Metabolic classification of embryos and oocytes based on hyperspectral imaging and machine learning
HUMAN REPRODUCTION(2023)
Abstract
Abstract Study question To classify embryos according to their metabolic fingerprint with a robust, non-invasive methodology Summary answer Our Hyperspectral (HS) phasor analysis coupled to artificial intelligence simultaneously detects 6+ metabolites to profile and classify competent embryos and oocytes safely and consistently. What is known already Metabolism plays a key role in oocyte and embryo developmental competence. However, current methods to measure oocyte and embryo metabolism are either invasive, phototoxic, too slow or based on indirect analysis in cumulus cells or culture media. Some intracellular metabolites are autofluorescent and their concentration can be quantified using HS imaging when using the correct light excitation. HS imaging is an optical technique whereby the full emission spectrum is obtained for each pixel of an image. Thus, raw HS data encodes rich metabolic information of an imaged cell as numerous metabolites are excited simultaneously. Study design, size, duration A total of 96 mouse embryos and 115 oocytes were analyzed. In vivo fertilized embryo cohorts included control, glucose-starved, pyruvate/lactate-starved, and glucose/pyruvate/lactate-starved. Oocyte cohorts included oocytes obtained from young female mice analyzed blindly either immediately after collection or after overnight culture (in vitro aged), and from old female mice. After HS analysis, oocytes were inseminated by ICSI and cultured in vitro to correlate their potential to develop up to blastocyst with their metabolic profiles. Participants/materials, setting, methods Samples were imaged using multiphoton illumination near the infra-red to avoid phototoxicity. The HS detection covered the whole visible spectrum allowing simultaneous measurement of 6+ relevant metabolites for the embryo/oocyte biology: NADH (free and bound), FAD+, retinol, retinoic acid, and flavins among others. Afterwards, we applied dimensionality reduction (2D phasor image) from which machine learning algorithms learned to classify the different samples. We used Akaike Information Criterion (AIC) model. Cross validation was performed using 80%/20%. Main results and the role of chance The Area Under the ROC Curve (AUC) achieved by binary classification as well as the average class-accuracy (computed as the average of the confusion matrix diagonal) were calculated in four-class classification in the case of embryos. [EM1] Brightfield images of the test set of embryos were evaluated by 4 embryologists to look for compromised embryos. Human graders reached an AUC of 51% of the samples using brightfield compared to 93.7% of the AI algorithm using HS-Phasor. A similar approach using the HS-Phasor was conducted on the different cohorts of mouse oocytes. It was able to separate the oocyte classes with an average AUC of 96.2% and make a statistical correlation with their blastulation efficiency with a 82.2% AUC. The viability of embryos and oocytes after imaging was comparable to that of non-imaged controls, indicating that the imaging procedure had no effect on the posterior in vitro development of the embryos. Limitations, reasons for caution The cohort size is limited although statistically robust. Blastulation efficiency will need to be complemented (ongoing work) with implantation measurements. Wider implications of the findings The HS-phasor method has the potential to become a paradigm shift in IVF embryo selection due to the reliable, non-invasive direct measurement of the oocyte/embryo physiology. The adaptation into clinical practice can be straightforward as it offers an additional layer of information for oocyte/embryo assessment without compromising time nor viability. Trial registration number NA
MoreTranslated text
Key words
hyperspectral imaging,metabolic,embryos,oocytes,classification
AI Read Science
Must-Reading Tree
Example
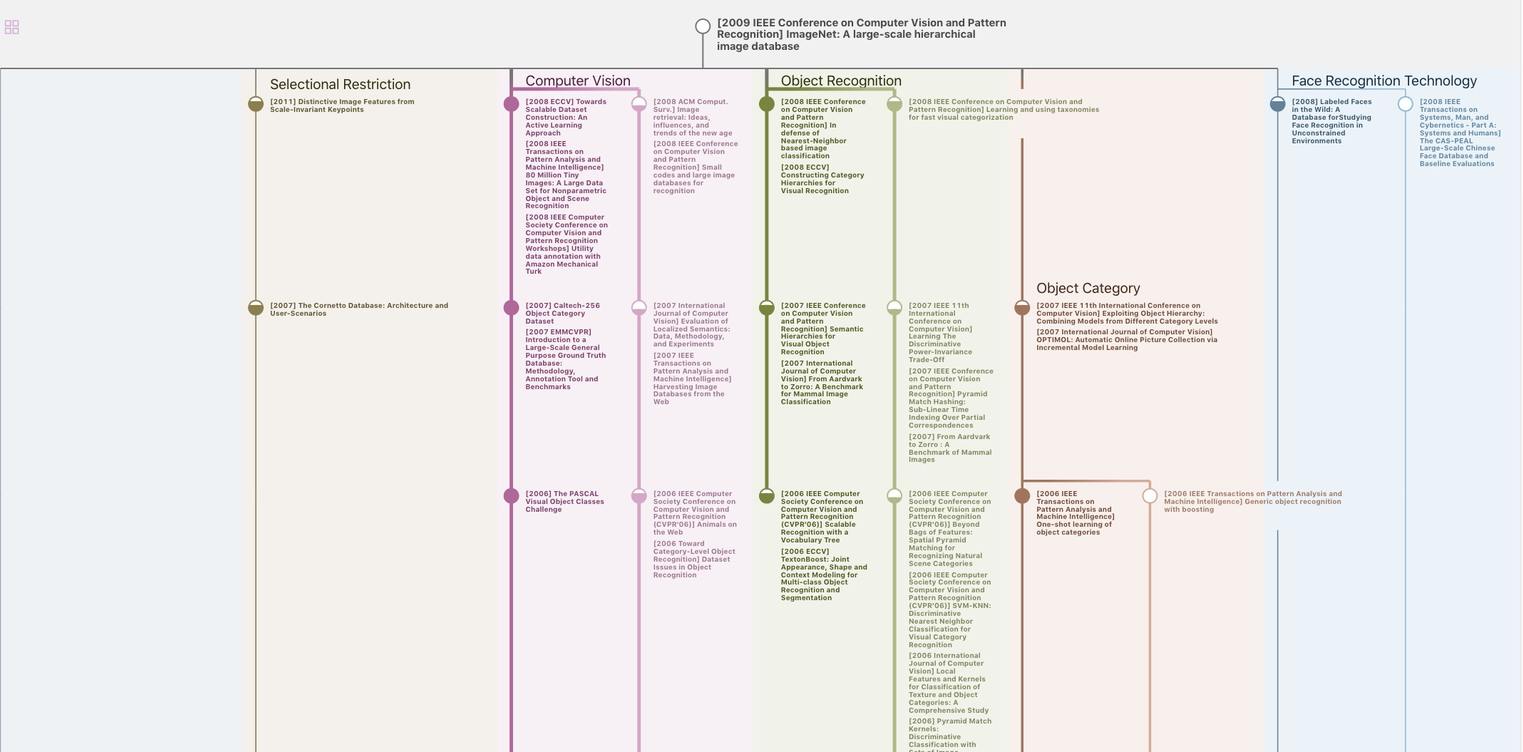
Generate MRT to find the research sequence of this paper
Chat Paper
Summary is being generated by the instructions you defined