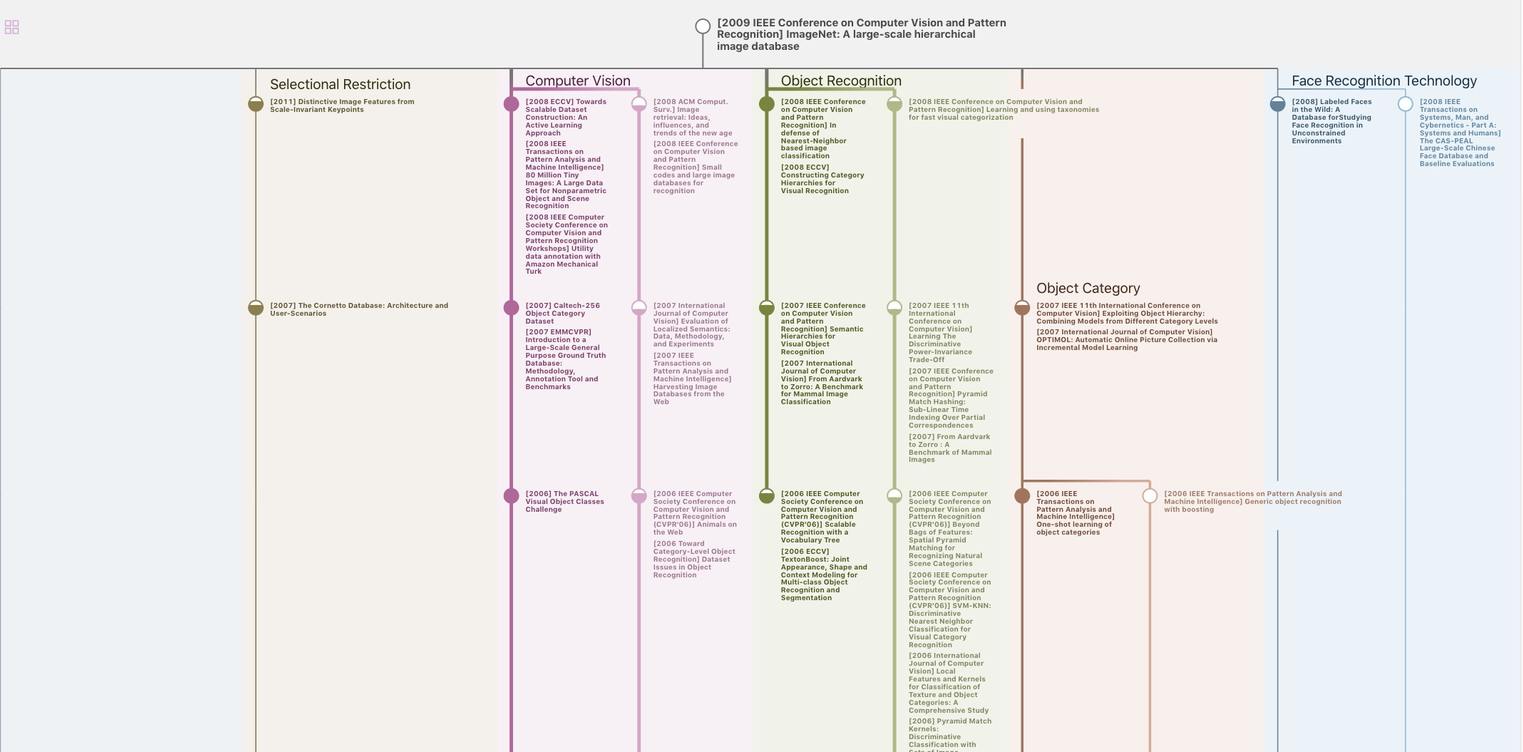
生成溯源树,研究论文发展脉络
Chat Paper
正在生成论文摘要